Menu
Publications
2025
2024
2023
2022
2021
2020
2019
2018
2017
2016
2015
2014
2013
2012
2011
2010
2009
2008
2007
2006
2005
2004
2003
2002
2001
Editor-in-Chief
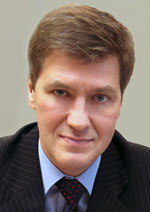
Nikiforov
Vladimir O.
D.Sc., Prof.
Partners
doi: 10.17586/2226-1494-2022-22-6-1205-1215
The effect of signal-to-noise ratio value on the error in measuring acoustic emission parameters: statistical assessment
Read the full article

Article in Russian
For citation:
Abstract
For citation:
Fedorov A.V., Altay Y., Stepanova K.A., Kuzivanov D.O. The effect of signal-to-noise ratio value on the error in measuring acoustic emission parameters: statistical assessment. Scientific and Technical Journal of Information Technologies,Mechanics and Optics, 2022, vol. 22, no. 6, pp. 1205–1215 (in Russian). doi: 10.17586/2226-1494-2022-22-6-1205-1215
Abstract
Modern acoustic emission diagnostic systems and complexes are a sensitive tool for detecting developing defects at an early stage when monitoring the technical condition of objects under operational loads. A significant limitation of the application acoustic emission method is the difficulty in isolating signals against the background of acoustic and electromagnetic interference. The effect of interference during acoustic emission recording significantly complicates the interpretation of parameters that characterize the technical condition of the test object. To increase the value signal-to-noise ratio and increase the reliability of the results of acoustic emission testing in the quantitative assessment of parameters, filtering methods are used. The subject of this study is the study of the effect of signal-to-noise ratio value on the measurement error acoustic emission parameters formatted during noise compensation using the polynomial filtering method. The basis of the statistical model characterizing the effect of signal-to-noise ratio value on the measurement error acoustic emission parameters is based on the machine learning method — linear regression. The dependence of the measurement error on the signal-to-noise ratio value was approximated by the least-squares method and visualized using a scattergram. It was found that when using the Butterworth filter, the relative measurement error acoustic emission parameters do not exceed 3 %, which are orders of magnitude lower than the values obtained for the Bessel filter and Daubechies mother functions 8 based on wavelet filter. A high inverse non-random correlation was established (r > 0.9), due to a decrease in the values of the relative measurement error emission parameters and an increase in the signal-to-noise ratio value. The developed statistical model describes the effect of the signal-to-noise ratio value on the value relative error in estimating the acoustic emission parameters. The adequacy of the developed model was confirmed by calculating the coefficient of determination and checking its statistical significance. It is shown that the use of Butterworth filter to compensate for interference significantly increases the information content of the results of measurements of acoustic emission parameters. The developed statistical model can be used in the development of new or improvement of existing complexes and systems for processing acoustic emission data to improve the reliability of the results of acoustic testing.
Keywords: statistical processing, measurement accuracy improvement, acoustic emission, signal-to-noise ratio, non-destructive
testing, noise, Butterworth filter, wavelet filter, Bessel filter
References
References
-
He Y., Li M., Meng Z., Chen S., Huang S., Hu Y., Zou X. An overview of acoustic emission inspection and monitoring technology in the key components of renewable energy systems. Mechanical Systems and Signal Processing, 2021, vol. 148, pp. 107146. https://doi.org/10.1016/j.ymssp.2020.107146
-
Kharrat M.А., Ramasso E., Placet V., Boubakar M.L. A signal processing approach for enhanced acoustic emission data analysis in high activity systems: Application to organic matrix composites. Mechanical Systems and Signal Processing, 2016, vol. 70-71, pp. 1038–1055. https://doi.org/10.1016/j.ymssp.2015.08.028
-
Il K.K., Hwan R.U., Pil C.B. An appropriate thresholding method of wavelet denoising for dropping ambient noise. International Journal of Wavelets, Multiresolution and Information Processing, 2018, vol. 16, no. 3, pp. 1850012. https://doi.org/10.1142/S0219691318500121
-
Barat V., Borodin Y., Kuzmin A. Intelligent AE signal filtering methods. Journal of Acoustic Emission, 2010, vol. 28, pp. 109–119.
-
Altay Y.A., Fedorov A.V., Stepanova K.A. Acoustic emission signal processing based on polynomial filtering method. Proc. of the 2022 IEEE Conference of Russian Young Researchers in Electrical and Electronic Engineering (ElConRus), 2022, pp. 1320–1326. https://doi.org/10.1109/ElConRus54750.2022.9755729
-
Barat V.A. Development of the acoustic emission method by automating data processing, increasing noise immunity and detection fidelity of the crack-like defects in metal structures. Dissertation abstract for the degree of doctor of technical sciences. Moscow, 2019, 40 p. (in Russian)
-
AltayY.E., FedorovA.V., StepanovaK.A. Estimation of relation ship between information component sand n oiseof acousticemissi on signals. Diagnostics, 2022, vol. 25, no. 6, pp. 38–47. (in Russian). https://doi.org/10.14489/td.2022.06.pp.038-047
-
Altay Y.,Fedorov A.V.,Stepanova K.A. Assessment the effect of filtering methods on the measurement error of acoustic emission signal parameters. International Conference on Soft Computing and Measurements, 2022, vol. 1, pp. 24–27.(in Russian)
-
PaarmanL.D. Designan dA nalysiso fAnalog Filters: A Signal Processing Perspective. NY, KluwerAcademic Publishers, 2001, 440 р.
