Menu
Publications
2025
2024
2023
2022
2021
2020
2019
2018
2017
2016
2015
2014
2013
2012
2011
2010
2009
2008
2007
2006
2005
2004
2003
2002
2001
Editor-in-Chief
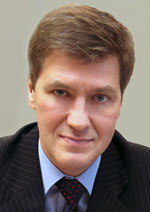
Nikiforov
Vladimir O.
D.Sc., Prof.
Partners
doi: 10.17586/2226-1494-2023-23-3-564-574
Exploring the possibility of predicting users’ career guidance preferences based on analysis of community topics and the gender in the online social network users’ profiles
Read the full article

Article in Russian
For citation:
Abstract
For citation:
Khlobystova A.O., