Menu
Publications
2024
2023
2022
2021
2020
2019
2018
2017
2016
2015
2014
2013
2012
2011
2010
2009
2008
2007
2006
2005
2004
2003
2002
2001
Editor-in-Chief
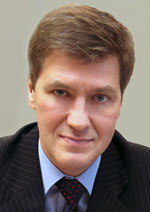
Nikiforov
Vladimir O.
D.Sc., Prof.
Partners
doi: 10.17586/2226-1494-2022-22-6-1159-1165
Application of the text wave model to the sentiment analysis problem
Read the full article

Article in Russian
For citation:
Abstract
For citation:
Gruzdeva A.S., Iurev R.N., Bessmertny I.A. Application of the text wave model to the sentiment analysis problem. Scientific and Technical Journal of Information Technologies, Mechanics and Optics, 2022, vol. 22, no. 6, pp. 1159–1165 (in Russian). doi: 10.17586/2226-1494-2022-22-6-1159-1165
Abstract
Authors researched the wave model of text representation which is one of the implementations of distributive semantics. This model takes into account not only the frequency of words occurrence in the text, but also their mutual location. The purpose of the study: to increase the accuracy of the analysis of the tonality of short texts based on the wave model. The method of determining the relationship between the text and the term is based on the calculation of the probability amplitude of the text and term proximity using a wave model. The term with the highest probability amplitude is considered to correspond most closely to the meaning of the text. The wave model allowed taking into account the fact that well-known methods define antonyms as semantically close lexical units. For the experimental study of this technique, a solution to the problem of sentiment analysis was chosen, exactly, finding the correspondence of user reviews about the product to the classes “positive” and “negative”. As a result, the accuracy of the text tonality defining was obtained up to 76.4 %, which exceeds the accuracy of the classical approach as well as the well-known methods of sentiment analysis for the Russian language. In addition, authors detected significant influence on classification accuracy of such model parameters as the choice of a basic distributive semantic model, the choice of a control point for calculating wave numbers, taking into account the influence of antonyms. The presented model has shown high accuracy in identifying the relationships of the text with concepts that are not explicitly present in it and can be successfully used as a mathematical basis for solving problems of sentiment analysis. In addition, the results obtained indicate the potential use of the wave model in other areas that require the classification of texts by indirect signs, for example, to determine the elements of author psychological portrait.
Keywords: sentiment analysis, classification, natural language processing, wave model, quantum-like model
Acknowledgements. The work was carried out within the framework of the project no. 620164 (Artificial intelligence methods for cyberphysical systems).
References
Acknowledgements. The work was carried out within the framework of the project no. 620164 (Artificial intelligence methods for cyberphysical systems).
References
-
Garg N., Sharma K. Text pre-processing of multilingual for sentiment analysis based on social network data. International Journal of Electrical and Computer Engineering, 2022, vol. 12, no. 1, pp. 776–784. https://doi.org/10.11591/ijece.v12i1.pp776-784
-
Korney A.O., Kryuchkova E.N. Performance aspects of the russian texts sentiment analysis in social networks. High-perfomance computing systems and technologies, 2018, vol. 2, no. 2, pp. 87–92. (in Russian)
-
Pazelskaia A.G., Solovev A.N. Method for determining emotions in Russian texts. Proc. of the International conference on computational linguistics and intellectual technologies “Dialogue 2011”. Moscow, RSUH, 2011, pp. 510–522. (in Russian)
-
KlekovkinaM., KotelnikovE. The automatics entimentt extclassificat ion method based on emotional vocabulary. Digital Libraries: Advanced Methods and Technologies. Proceedings of the RCDL 2012, 2012, pp. 118–123. (inRussian)
-
BessmertnyiI.A., DzhaliashviliZ.O., MaksimovV.V., MarkinD.A. Linguistic evaluation of the text. Abstracts of the X International Conference "Application of New Technologies in Education", Troitsk, BYTIC, 1999. (in Russian)
-
Harris Z. Mathematical Structures of Language. Wiley, 1968, 230 p.