-
SomefunO., AkingbadeK., DahunsiF. Uniformly dampedb inomi al filters: five-percent maxi mumover shoot optimalr esponse design. Circuits, Systems, and Signal Processing, 2022, vol. 41, no. 6, pp. 3282–3305. https://doi.org/10.1007/s00034-021-01931-2
-
BystrovS.V., VunderN.A., UshakovA.V. Solution ofs ignalu ncerta intyp roblematanalyti calde signofcon secutivecom pensato rin piezo actuatorcontro l. Scientific and Technical Journal of Information Technologies, Mechanics and Optics, 2016, no. 3,pp. 451–459. (in Russian). https://doi.org/10.17586/2226-1494-2016-16-3-451-459
-
Bystrov S.V., Vunder N.A., Sinetova M.M., Ushakov A.V. Analytical design of consecutive compensator for control systems with delay based on modification of typical polynomial models. SPIIRAS Proceedings, 2017, no. 3(52), pp. 115–136. (in Russian). https://doi.org/10.15622/sp.52.6
-
SeryeznovA.N., StepanovaL.N., KabanovS.I., ChernovaV.V. Diagnos ticmoduleof acousticemissionsystemwith autom aticnoise fil tering. Sensors & Systems, 2020, no. 5, pp. 3–14. (inRussian). https://doi.org/10.25728/datsys.2020.5.1
-
Altay Y., Fedorov A.V., Stepanova K.A., Kuzivanov D.O. Estimating efficiency of acoustic emission signal processing methods in implementation of polynomial digital filters. Omsk Scientific Bulletin, 2022, no. 3, pp. 128–134. (in Russian). https://doi.org/10.25206/1813-8225-2022-183-128-134
-
Kharrat M., Ramasso E, Placet V., Baubakar M.L. A signal processing approach for enhanced Acoustic Emission data analysis in high activity systems: Application to organic matrix composites. Mechanical Systems and Signal Processing, 2016, vol. 70-71, pp. 1038–1055.
-
Levin B.R. Theoretical Background Of Statistical Radio Engineering. Moscow, Sovetskoe radio Publ., 1968, 504 p. (in Russian)
-
ZakharovL.А., MartyushevD.А., PonomarevaI.N. Predictingdyna micformatio npressu reusingartificia lintelligenceme thods. JournalofMiningInstitute, 2022,vol. 253, no. 1, pp. 23–32. https://doi.org/10.31897/PMI.2022.11
-
Bekher S.A., Bobrov A.L. Fundamentals of Nondestructive Testing by the Method of Acoustic Emission. Novosibirsk, STU, 2013, 145 p. (in Russian)
-
Salin V.N., Churilova E.Iu. Practical Course on "Statistics". Moscow, Perspektiva Publ., 2002, 188 p. (in Russian)
-
ElforjaniM., ShanbrS. Prognosis ofbearingacoustice missionsignals usings upervise dmachin elearni ng. IEEETransactio nsonIn dustria lElectronics, 2018, vol. 65,no. 7, pp. 5864–5871. https://doi.org/10.1109/TIE.2017.2767551
-
Ovcharuk V.N., Turisev Iu.A. Registration and Pro cessing of Aco ustic-E mission Information in Multichannel Systems. Khabarovsk, PNU, 2017, 116 p. (in Russian)
-
Rakshit M., Das S. An efficient ECG denoising methodology using empirical mode decomposition and adaptive switching mean filter. Biomedical Signal Processing and Control, 2018, vol. 40, pp. 140–148. https://doi.org/10.1016/j.bspc.2017.09.020
-
Altay Y.A., Kremlev A.S. Signal-to-noise ratio and mean square error improving algorithms based on newton filters for measurement ECG data processing.Proc. of the 2021 IEEE Conference of Russian Young Researchers in Electrical and Electronic Engineering (ElConRus),2021, pp. 1590–1595. https://doi.org/10.1109/ElConRus51938.2021.9396391
-
Altay Y.A., Kremlev A.S., Zimenko K.A., Margun A.A. The effect of filter parameters on the accuracy of ECG signal measurement. Biomedical Engineering, 2019, vol. 53, no. 3, pp. 176–180. https://doi.org/10.1007/s10527-019-09903-2
-
Avdeeva D.K., KazakovV.Y., Natalinova N.M., Ivanov M.L., Yuzhakova M.A., Turushev N.V. The simulation results of the high-pass and low-pass filter effect on the quality of micropotential recordings on the electrocardiogram. European Journal of Physical and Health Education, 2014, vol. 6, pp. 1–10.
-
Malghan P.G., Hota M.K. A review on ECG filtering techniques for rhythm analysis. Research on Biomedical Engineering, 2020, vol. 36, no. 2, pp. 171–186. https://doi.org/10.1007/s42600-020-00057-9