Menu
Publications
2024
2023
2022
2021
2020
2019
2018
2017
2016
2015
2014
2013
2012
2011
2010
2009
2008
2007
2006
2005
2004
2003
2002
2001
Editor-in-Chief
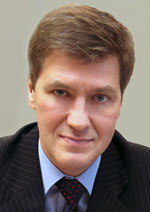
Nikiforov
Vladimir O.
D.Sc., Prof.
Partners
doi: 10.17586/2226-1494-2022-22-5-962-969
Ice reconnaissance data processing under low quality source images
Read the full article

Article in English
For citation:
Abstract
For citation:
Timofeev A.V., Groznov D.I. Ice reconnaissance data processing under low quality source images. Scientific and Technical Journal of Information Technologies, Mechanics and Optics, 2022, vol. 22, no. 5, pp. 962–969. doi: 10.17586/2226-1494-2022-22-5-962-969
Abstract
A practically effective solution to the problem of automated processing of ice reconnaissance data in high latitudes is proposed. The intermediate result of ice reconnaissance is huge aerial survey data set consisting of images of low quality; this is a consequence of the difficult conditions of aerial survey in high latitudes. The goal of the study is to create a high-level method that can either efficiently process this pre-collected data set or perform real-time processing of similar images while ensuring high reliability in solving the problem of recognizing ice class distribution on the water surface with minimal computing resources. In particular, the problem of automatic classification of ice-floe size distribution (FSD) type for a three-class model based on aerial survey data is solved. The practically important case of low-quality images is considered, a common situation for the meteorological conditions of the Far North. The proposed approach is based on the use of machine learning methods, in particular on the well-known multi-class SVM (Support Vector Machine), which is extremely undemanding to computing resources and therefore can be implemented even by the onboard computer of an ice reconnaissance UAV. From the input images of low quality some numerical characteristics of the image are calculated which informatively characterize the image. These characteristics (features) are invariant to scaling, rotation and illumination as well as have a much smaller dimensionality than the original image. The main idea underlying the proposed method is to form an original set of features which are implemented in the original feature space. These features characterize large fragments of the analyzed image and are “stable”, in contrast to the features that characterize small details. A new method of FSD type classification based on the processing of aerial survey data by using machine learning methods, which is sufficiently effective for processing low-quality images, has been proposed. Also, the original feature space for classification was proposed which ensured high practical efficiency of this method. The method has shown high efficiency when it is tested on a data set composed of low-quality real images (high blurriness, vagueness, presence of meteorological noises). The developed algorithm can be used for express analysis of ice reconnaissance data, including an ice reconnaissance UAV on-board software component.
Keywords: sea-ice floe size distribution, ice reconnaissance, image classification, multi-class SVM, image histogram, blurry images, sea-ice type classification
References
References
-
Pershin N.V. Rational creation of navigation routes taking into account hydrometeorological conditions. Proceedings of the Russian State Hydrometeorological University, 2018, vol. 52, pp. 61–66. (in Russian)
-
Mironenko A.A. The Gradient Model of the Programmed Vessels Movement. Navigation and Hydrography, 2012, vol. 34, pp. 35–42. (in Russian)
-
Kalinina N.V. Influence of the ice navigation characteristics on the ice-breaker performance. Transport Systems, 2017, vol. 3(6), pp. 37–44. (in Russian). https://doi.org/10.46960/62045_2017_3_37
-
Horvat C., Tziperman E. The evolution of scaling laws in the sea ice floe size distribution. Journal of Geophysical Research: Oceans, 2017, vol. 122, no. 9, pp. 7630–7650. https://doi.org/10.1002/2016JC012573
-
Rothrock D., Thorndike A. Measuring the sea ice floe size distribution. Journal of Geophysical Research, 1984, vol. 89, no. C4, pp. 6477–6486. https://doi.org/10.1029/JC089iC04p06477
-
Alberello A., Onorato M., Bennetts L., Vichi M., Eayrs C., MacHutchon K., Toffoli A. Brief communication: Pancake ice floe size distribution during the winter expansion of the Antarctic marginal ice zone. Cryosphere, 2019, vol. 13, no. 1, pp. 41–48. https://doi.org/10.5194/tc-13-41-2019
-
Zhang J., Schweiger A., Steele M., Stern H. Sea ice floe size distribution in the marginal ice zone: Theory and numerical experiments. Journal of Geophysical Research: Oceans, 2015, vol. 120, no. 5, pp. 3484–3498. https://doi.org/10.1002/2015jc010770
-
