Menu
Publications
2025
2024
2023
2022
2021
2020
2019
2018
2017
2016
2015
2014
2013
2012
2011
2010
2009
2008
2007
2006
2005
2004
2003
2002
2001
Editor-in-Chief
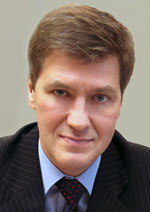
Nikiforov
Vladimir O.
D.Sc., Prof.
Partners
doi: 10.17586/2226-1494-2023-23-2-364-373
Monitoring the health status of the population by age groups
Read the full article

Article in Russian
For citation:
Abstract
For citation:
Ignatev N.A., Rakhimova M.A. Monitoring the health status of the population by age groups. Scientific and Technical Journal of Information Technologies, Mechanics and Optics, 2023, vol. 23, no. 2, pp. 364–373 (in Russian). doi: 10.17586/2226-1494-2023-23-2-364-373
Abstract
A multi-criteria method for selecting informative sets of different features for a quantitative assessment of the population’s health status in 14 age groups is considered. To compare samples from two classes (groups), it is proposed to form a unified description of objects according to two gradations of nominal features. The unified description is used to synthesize latent features and calculate the values of the compactness measure of class objects on the numerical axis. The transformation of quantitative features into nominal gradations is implemented according to the search criterion for the minimum coverage of their values by non-overlapping intervals. The values of the boundaries of the intervals and their number are determined by a recursive algorithm considering the objects belonging to classes. An important property of the transformation is the invariance to measurement scales. A formula is proposed for calculating the membership function of class objects for each feature gradation. Function values are used to unify object descriptions and calculate the stability index of a feature, regardless of its measurement scale. The unification of descriptions by two gradations does not change the stability index but increases the contribution of each gradation to the separation of class objects. The ranking of features about their stability was used both for individual samples and for a set of defined samples. The results of ranking over a set of samples were used to search for patterns in individual features and to form sets from them to calculate the values of latent features of objects. A set of thirteen data samples from representatives of two classes was formed as follows. The first class was represented by objects of the younger age group, and the second class — by objects of different age groups. A set of seven different types of features has been identified. For each of 13 samples, the values of latent features on this set and measures of compactness of class objects on the numerical axis were calculated. A monotonically non-decreasing sequence of values of measures of compactness of data samples that are invariant to the order of precedence of age groups is obtained. The property of monotonicity of sequence values is consistent with empirical estimates of the health state in the process of population aging.
Keywords: nonlinear transformations, membership functions, ranging of features, generalized estimates of objects
Acknowledgements. The work was carried out within the framework of the scientific research plan of the Department of Artificial Intelligence of the National University of Uzbekistan.
References
Acknowledgements. The work was carried out within the framework of the scientific research plan of the Department of Artificial Intelligence of the National University of Uzbekistan.
References
1. Shumakov V.I., Novoseltcev V.N., Sakharov M.P., Shtengold E.Sh. Simulation of The Body Physiological Systems. Moscow, Medicina Publ., 1971, 352 p. (in Russian)
2. Krivenko M.P. Data model selection in medical diagnostic tasks. Informatics and Applications, 2019, vol. 13, no. 4, pp. 27–29. (in Russian). https://doi.org/10.14357/19922264190404
3. Korepanova N.V. Machine learning for treatment optimization in subgroups of patients. Artificial Intelligence and Decision Making, 2018, no. 1, pp. 53–65. (in Russian)
4. Ignatyev N.A., Rakhimova M.A. Formation and analysis of sets of informative features of objects by pairs of classes. Artificial Intelligence and Decision Making, 2021, no. 4, pp. 18–26. (in Russian). https://doi.org/10.14357/20718594210402
5. Ignatev N.A., Zguralskaya E.N., Markovtseva M.V. Searching for hidden patterns that affect the overall patient survival with data mining. Scientific and Technical Information Processing, 2021, vol. 48, no. 6, pp. 461–466. (in Russian). https://doi.org/10.3103/S014768822106006X
6. Ignat’ev N.A. Computing generalized parameters and data mining. Automation and Remote Control, 2011, vol. 72, no. 5, pp. 1068–1074. https://doi.org/10.1134/S0005117911050146
7. Zguralskaya E. Sustainability of dividing data in intervals in the problems of recognition and searching for hidden laws. Izvestia of Samara Scientific Center of the Russian Academy of Sciences, 2018, vol. 20, no. 4-3, pp. 451–455. (in Russian)
8. Piatetsky-Shapiro G. Data mining and knowledge discovery 1996 to 2005: overcoming the hype and moving from «university» to «business» and «analytics». Data Mining and Knowledge Discovery, 2007, vol. 15, no. 1, pp. 99–105. https://doi.org/10.1007/s10618-006-0058-2
9. Joseph R. (2019, April 23). Ensemble methods: bagging, boosting and stacking. Understanding the key concepts of ensemble learning. Available at: https://towardsdatascience.com/ensemble-methods-bagging-boosting-and-stacking-c9214a10a205 (accessed: 25.12.2022).