Menu
Publications
2025
2024
2023
2022
2021
2020
2019
2018
2017
2016
2015
2014
2013
2012
2011
2010
2009
2008
2007
2006
2005
2004
2003
2002
2001
Editor-in-Chief
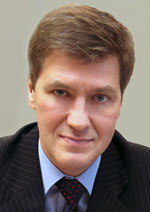
Nikiforov
Vladimir O.
D.Sc., Prof.
Partners
doi: 10.17586/2226-1494-2022-22-5-881-888
Residue feature analysis with empirical mode decomposition for mining spatial sequential patterns from serial remote sensing images.
Read the full article

Article in English
For citation:
Abstract
For citation:
Angelin Preethi R., Anandharaj G. Residue feature analysis with empirical mode decomposition for mining spatial sequential patterns from serial remote sensing images. Scientific and Technical Journal of Information Technologies, Mechanics and Optics, 2022, vol. 22, no. 5, pp. 881–888. doi: 10.17586/2226-1494-2022-22-5-881-888
Abstract
An extensive growth of serial remote sensing images paves the way for abundant data intended for the sequential spatial pattern determination in several fields like monitoring of agriculture, development of urban areas, and the vegetative area. However, conventional spatial sequential pattern mining is not applied efficiently or directly in the aspect of serial remote sensing images. Therefore, a residue feature analysis with empirical mode decomposition is proposed so as to enhance the spatial sequential pattern mining efficacy from the raster serial remote sensing images. At first, input images are being extracted by means of minima and maxima pattern by computing the mean of envelops and the intrinsic mode function components. If the intrinsic mode function condition is satisfied, then it is being subtracted from the original image; finally, the image is decomposed into many intrinsic mode functions and residue. The experimental outcomes attained indicate that the proposed strategy is proficient of mining spatial sequential pattern from the images of serial remote sensing. Though the support values of the patterns might not be attained accurately, the presented scheme guarantees that the whole patters are being extracted at lower consumption of time.
Keywords: serial remote sensing images, intrinsic mode function (IMF), image decomposition, residue feature analysis, empirical mode decomposition (EMD)
References
References
-
Kumar T.S., Nagarajan V. Local tetra pattern based on contourlet directions. Proc. of the 6th IEEE International Conference on Communication and Signal Processing (ICCSP), 2017, pp. 754–757. https://doi.org/10.1109/ICCSP.2017.8286462
-
Zhang P., Gong M., Su L., Liu J., Li Z. Change detection based on deep feature representation and mapping transformation for multi-spatial-resolution remote sensing images. ISPRS Journal of Photogrammetry and Remote Sensing, 2016, vol. 116, pp. 24–41. https://doi.org/10.1016/j.isprsjprs.2016.02.013
-
Wright A.P., Wright A.T., McCoy A.B., Sittig D.F. The use of sequential pattern mining to predict next prescribed medications. Journal of Biomedical Informatics, 2015, vol. 53, pp. 73–80. https://doi.org/10.1016/j.jbi.2014.09.003
-
Molijn R.A., Iannini L., Hanssen R.F., Vieira Rocha J. Sugarcane growth monitoring through spatial cluster and temporal trend analysis of radar and optical remote sensing images. Proc. of the 36th IEEE International Geoscience and Remote Sensing Symposium (IGARSS), 2016, pp. 7141–7144. https://doi.org/10.1109/IGARSS.2016.7730863
-
Tiwari P., Shukla P.K. A review on various features and techniques of crop yield prediction using geo-spatial data. International Journal of Organizational and Collective Intelligence (IJOCI), 2019, vol. 9, no. 1, pp. 37–50. https://doi.org/10.4018/IJOCI.2019010103
-
Wei J., Mi L., Hu Y., Ling J., Li Y., Chen Z. Effects of lossy compression on remote sensing image classification based on convolutional sparse coding. IEEE Geoscience and Remote Sensing Letters, 2022, vol. 19. https://doi.org/10.1109/LGRS.2020.3047789