Lu P., Li Z.J., Zhang Z.H., Dong X.L. Aerial observations of floe size distribution in the marginal ice zone of summer Prydz Bay. Journal of Geophysical Research: Oceans, 2008, vol. 113, no. 2, pp. C02011. https://doi.org/10.1029/2006jc003965
-
Toyota T., Takatsuji S., Nakayama M. Characteristics of sea ice floe size distribution in the seasonal ice zone. Geophysical Research Letters, 2006, vol. 33, no. 2, pp. L02616. https://doi.org/10.1029/2005gl024556
-
Toyota T., Haas C., Tamura T. Size distribution and shape properties of relatively small sea-ice floes in the Antarctic marginal ice zone in late winter. Deep-Sea Research Part II: Topical Studies in Oceanography, 2011, vol. 58, no. 9-10, pp. 1182–1193. https://doi.org/10.1016/j.dsr2.2010.10.034
-
Stern H.L., Schweiger A.J., Zhang J., Steele M. On reconciling disparate studies of the sea-ice floe size distribution. Elementa, 2018, vol. 6, pp. 49. https://doi.org/doi.org/10.1525/elementa.304
-
Nautical Encyclopedic Reference Book. V. 2. Ed. by N. Isanin. Leningrad, Sudostroenie Publ., 1987, 520 с. (in Russia)
-
Leshkevich G.A. Machine classification of freshwater ice types from Landsat - I digital data using ice albedos as training sets. Remote Sensing of Environment, 1985, vol. 17, no. 3, pp. 251–263. https://doi.org/10.1016/0034-4257(85)90098-7
-
Leshkevich G.A., Nghiem S.V. Satellite SAR remote sensing of Great Lakes ice cover, part 2. Ice classification and mapping. Journal of Great Lakes Research, 2007, vol. 33, no. 4, pp. 736–750. https://doi.org/10.3394/0380-1330(2007)33[736:SSRSOG]2.0.CO;2
-
Nghiem S.V., Leshkevich G.A. Satellite SAR remote sensing of Great Lakes ice cover, part 1. Ice backscatter signatures at C band. Journal of Great Lakes Research, 2007, vol. 33, no. 4, pp. 722–735. https://doi.org/10.3394/0380-1330(2007)33[722:SSRSOG]2.0.CO;2
-
Nghiem S.V., Leshkevich G.A. Advancing a satellite synthetic aperture radar (SAR) ice classification algorithm. 54th Annual Conference on Great Lakes Research. International Association for Great Lakes Research: Abstract Book, 2011, pp. 181.
-
Schertler R.J., Mueller R.A., Jirberg R.J., Cooper D.W., Heighway J.E., Holmes A.D., Gedney R.T., Mark H. Great Lakes All-weather Ice Information System: NASA Technical Memorandum NASA TM X-71815.1975.
-
Leshkevich G., Nghiem S.V. Great Lakes ice classification using satellite C-band SAR multi-polarization data. Journal of Great Lakes Research, 2013, vol. 39, pp. 55–64. https://doi.org/10.1016/j.jglr.2013.05.003
-
Kamangir H., Rahnemoonfar M., Dobbs D., John Paden D., Fox G. Detecting ice layers in radar images with deep learning, 2018.
-
Birnbaum G., Lüpkes C. A new parameterization of surface drag in the marginal sea ice zone. Tellus, Series A: Dynamic Meteorology and Oceanography, 2002, vol. 54, no. 1, pp. 107–123. https://doi.org/10.3402/tellusa.v54i1.12121
-
Zhang Q., Skjetne R. Image processing for identification of sea-ice floes and the floe size distributions. IEEE Transactions on Geoscience and Remote Sensing, 2015, vol. 53, no. 5, pp. 2913–2924. https://doi.org/10.1109/tgrs.2014.2366640
-
Smith D., Barrett E., Scott J. Sea-ice type classification from ERS-1 SAR data based on grey level and texture information. Polar Record, 1995, vol. 31, no. 177, pp. 135–146. https://doi.org/10.1017/S0032247400013632
-
Shen X., Zhang J., Meng J., Zhang J., Ke C. Sea ice type classification based on random forest machine learning with Cryosat-2 altimeter data. Proc. of the International Workshop on Remote Sensing with Intelligent Processing (RSIP), 2017, pp. 7958792. https://doi.org/10.1109/rsip.2017.7958792
-
Aksenov Y., Bateson A.W., Feltham D.L., Schröder D., Hosekova L., Ridley J.K., Impact of sea ice floe size distribution on seasonal fragmentation and melt of Arctic sea ice. Cryosphere, 2020, vol. 14, no. 2, pp. 403–428. https://doi.org/10.5194/tc-14-403-2020
-
Johannessen O.M., Bobylev L.P., Shalina E.V., Sandven S. Sea Ice in the Arctic: Past, Present and Future. Springer Polar Sciences, 2020, X, 575 p. https://doi.org/10.1007/978-3-030-21301-5
-
Timofeev A.V. Detection of a small target object in blurry images affected by affine distortions. Scientific and Technical Journal of Information Technologies, Mechanics and Optics, 2021, vol. 21, no. 2, pp. 206–224 (in Russian). https://doi.org/10.17586/2226-1494-2021-21-2-206-224
-
Cristianini N., Shawe-Taylor J. An Introduction to Support Vector Machines and Other Kernel-based Learning Methods. Cambridge University Press, 2000. https://doi.org/10.1017/CBO9780511801389
-
He K., Zhang X., Ren S., Sun J. Deep residual learning for image recognition. Proc. of the 29th IEEE Conference on Computer Vision and Pattern Recognition (CVPR), 2016, pp. 770–778. https://doi.org/10.1109/cvpr.2016.90
-
Hastie T., Tibshirani R., Friedman J. The Elements of Statistical Learning: Data Mining, Inference and Prediction. Springer, 2009,XXII, 745 p. https://doi.org/10.1007/978-0-387-84858-7