Menu
Publications
2025
2024
2023
2022
2021
2020
2019
2018
2017
2016
2015
2014
2013
2012
2011
2010
2009
2008
2007
2006
2005
2004
2003
2002
2001
Editor-in-Chief
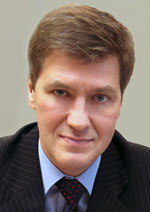
Nikiforov
Vladimir O.
D.Sc., Prof.
Partners
doi: 10.17586/2226-1494-2023-23-2-352-363
A survey of network intrusion detection systems based deep learning approaches
Read the full article

Article in English
For citation:
Abstract
For citation:
Al-Safaar D.W., Al-Yaseen W.L. A survey of network intrusion detection systems based on deep learning approaches. Scientific and Technical Journal of Information Technologies, Mechanics and Optics, 2023, vol. 23, no. 2, pp. 352–363. doi: 10.17586/2226-1494-2023-23-2-352-363
Abstract
Currently, most IT organizations are inclined towards a cloud computing environment because of its distributed and scalable nature. However, its flexible and open architecture is receiving lots of attention from potential intruders for cyber threats. Here, Intrusion Detection System (IDS) plays a significant role in monitoring malicious activities in cloud-based systems. The state of the art of this paper is to systematically review the existing methods for detecting intrusions based upon various techniques, such as data mining, machine learning, and deep learning methods. Recently, deep learning techniques have gained momentum in the intrusion detection domain, and several IDS approaches are provided in the literature using various deep learning techniques to deal with privacy concerns and security threats. For this purpose, the article focuses on the deep IDS approaches and investigates how deep learning networks are employed by different approaches in various steps of the intrusion detection process to achieve better results. Then, it provided a comparison of the deep learning approaches and the shallow machine learning methods. Also, it describes datasets that are most used in IDS.
Keywords: cloud computing, intrusion detection system, machine learning, deep learning
References
References
-
Deshpande P., Sharma S.C., Peddoju S.K., Junaid S. HIDS: A host based intrusion detection system for cloud computing environment. International Journal of System Assurance Engineering and Management, 2018, vol. 9, no. 3, pp. 567–576. https://doi.org/10.1007/s13198-014-0277-7
-
Shamshirband S., Fathi M., Chronopoulos A.T., Montieri A., Palumbo F., Pescapè A. Computational intelligence intrusion detection techniques in mobile cloud computing environments: Review, taxonomy, and open research issues. Journal of Information Security and Applications, 2020, vol. 55, pp. 102582. https://doi.org/10.1016/j.jisa.2020.102582
-
Aldweesha A., Derhabb A., Emamc A.Z. Deep learning approaches for anomaly-based intrusion detection systems: A Survey, taxonomy, and open issues. Knowledge-Based Systems, 2020, vol. 189, pp. 105124. https://doi.org/10.1016/j.knosys.2019.105124
-
AbdAllah E.G., Zulkernine M., Hassanein H.S. Preventing unauthorized access in information centric networking. Security and Privacy, 2018, vol. 1, no. 4, pp. e33. https://doi.org/10.1002/spy2.33
-
Ahmad Z., Khan A.S., Shiang C.W., Abdullah J., Ahmad F.Network intrusion detection system: A systematic study of machine learning and deep learning approaches. Transactions on Emerging Telecommunications Technologies, 2021, vol. 32, no. 1, pp. e4150. https://doi.org/10.1002/ett.4150
-
Tun H., Lupin S., Linn H.H., Lin K.N.Z. Selection the perimeter protection equipment in security systems. Proc. of the IEEE Conference of Russian Young Researchers in Electrical and Electronic Engineering (EIConRus), 2018, pp. 1504–1508. https://doi.org/10.1109/eiconrus.2018.831738
-
Saxena A., Mueller C. Intelligent intrusion detection in computer networks using swarm intelligence. International Journal of Computer Applications, 2018, vol. 179, no. 16, pp. 1–9. https://doi.org/10.5120/ijca2018916224
-
Liu G., Zhang J. CNID: Research of network intrusion detection based on convolutional neural network. Discrete Dynamics in Nature and Society, 2020, vol. 2020, pp. 1–11. https://doi.org/10.1155/2020/4705982
-
Wu Y., Wei D., Feng J. Network attacks detection methods based on deep learning techniques: A survey. Security and Communication Networks, 2020, vol. 2020, pp. 1–17. https://doi.org/10.1155/2020/8872923
-
Vincent P., Larochelle H., Bengio Y., Manzagol P.-A. Extracting and composing robust features with Denoising autoencoders. Proceedings of the 25th International Conference on Machine learning (ICML), pp. 1096–1103. https://doi.org/10.1145/1390156.1390294
-
Vincent P., Larochelle H., Lajoie I., Bengio Y., Manzagol P.A. Stacked denoising autoencoders: Learning useful representations in a deep network with a local denoising criterion. Journal of Machine Learning Research, 2010, vol. 11, pp. 3371–3408.
