Menu
Publications
2024
2023
2022
2021
2020
2019
2018
2017
2016
2015
2014
2013
2012
2011
2010
2009
2008
2007
2006
2005
2004
2003
2002
2001
Editor-in-Chief
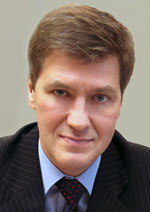
Nikiforov
Vladimir O.
D.Sc., Prof.
Partners
doi: 10.17586/2226-1494-2022-22-5-929-940
Pressure control in material extrusion additive manufacturing
Read the full article

Article in Russian
For citation:
Abstract
For citation:
Zimenko K.V., Afanasev M.Ya., Kolesnikov M.V. Pressure control in material extrusion additive manufacturing. Scientific and Technical Journal of Information Technologies, Mechanics and Optics, 2022, vol. 22, no. 5, pp. 929–940 (in Russian). doi: 10.17586/2226-1494-2022-22-5-929-940
Abstract
This paper discusses the effect called undesirable extrusion dynamics that occur during material extrusion printing or fused deposition modeling. This effect is revealed during high acceleration and deceleration of printing head and results in over-extrusion on corners of parts and printed layer unevenness. To minimize this effect, modern control systems use Advance algorithms for dynamics control. However, such disadvantages as inertia, reduced printing speed, need for manual calibration, as well as uncertainty of the influence of material, nozzle geometry, and printing process parameters on the algorithm performance do not allow this group of solutions to be applied on an industrial scale. The paper presents a study of the influence of printing characteristics, such as material type, extruder type, printing temperature, layer and nozzle geometry, on extrusion dynamics through a series of experiments. The experiments were carried out on a Creality Ender 3 printer. The obtained experimental data will allow us to deepen the understanding of extrusion dynamics influence in FDM printing; they are also used in the present study to modify existing dynamics control algorithms. The paper proposes a modification of Advance algorithm based on machine learning. It is proposed to implement an algorithm containing two trained neural network models. One model predicts changes in printing head motion to minimize residual defects and increase average print speed. The second model predicts the compensation parameter for specific printing conditions without the need for manual calibration. A neural network model was trained to determine the compensation parameter depending on the type of material, layer thickness, nozzle geometry and printing temperature. The model was trained based on experimental data. The developed algorithm was introduced into Linear Advance algorithm of the Marlin firmware and tested on Creality Ender 3. The experiments showed that the developed model can successfully predict the required compensation and can be applied during the printing process. The proposed algorithm helps to facilitate and automate the process of extrusion dynamics compensation which will expand the possibilities of FDM printers’ application in industrial conditions. The obtained algorithm can improve the accuracy and printing speed which will subsequently help to increase the economic independence and competitiveness of small design organizations and enterprises in Russia that use 3D printers. This research expands the possibilities of rapid prototyping and may help to ensure the rapid creation of pilot batches.
Keywords: 3D printing, additive manufacturing, advance, extrusion dynamics, pressure control, extruder, fused deposition modeling, fused deposition modelling, fused filament fabrication
Acknowledgements. The work was carried out under the project no. 620164 “Artificial intelligence methods for cyber-physical systems” conducted at the Faculty of Control Systems and Robotics, ITMO University.
References
Acknowledgements. The work was carried out under the project no. 620164 “Artificial intelligence methods for cyber-physical systems” conducted at the Faculty of Control Systems and Robotics, ITMO University.
References
-
Tronvoll S., Popp S., Elverum C., Welo T. Investigating pressure advance algorithms for filament-based melt extrusion additive manufacturing: theory, practice and simulations. Rapid Prototyping Journal, 2019, vol. 25, no. 5, pp. 830–839. https://doi.org/10.1108/RPJ-10-2018-0275
-
Bellini A., Güçeri S., Bertoldi M. Liquefier dynamics in fused deposition. Journal of Manufacturing Science and Engineering, 2004, vol. 126, no. 2, pp. 237–246. https://doi.org/10.1115/1.1688377
-
Oskolkov A.A., Matveev E.V., Bezukladnikov I.I., Trushnikov D.N., Krotova E.L. Advanced technologies for additive manufacturing of metal product. Bulletin PNRPU. Mechanical engineering, materials science, 2018, vol. 20, no. 3, pp. 90–105. (in Russian). https://doi.org/10.15593/2224-9877/2018.3.11
-
Ahn D., Kweon J.-H., Kwon S., Song J., Lee S. Representation of surface roughness in fused deposition modeling. Journal of Materials Processing Technology, 2009, vol. 209, no. 15-16, pp. 5593–5600. https://doi.org/10.1016/j.jmatprotec.2009.05.016
-
Pandey P.M., Reddy V.N., Dhande S.G. Improvement of surface finish by staircase machining in fused deposition modeling. Journal of Materials Processing Technology, 2003, vol. 132, no. 1-3, pp. 323–331. https://doi.org/10.1016/S0924-0136(02)00953-6
-
Aourik O., Othmani M., Saadouki B., Abouzaid Kh., Chouaf A. Fracture toughness of ABS additively manufactured by FDM process. Journal of Achievements in Materials and Manufacturing Engineering, 2021, vol. 109, no. 2, pp. 49–58. https://doi.org/10.5604/01.3001.0015.6258
-
Beniak J., Šooš L., Križan P., Matúš M., Ruprich V. Resistance and strength of conductive PLA processed by FDM additive manufacturing. Polymers, 2022, vol. 14, no. 4, pp. 678. https://doi.org/10.3390/polym14040678
-
Srinivasan R., Prathap P., Raj A., Kannan A.S., Deepak V.Influence of fused deposition modeling process parameters on the mechanical properties of PETG parts. Materials Today: Proceedings, 2020, vol. 27, no. 2, pp. 1877–1883. https://doi.org/10.1016/j.matpr.2020.03.809
-
Meng L., McWilliams B., Jarosinski W., Park H.-Y., Jung Y.-G., Lee J., Zhang J. Machine learning in additive manufacturing: A review. JOM: The journal of the Minerals, Metals & Materials Society, 2019, vol. 72, no. 6, pp. 2363–2377. https://doi.org/10.1007/s11837-020-04155-y
-
Pérez M., Carou D., Rubio E.M., Teti R. Current advances in additive manufacturing. Procedia CIRP, 2020, vol. 88, pp. 439–444. https://doi.org/10.1016/j.procir.2020.05.076
-
Razvi S.S., Feng S., Narayanan A., Lee Y.-T., Witherell P. A review of machine learning applications in additive manufacturing. Proc. of the ASME International Design Engineering Technical Conferences and Computers and Information in Engineering Conference, (IDETC-CIE), 2019, pp. 1–10. https://doi.org/10.1115/DETC2019-98415
-
Suh S.-H., Kang S.-K., Chung D.-H., Stroud I. Theory and Design of CNC Systems. Springer London, 2008, 508 p. Springer Series in Advanced Manufacturing. https://doi.org/10.1007/978-1-84800-336-1
-
Smid P. CNC Programming Handbook: A Comprehensive Guide to Practical CNC Programming. NY, Industrial Press Inc., 2003, 508 p.