Menu
Publications
2025
2024
2023
2022
2021
2020
2019
2018
2017
2016
2015
2014
2013
2012
2011
2010
2009
2008
2007
2006
2005
2004
2003
2002
2001
Editor-in-Chief
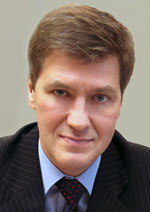
Nikiforov
Vladimir O.
D.Sc., Prof.
Partners
doi: 10.17586/2226-1494-2022-22-5-970-981
Korsakov I.N., and others
Prediction of fatal outcome in patients with confirm COVID-19
Read the full article

Article in Russian
For citation:
Abstract
For citation:
Korsakov I.N., Karonova T.L., Konradi A.O., Rubin A.D., Kurapeev D.I., Chernikova A.T., Mikhaylova A.A., Shlyakhto E.V. Prediction of fatal outcome in patients with confirm COVID-19. Scientific and Technical Journal of Information Technologies, Mechanics and Optics, 2022, vol. 22, no. 5, pp. 970–981 (in Russian). doi: 10.17586/2226-1494-2022-22-5-970-981
Abstract
SARS-CoV-2, the new coronavirus underlying the development of the COVID-19 pandemic, has led to a sharp increase in the burden on healthcare systems, high mortality and significant difficulties in organizing medical care. The aim of the study was to conduct a systematic analysis of factors affecting the course of infectious disease in patients with diagnosed COVID-19 hospitalized. In order to predict the course of the disease and determine the indications for more aggressive treatment, many different clinical and biological markers have been proposed, however, clinical and laboratory assessment of the condition is not always simple and can clearly predict the development of a severe course. Technologies based on artificial intelligence (AI) have played a significant role in predicting the development of the disease. One of the main requirements during a pandemic is an accurate prediction of the required resources and likely outcomes. In the present study, a machine learning (ML) approach is proposed to predict the fatal outcome in patients with an established diagnosis of COVID-19 based on the patient’s medical history and clinical, laboratory and instrumental data obtained in the first 72 hours of the patient’s stay in the hospital. A machine learning algorithm for predicting the lethal outcome in patients with COVID-19 during 72 hours of hospitalization demonstrated high sensitivity (0.816) and specificity (0.865). Given the serious concerns about limited resources, including ventilators, during the COVID-19 pandemic, accurately predicting patients who are likely to require artificial ventilation can help provide important recommendations regarding patient triage and resource allocation among hospitalized patients. In addition, early detection of such persons may allow for routine ventilation procedures, reducing some of the known risks associated with emergency intubation. Thus, this algorithm can help improve patient care, reduce patient mortality and minimize the burden on doctors during the COVID-19 pandemic.
Keywords: machine learning, mathematical model, classification, model metrics, ROC analysis, risk factor, COVID-19
Acknowledgements. This work was financially supported by the Ministry of Science and Higher Education of the Russian Federation (Agreement No. 075-15-2022-301).
References
Acknowledgements. This work was financially supported by the Ministry of Science and Higher Education of the Russian Federation (Agreement No. 075-15-2022-301).
References
1. Lee E.E., Hwang W., Song K.H., Jung J., Kang C.K., Kim J.H., Oh H.S., Kang Y.M., Lee E.B., Chin B.S., Song W., Kim N.J., Park J. K. Predication of oxygen requirement in COVID-19 patients using dynamic change of inflammatory markers: CRP, hypertension, age, neutrophil and lymphocyte (CHANeL). Scientific Reports, 2021, vol. 11, no. 1, pp. 13026. https://doi.org/10.1038/s41598-021-92418-2
2. Yu L., Halalau A., Dalal B., Abbas A.E., Ivascu F., Amin M., Nair G.B. Machine learning methods to predict mechanical ventilation and mortality in patients with COVID-19. PLoS One, 2021, vol. 16, no. 4, pp. e0249285. https://doi.org/10.1371/journal.pone.0249285
3. Burdick H., Lam C., Mataraso S., Siefkas A., Braden G., Dellinger R.P., McCoy A., Vincent J.L., Green-Saxena A., Barnes G., Hoffman J., Calvert J., Pellegrini E., Das R. Prediction of respiratory decompensation in Covid-19 patients using machine learning: The READY trial. Computers in Biology and Medicine, 2020, vol. 124, pp. 103949. https://doi.org/10.1016/j.compbiomed.2020.103949
4. Syeda H.B., Syed M., Sexton K.W., Syed S., Begum S., Syed F., Prior F., Yu F. Role of machine learning techniques to tackle the COVID-19 crisis: systematic review. JMIR Medical Informatics, 2021, vol. 9, no. 1, pp. e23811. https://doi.org/10.2196/23811
5. Jehi L., Ji X., Milinovich A., Erzurum S., Merlino A., Gordon S., Young J.B., Kattan M.W. Development and validation of a model for individualized prediction of hospitalization risk in 4,536 patients with COVID-19. PloS ONE, 2020, vol. 15, no. 8, pp. e0237419. https://doi.org/10.1371/journal.pone.0237419
6. Moez Ali. PyCaret: An open source, low-code machine learning library in Python. PyCaret version 2.1. 2020. Available at: https://www.pycaret.org (accessed: 21.07.2022).
