Menu
Publications
2025
2024
2023
2022
2021
2020
2019
2018
2017
2016
2015
2014
2013
2012
2011
2010
2009
2008
2007
2006
2005
2004
2003
2002
2001
Editor-in-Chief
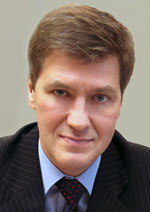
Nikiforov
Vladimir O.
D.Sc., Prof.
Partners
doi: 10.17586/2226-1494-2022-22-4-708-715
Applying the FN-corrector to improve the quality of audio event classification
Read the full article

Article in Russian
For citation:
Abstract
For citation:
Golubkov A.M., Shuranov E.V. Applying the FN-corrector to improve the quality of audio event classification. Scientific and Technical Journal of Information Technologies, Mechanics and Optics, 2022, vol. 22, no. 4, pp. 708–715 (in Russian). doi: 10.17586/2226-1494-2022-22-4-708-715
Abstract
The paper deals with the problem of acoustic events classification which is actively applied to the problems of a safe city, smart home, IoT devices, and for the detection of industrial accidence. A solution to improve the accuracy of classifiers without changing their structure and collecting additional data is proposed. The main data source for the experiments was the TUT Urban Acoustic Scenes 2018, Development Dataset. The paper presents the way to increase the accuracy of audio event classification by using the FN-corrector. The FN-corrector is a linear two-stage classifier performing the transformation of the feature space into a linearly separable space and the linear separation of one class from another. If a corrector is applied, the responses of the original classifier generate four classes: positive (P), negative (N), false positive (FP), and false negative (FN). As a result, it becomes possible to train two types of correctors: the FP-corrector separating positive and false positive classifier responses, and the FN-corrector separating negative and false negative classifier responses. In the experiments, the VGGish convolutional neural network was used as the initial classifier. The audio signal is converted into a spectrogram and is fed to the input of the neural network which forms the spectrogram feature description and performs a classification. As an example, two ”confused“ classes are selected to demonstrate the increase in classification accuracy. Using the feature description of audio recordings of these classes, an FN-corrector was built, trained and connected to the original classifier. The response from the classifier, as well as the feature description, has been passed to the corrector input. Next, the corrector translated the feature space into a new basis (into a linearly separable space) and classified the classifier answer responding to the question whether the original classifier makes a mistake on such a feature vector or not. If the original classifier made a mistake, then his answer is changed by the corrector to the opposite, otherwise the answer remains the same. The results of the experiments demonstrated a decrease in the level of class confusion and, accordingly, an increase in the accuracy of the original classifier without changing its structure and without collecting an additional data set. The results obtained can be used on IoT devices that have significant limitations on the size of the models used, as well as in solving the problems of domain adaptation which is relevant in audio analytics
Keywords: acoustic event detection, audio processing, FN-corrector, false negative corrector, DSP, CNN, convolutional neural network, audio analytics
Acknowledgements. The work was carried out as part of research supported by LETI
References
Acknowledgements. The work was carried out as part of research supported by LETI
References
-
Grollmisch S., Cano E., Kehling C., Taenzer M. Analyzing the potential of pre-trained embeddings for audio classification tasks. Proc. of the28th European Signal Processing Conference (EUSIPCO), 2021, pp. 790–794. https://doi.org/10.23919/Eusipco47968.2020.9287743
-
Matveev Y.N., Shuranov E.V., Avdeeva A.S., Shchemelinin V.L., Krylova E.V. Acoustic data based automatic object detection system. Proc. of the 2nd International Conference on Control in Technical Systems (CTS), 2017, pp. 301–303. https://doi.org/10.1109/CTSYS.2017.8109551
-
GolubkovA.M. Face recognition classification methods. Proceedings of Saint- Petersburg Electrotechnical University Journal, 2018, no. 7, pp. 26–30. (in Russian)
-
Golubkov A.M., Klionskii D.M. Cascade reduction method applied to face recognition probleb. Proceedings of Saint-Petersburg Electrotechnical UniversityJournal,2019, no. 8, pp. 47–53. (in Russian)
-
Ono N., Miyamoto K., Le Roux J., Kameoka H., Sagayama S. Separation of a monaural audio signal into harmonic/percussive components by complementary diffusion on spectrogram. Proc. of the 16th European Signal Processing Conference (EUSIPCO), 2008, pp. 1–4.
-
Sutskever I., Martens J., Dahl G., Hinton G. On the importance of initialization and momentum in deep learning. Proc. of the 30th International Conference on Machine Learning (ICML), 2013, pp. 2176–2184.
-
Gorban A., Golubkov A.M., Grechuk B., Mirkes E., Tyukin I.Y. Correction of AI systems by linear discriminants: probabilistic foundations. Information Sciences, 2018, vol. 466, pp. 303–322. https://doi.org/10.1016/j.ins.2018.07.040