-
Deng J., Zhang Z., Marchi E., Schuller B. Sparse autoencoder-based feature transfer learning for speech emotion recognition. Proc. of the 2013 Humaine Association Conference on Affective Computing and Intelligent Interaction, 2013, pp. 511–516. https://doi.org/10.1109/acii.2013.90
-
Yan B., Han G. Effective feature extraction via stacked sparse autoencoder to improve intrusion detection system. IEEE Access, 2018, vol. 6, pp. 41238–41248. https://doi.org/10.1109/access.2018.2858277
-
Farahnakian F., Heikkonen J. A deep auto-encoder based approach for intrusion detection system. Proc. of the 20th International Conference on Advanced Communication Technology (ICACT), 2018, pp. 178–183. https://doi.org/10.23919/icact.2018.8323688
-
Shone N., Ngoc T.N. A deep learning approach to network intrusion detection. IEEE Transactions on Emerging Topics in Computational Intelligence, 2018, vol. 2, no. 1, pp. 41–50. https://doi.org/10.1109/tetci.2017.2772792
-
Zhang C., Ruan F., Yin L., Chen X., Zhai L., Liu F. A deep learning approach for network intrusion detection based on NSL-KDD dataset. Proc. of the IEEE 13th International Conference on Anti-counterfeiting, Security, and Identification (ASID), 2019, pp. 41–45. https://doi.org/10.1109/icasid.2019.8925239
-
Al-Qatf M., Lasheng Y., Al-Habib M., Al-Sabahi K. Deep learning approach combining sparse autoencoder with SVM for network intrusion detection. IEEE Access, 2018, vol. 6, pp. 2169–3536. https://doi.org/10.1109/access.2018.2869577
-
Zhang Y., Zhang Y., Zhang N., Xiao M. A network intrusion detection method based on deep learning with higher accuracy. Procedia Computer Science, 2020, vol. 174, pp. 50–54. https://doi.org/10.1016/j.procs.2020.06.055
-
Hinton G.E., Osindero S., Teh Y.-W. A fast learning algorithm for deep belief nets. Neural Computation, 2006, vol. 18, no. 7, pp. 1527–1554. https://doi.org/10.1162/neco.2006.18.7.1527
-
Ranzato M.A., Boureau Y.l., Cun Y.L. Sparse feature learning for deep belief networks. Proc. of the 21st Annual Conference on Neural Information Processing Systems (NIPS), 2008, pp. 1185–1192.
-
Gao N., Gao L., Gao Q., Wang H. An intrusion detection model based on deep belief networks. Proc. of the Second International Conference on Advanced Cloud and Big Data, 2014, pp. 247–252. https://doi.org/10.1109/cbd.2014.41
-
Coli G.O., Aina S., Okegbile S.D., LawalA.R., Oluwaranti A.I. DDoS attacks detection in the IoT using deep gaussian-bernoulli restricted boltzmann machine. Modern Applied Science, 2022, vol. 16, no. 2, pp. 12. https://doi.org/10.5539/mas.v16n2p12
-
Erpek T., Sagduyu Y.E., Shi Y. Deep learning for launching and mitigating wireless jamming attacks. IEEE Transactions on Cognitive Communications and Networking, 2019, vol. 5, no. 1, pp. 2–14. https://doi.org/10.1109/tccn.2018.2884910
-
Tang C., Luktarhan N., Zhao Y. SAAE-DNN: deep learning method on intrusion detection. Symmetry, 2020, vol. 12, no. 10, pp. 1695. https://doi.org/10.3390/sym12101695
-
Naseer S., Saleem Y., Khalid S., Bashir M.K., Han J., Iqbal M.M., Han K. Enhanced network anomaly detection based on deep neural networks. IEEE Access, 2018, vol. 6, pp. 48231–48246. https://doi.org/10.1109/access.2018.2863036
-
Liu G., Zhang J. CNID: Research of network intrusion detection based on convolutional neural network. Discrete Dynamics in Nature and Society, 2020, vol. 2020, pp. 1–11. https://doi.org/10.1155/2020/4705982
-
Al-Emadi S., Al-Mohannadi A., Al-Senaid F. Using deep learning techniques for network intrusion detection. IEEE International Conference on Informatics, IoT, and Enabling Technologies (ICIoT), 2020, pp. 171–176.https://doi.org/10.1109/iciot48696.2020.9089524
-
Graves A., Mohamed A.R., Hinton G. Speech recognition with deep recurrent neural networks. Proc. of the 2013 IEEE International Conference on Acoustics, Speech and Signal Processing, 2013, pp. 6645–6649. https://doi.org/10.1109/icassp.2013.6638947
-
Sutskever O., Vinyals Q.V., Le Q.V. Sequence to sequence learning with neural networks. Proc. of the 27th International Conference on Neural Information Processing Systems (NIPS'14), 2014, pp. 3104–3112.
-
Thilagam T., Aruna R. Intrusion detection for network based cloud computing by custom RC-NN and optimization. ICT Express, 2021, vol. 2, no. 4, pp. 512–520. https://doi.org/10.1016/j.icte.2021.04.006
-
Prabhakaran V., Kulandasamy A. Hybrid semantic deep learning architecture and optimal advanced encryption standard key management scheme for secure cloud storage and intrusion detection. Neural Computing and Applications, 2021, vol. 33, no. 21, pp. 14459–14479. https://doi.org/10.1007/s00521-021-06085-5
-
Al-Yaseen W.L. Multiagent System for an Adaptive Real Time Intrusion Detection System. LAP Lambert Academic Publishing, 2016, 272 p.