7. Lundberg S.M., Erion G., Chen H., DeGrave A., Prutkin J.M., Nair B., Katz R., Himmelfarb J., Bansal N., Lee S.-I. From local explanations to global understanding with explainable AI for trees. Nature Machine Intelligence, 2020, vol. 2, no. 1, pp. 56–67. https://doi.org/10.1038/s42256-019-0138-9
8. Chen H., Lundberg S.M., Lee S.I. Explaining models by propagating shapley values of local components. Studies in Computational Intelligence, 2021, vol. 914, pp. 261–270. https://doi.org/10.1007/978-3-030-53352-6_24
9. Linardatos P., Papastefanopoulos V., Kotsiantis S. Explainable ai: A review of machine learning interpretability methods. Entropy, 2021, vol. 23, no. 1, pp. 18. https://doi.org/10.3390/e23010018
10. Li X., Xiong H., Li X., Wu X., Zhang X., Liu J., Bian J., Dou D. Interpretable deep learning: interpretation, interpretability, trustworthiness, and beyond. Knowledge and Information Systems, 2022, in press. https://doi.org/10.1007/s10115-022-01756-8
11. Yang Y., Zhu X.F., Huang J., Chen C., Zheng Y., He W., Zhao L.H., Gao Q., Huang X.X., Fu L.J., Zhang Y., Chang Y.Q., Zhang H.J., Lu Z.J. Nomogram for prediction of fatal outcome in patients with severe COVID-19: a multicenter study. Military Medical Research, 2021, vol. 8, no. 1, pp. 21. https://doi.org/10.1186/s40779-021-00315-6
12. Zarei J., Jamshidnezhad A., Haddadzadeh Shoushtari M., Mohammad Hadianfard A., Cheraghi M., Sheikhtaheri A. Machine learning models to predict in-hospital mortality among inpatients with COVID-19: Underestimation and overestimation bias analysis in subgroup populations. Journal of Healthcare Engineering, 2022, pp. 1644910. https://doi.org/10.1155/2022/1644910
13. Baker K.F., Hanrath A.T., van der Loeff I.S., Kay L.J., Back J., Duncan C.J. National early warning score 2 (NEWS2) to identify inpatient COVID-19 deterioration: a retrospective analysis. Clinical Medicine, Journal of the Royal College of Physicians of London, 2021, vol. 21, no. 2, pp. 84–89. https://doi.org/10.7861/clinmed.2020-0688
14. Kondapalli A.R., Koganti H., Challagundla S.K., Guntaka C., Biswas S. Machine learning predictions of COVID-19 second wave end-times in Indian states. Indian Journal of Physics, 2022, vol. 96, no. 8, pp. 2547–2555. https://doi.org/10.1007/s12648-021-02195-x
15. Mahdavi M., Choubdar H., Zabeh E., Rieder M., Safavi-Naeini S., Jobbagy Z., Ghorbani A., Abedini A., Kiani A., Khanlarzadeh V., Lashgari R., Kamrani E. A machine learning based exploration of COVID-19 mortality risk. Plos ONE. 2021, vol. 16, no. 7, pp. e0252384. https://doi.org/10.1371/journal.pone.0252384