Menu
Publications
2025
2024
2023
2022
2021
2020
2019
2018
2017
2016
2015
2014
2013
2012
2011
2010
2009
2008
2007
2006
2005
2004
2003
2002
2001
Editor-in-Chief
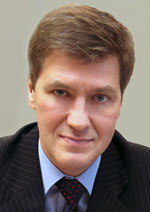
Nikiforov
Vladimir O.
D.Sc., Prof.
Partners
doi: 10.17586/2226-1494-2022-22-6-1055-1062
Distribution optimization method of pixel density by surveillance area
Read the full article

Article in Russian
For citation:
Abstract
For citation:
Volkhonskiy V.V., Kovalevskiy V.A. Distribution optimization method of pixel density by surveillance area. Scientific and Technical Journal of Information Technologies, Mechanics and Optics, 2022, vol. 22, no. 6, pp. 1055–1062
(in Russian). doi: 10.17586/2226-1494-2022-22-6-1055-1062
Abstract
The task of optimizing the parameters of the video camera matrix to reduce the redundancy of the generated video signal is considered. The relevance of the topic is due to the existing redundancy of video signals generated by matrices in different parts of the observation zone and, as a result, excessive loading of signal transmission, storage and processing devices. The problem is solved by achieving a uniform distribution of pixel density over the observation area. The methodology is based on solving the problem programmatically, unlike existing solutions using hardware methods. It is based on the representation of the view area as a set of subsets of the observation task space with its subsequent fragmentation in accordance with the observation tasks being solved which determine the required minimum pixel density in different fragments. To solve the problem, the matrix is segmented in according to the size of the fragments of the observation area and the specified allowable range of changes in the distribution of pixel density in the viewing area of the camera. Mandatory and additional distribution optimization criteria are proposed. The optimization technique is formulated. In according to the redundancy coefficient of the pixel density distribution in different segments, pixels are combined into groups that are different in different segments. Examples of solving optimization problems according to different criteria are given. The proposed approach makes it possible to minimize the pixel density redundancy and thereby reduce the load on communication channels, the amount of memory in video information storage devices, and the performance requirements for video signal processing devices. In this case, the problem of forming a continuous image of the observation zone is also solved. Results could be used for video signal processing and design of new cameras for video surveillance systems.
Keywords: pixel density distribution, pixel density, observation task, camera matrix, view area
References
References
-
Volkhonsky V.V. Video Surveillance Systems: Design and Application Fundamentals. Moscow, Gorjachaja linija-Telekom Publ., 2020, 392 p. (in Russian)
-
Volkhonskii V.V., Kovalevskii V.A. Approach to the choice of TV-camera matrix resolution for optimizing video signal parameters. Micro- and nanotechnologies in electronics: Proceedings of the XII International scientific and technical conference, Nalchik, KBSU, 2021, pp. 412–416. (in Russian)
-
Volkhonsky V., Kovalevsky V. Influence of camera installation parameters on the information content of the video image. Security and Safety, 2022, no. 2(164), pp. 78–80. (in Russian)
-
Alpert S., Galun M., Brandt A., Basri R. Image segmentation by probabilistic bottom-up aggregation and cue integration. IEEE Transactions on Pattern Analysis and Machine Intelligence, 2012, vol. 34, no. 2, pp. 315–327. https://doi.org/10.1109/TPAMI.2011.130
-
Chen C.H., Patel V.M., Chellappa R. Matrix completion for resolving label ambiguity. Proc. of the IEEE Conference on Computer Vision and Pattern Recognition (CVPR), 2015, pp. 4110–4118. https://doi.org/10.1109/CVPR.2015.7299038
-
Wronski B., Garcia-Dorado I., Ernst M., Kelly D., Krainin M. Handheld multi-frame super-resolution. ACM Transactions on Graphics (TOG), 2019, vol. 38, no. 4, pp. 1–18. https://doi.org/10.1145/3306346.3323024
-
Panda R., Roy-Chowdhury A.K. Multi-view surveillance video summarization via joint embedding and sparse optimization. IEEE Transactions on Multimedia, 2017, vol. 19, no. 9, pp. 2010–2021. https://doi.org/10.1109/TMM.2017.2708981
-
Luo Z., Huang Y., Li S., Wang L., Tan T. Unfolding the alternating optimization for blind super resolution. Advances in Neural Information Processing Systems, 2020, vol. 33, pp. 5632–5643.
-
Su H., Yu L., Jung C. Joint contrast enhancement and noise reduction of low light images via JND transform. IEEE Transactions on Multimedia, 2020, vol. 24, pp. 17–32. https://doi.org/10.1109/TMM.2020.3043106
-
Ma L., Liu R., Wang Y., Fan X., Luo Z. Low-light image enhancement via self-reinforced retinex projection model. IEEE Transactions on Multimedia, 2022, in press. https://doi.org/10.1109/TMM.2022.3162493
-
Liang C.H., Chen Y.-A., Liu Y.-C., Hsu W.H. Raw image deblurring. IEEE Transactions on Multimedia, 2020, vol. 24, pp. 61–72. https://doi.org/10.1109/TMM.2020.3045303
-
Volkhonskii V.V., Muratov A.S. Specific features of multimodule TV-cameras application. Tehnologii zashhity, 2021, no. 2, pp. 52–54. (in Russian)
-
Dallmeier D. Monitoring Device. Patent WO 2012/164089 A1.
-
Dallmeier D. Monitoring Device. Patent EAPO 026475, 2017. (in Russian)
-
Stening J., Persson H., Rusz A., Bengtsson A. System for panoramic imaging. Patent US 2020/0195845 A1.