Menu
Publications
2025
2024
2023
2022
2021
2020
2019
2018
2017
2016
2015
2014
2013
2012
2011
2010
2009
2008
2007
2006
2005
2004
2003
2002
2001
Editor-in-Chief
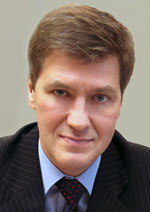
Nikiforov
Vladimir O.
D.Sc., Prof.
Partners
doi: 10.17586/2226-1494-2023-23-3-547-552
Time parameters linear approximation method in elastic systems
Read the full article

Article in Russian
For citation:
Abstract
For citation:
Martynchuk I.G., Zhmylev S.A. Time parameters linear approximation method in elastic systems. Scientific and Technical Journal of Information Technologies, Mechanics and Optics, 2023, vol. 23, no. 3, pp. 547–552 (in Russian). doi: 10.17586/2226-1494-2023-23-3-547-552
Abstract
In modern elastic systems, an important task is to predict changes in load processes. Estimating the load change rate helps to adapt the system structure in advance to maintain the quality of user experience. In modern solutions, little attention is paid to the analysis of the load change rate which directly affects how far in advance it is necessary to turn nodes on or off from the computing process. In most cases, these trigger intervals are set to pre-set static values. In order to determine the load process change rate, it is sufficient to solve the linear approximation problem over the interval of increase or decrease in the load function over time. The existing methods of linear approximation do not satisfy all the requirements for the elastic systems environments, which necessitates the development of own approximation method. A simplified linear approximation method ZFLAM is based on the calculation of the center of the initial data set mass as well as the average relative deviation of the ordered points along the ordinate axis from each other. The novelty of the proposed method lies in the simultaneous constant consumption of memory and the absence of operations with quadratic dependencies, which makes it possible to satisfy all the requirements for methods operating in elastic system environments. A two-dimensional plane point generator has been developed which makes it possible to obtain a set of ordered points scattered relative to a given line. The developed generator makes it possible to evaluate the accuracy of the proposed approximation method relative to other methods by calculating the average resulting deviation of the generated points from a given straight line. It was revealed that with a confidence probability of 0.95, with the maximum number of points in the original data set equal to 10,000, the reduction in the approximation execution time due to the developed method reaches 23 %. It was determined that with a confidence probability of 0.95, the value of the mean deviation for both methods in the framework of the experiments is the same. The obtained results can be applied in the elastic systems automatic scaling services in order to reduce the execution time of load processes change rate forecasts. The developed method, in contrast to the least squares method, is free from the disadvantage associated with operations with quadratic dependencies, which makes it possible to use it more widely in the conditions of limited bit grid of some architectures.
Keywords: linear approximation, elastic systems, automatic scaling, least squares, random sample consensus, principal component analysis
References
References
-
Aliev T.I., Rebezova M.I., Russ A.A. Statistical methods for monitoring travel agencies in the settlement system. Automatic Control and Computer Sciences, 2015, vol. 49, no. 6, pp. 321–327. https://doi.org/10.3103/s0146411615060024
-
Lorido-Botran T., Miguel-Alonso J., Lozano J.A. A review of auto-scaling techniques for elastic applications in cloud environments. Journal of Grid Computing, 2014, vol. 12, no. 4, pp. 559–592. https://doi.org/10.1007/s10723-014-9314-7
-
Nikravesh A.Y., Ajila S.A., Lung C.H. Towards an autonomic auto-scaling prediction system for cloud resource provisioning. Proc. of the IEEE/ACM 10th International Symposium on Software Engineering for Adaptive and Self-Managing Systems, 2015, pp. 35–45. https://doi.org/10.1109/seams.2015.22
-
Moore L.R., Bean K., Ellahi T. Transforming reactive auto-scaling into proactive auto-scaling. Proc. of the 3rd International Workshop on Cloud Data and Platforms (CloudDP '13), 2013, pp. 7–12. https://doi.org/10.1145/2460756.2460758
-
Aceto G., Botta A., de Donato W., Pescapè A. Cloud monitoring: A survey. Computer Networks, 2013, vol. 57, no. 9, pp. 2093–2115. https://doi.org/10.1016/j.comnet.2013.04.001
-
Portnoy M. Virtualization Essentials. John Wiley & Sons, 2012, 286 p.
-
Bogatyrev V.A., Bogatyrev A.V. Functional reliability of a real-time redundant computational process in cluster architecture systems. Automatic Control and Computer Sciences, 2015, vol. 49, no. 1, pp. 46–56. https://doi.org/10.3103/S0146411615010022
-
Bogatyrev V.A., Parshutina S.A., Poptcova N.A., Bogatyrev A.V. Efficiency of redundant service with destruction of expired and irrelevant request copies in real-time clusters. Communications in Computer and Information Science,2016, vol. 678, pp. 337–348. https://doi.org/10.1007/978-3-319-51917-3_30
-
Bogatyrev V.A., Bogatyrev S.V. Redundant data transmission using aggregated channels in real-time network. Journal of Instrument Engineering, 2016, vol. 59, no. 9, pp. 735–740.(in Russian). https://doi.org/10.17586/0021-3454-2016-59-9-735-740
-
Remondino F. Detectors and descriptors for photogrammetric applications. International Archives of the Photogrammetry, Remote Sensing and Spatial Information Sciences, 2006, vol. 36, no. 3, pp. 49–54.
-
Markovsky I., Van Huffel S. Overview of total least-squares methods. Signal Processing, 2007, vol. 87, no. 10, pp. 2283–2302. https://doi.org/10.1016/j.sigpro.2007.04.004
-
Fischler M.A., Bolles R.C. Random sample consensus: a paradigm for model fitting with applications to image analysis and automated cartography. Communications of the ACM, 1981, vol. 24, no. 6, pp. 381–395. https://doi.org/10.1145/358669.358692
-
Abdi H., Williams L.J. Principal component analysis. Wiley Interdisciplinary Reviews: Computational Statistics, 2010, vol. 2, no. 4, pp. 433–459. https://doi.org/10.1002/wics.101
-
Othman M.M., El-Mousa A. Internet of Things & Cloud Computing Internet of Things as a service approach. Proc. of the 11th International Conference on Information and Communication Systems (ICICS), 2020, pp. 318–323. https://doi.org/10.1109/icics49469.2020.239503