-
BogdanovA.L., DulyaI.S. Sentimentanalysis of shor tRussian texts inso cial media. Tomsk State University Journal of Economics, 2019, no. 47, pp. 220–241. (in Russian). https://doi.org/10.17223/19988648/47/17
-
Riabykin A.S., Sukhov E.A.Neural network methods in the Sentiment Analysis problem. DSPA, 2022, vol. 2, no. 2, pp. 41–57. (in Russian)
-
VorobyovA.A., RybakA.M., SeredkinR.A., AndrosovA.Yu, SolovyovB.I. Method sof collecting and processing sociological information from theI nternet. Izvestiya Tula State University. Technical Sciences, 2022, no. 2, pp. 208–213. (in Russian). https://doi.org/10.24412/2071-6168-2022-2-208-214
-
Fomina I.K., Tataurova A.S. Increasing the efficiency of the classification model for determining the text key. Aktual’nye problemy ekonomiki i upravleniya, 2022, no. 1, pp. 55–58. (in Russian)
-
Zuccon G., Azzopardi L., van Rijsbergen K. The quantum probability ranking principle for information retrieval. Lecture Notes in Computer Science (including subseries Lecture Notes in Artificial Intelligence and Lecture Notes in Bioinformatics),2009,vol. 5766, pp. 232–240.https://doi.org/10.1007/978-3-642-04417-5_21
-
Melucci M. Introduction to Information Retrieval and Quantum Mechanics. Springer-Verlag Berlin Heidelberg, 2015, 247 р. https://doi.org/10.1007/978-3-662-48313-8
-
KhrennikovA. Introduction to QuantumI nformationTheory. Moscow, FizmatlitPubl., 2008, 283 p. (in Russian)
-
Blacoe W., Kashefi E., Lapata M. A quantum-theoretic approach to distributional semantics. Proc. of the 2013 Conference of the North American Chapter of the Association for Computational Linguistics: Human Language Technologies(NAACL HLT), 2013, pp. 847–857.
-
Jaiswal A.K., Holdack G., Frommholz I., Liu H. Quantum-like Generalization of Complex Word Embedding: a lightweight approach for textual classification. CEUR Workshop Proceedings,2018, vol. 2191, pp. 159–168.
-
SurovI.A., Semenenko E., Platonov A.V., Bessmertny I.A., Galofaro F., Toffano Z., Khrennikov A.Yu., Alodjants A.P. Quantum semantics oft extperception. Scientific Reports, 2021,vol. 11, no. 1, pp. 4193. https://doi.org/10.1038/s41598-021-83490-9
-
GruzdevaA.S., BessmertnyI.A Classification of short texts using awave model. Scientific and Technical Journal of Information Technologies, Mechanics and Optics, 2022, vol. 22, no. 2, pp. 287–293. (inRussian). https://doi.org/10.17586/2226-1494-2022-22-2-287-293
-
Frenkel Ia.I.Wave Mechanics. Part I. Elementary Theory. Quantum physics. Moscow, 2019, 386 p. (in Russian)
-
KutuzovA., KuzmenkoE. Webvectors: At oolkitf orbuilding web interfac esforve ctorse mantic models. Communications in Computer and Information S cience,2017,vol. 661,pp. 155–161. https://doi.org/10.1007/978-3-319-52920-2_15
-
Panchenko A., Ustalov D., Arefyev N., Paperno D., Konstantinova N., Loukachevitch N.,Biemann C. Human and machine judgements about russian semantic relatedness.Communications in Computer and Information Science, 2016, vol. 661, pp. 221–235. https://doi.org/10.1007/978-3-319-52920-2_21
-
Pang B., Lee L. A sentimental education: Sentiment analysis using subjectivity summarization based on minimum cuts. Proc. of the 42nd Annual Meeting Association for Computational Linguistics (ACL),2004, pp. 271–278.https://doi.org/10.3115/1218955.1218990
-
Menshikov I. Sentiment analysis of the text in Russian using graph models. Reports of the All-Russian Scientific Conference "Analysis of Images, Networks and Texts, AINT (AIST)", 2013, pp. 151-155. (in Russian)
-
González F.A., Caicedo J.C. Quantum latent semantic analysis.Lecture Notes in Computer Science (including subseries Lecture Notes in Artificial Intelligence and Lecture Notes in Bioinformatics),2011, vol. 6931, pp. 52–63.https://doi.org/10.1007/978-3-642-23318-0_7
-
Dalla Chiara M.L., Giuntini R., Luciani A.R., Negri E.A quantum-like semantic analysis of ambiguity in music.Soft Computing,2017, vol. 21,no. 6, pp. 1473–1481.https://doi.org/10.1007/s00500-015-1895-y