-
Zhang C., Wei S., Ji S., Lu M. Detecting large-scale urban land cover changes from very high resolution remote sensing images using CNN-based classification. ISPRS International Journal of Geo-Information, 2019, vol. 8, no. 4, pp. 189. https://doi.org/10.3390/ijgi8040189
-
Xu X., Chen Y., Zhang J., Chen Y., Anandhan P., Manickam A. A novel approach for scene classification from remote sensing images using deep learning methods. European Journal of Remote Sensing, 2021, vol. 54, no. sup2, pp. 383–395. https://doi.org/10.1080/22797254.2020.1790995
-
He Z., Zhang S., Wu J. Significance-based discriminative sequential pattern mining. Expert Systems with Applications, 2019, vol. 122, pp. 54–64. https://doi.org/10.1016/j.eswa.2018.12.046
-
Cai G., Lee K., Lee I. Itinerary recommender system with semantic trajectory pattern mining from geo-tagged photos. Expert Systems with Applications, 2018, vol. 94, pp. 32–40. https://doi.org/10.1016/j.eswa.2017.10.049
-
Wu X., Zhang X.J.C. An efficient pixel clustering-based method for mining spatial sequential patterns from serial remote sensing images. Computers and Geosciences, 2019, vol. 124, pp. 128–139. https://doi.org/10.1016/j.cageo.2019.01.005
-
Preethi R.A., Anandharaj G. Quantized ternary pattern and singular value decomposition for the efficient mining of sequences in SRSI images. SN Applied Sciences, 2020, vol. 2, no. 10, pp. 1–14. https://doi.org/10.1007/s42452-020-03474-8
-
Hu W.-S., Li H-C., Pan L., Li W., Tao R., Du Q. Spatial–spectral feature extraction via deep ConvLSTM neural networks for hyperspectral image classification. IEEE Transactions on Geoscience and Remote Sensing, 2020, vol. 58, no. 6, pp. 4237–4250. https://doi.org/10.1109/TGRS.2019.2961947
-
Driss K., Boulila W., Leborgne A., Gançarski P. Mining frequent approximate patterns in large networks. International Journal of Imaging Systems and Technology, 2021, vol. 31, no. 3, pp. 1265–1279. https://doi.org/10.1002/ima.22533
-
Shen H., Lin Y., Tian Q., Xu K., Jiao J. A comparison of multiple classifier combinations using different voting-weights for remote sensing image classification. International Journal of Remote Sensing, 2018, vol. 39, no. 11, pp. 3705–3722. https://doi.org/10.1080/01431161.2018.1446566
-
Fournier-Viger P., Lin J.C.-W., Kiran R.U., Koh Y.S., Thomas R. A survey of sequential pattern mining. Data Science and Pattern Recognition, 2017, vol. 1, no. 1, pp. 54–77.
-
Song J., Zhang H., Li X., Gao L., Wang M., Hong R. Self-supervised video hashing with hierarchical binary auto-encoder. IEEE Transactions on Image Processing, 2018, vol. 27, no. 7, pp. 3210–3221. https://doi.org/10.1109/TIP.2018.2814344
-
Song J., Guo Y., Gao L., Li X., Hanjalic A., Shen H.T. From deterministic to generative: Multimodal stochastic RNNs for video captioning. IEEE Transactions on Neural Networks and Learning Systems, 2019, vol. 30, no. 10, pp. 3047–3058. https://doi.org/10.1109/TNNLS.2018.2851077
-
Menaka D., Padmasuresh L., Selvin Prem Kumar S. Classification of multispectral satellite images using sparse SVM classifier. Indian Journal of Science and Technology, 2015, vol. 8, no. 24, pp. 1–7. https://doi.org/10.17485/ijst/2015/v8i24/85355
-
Rajakumar A.P., Ganesan A. A modified extrema pattern with multilinear matrix decomposition based RLC scheme for efficient serial remote sensing images mining. Traitement du Signal, 2022, vol. 39, no. 1, pp. 153–163. https://doi.org/10.18280/ts.390115
-
Gopal B., Ganesan A. Real time deep learning framework to monitor social distancing using improved single shot detector based on overhead position. Earth Science Informatics, 2022, vol. 15, no. 1, pp. 585–602. https://doi.org/10.1007/s12145-021-00758-4
-
Kumar T.S., Nagarajan V. Local contourlet tetra pattern for image retrieval. Signal, Image and Video Processing, 2018, vol. 12, no. 3, pp. 591–598. https://doi.org/10.1007/s11760-017-1197-1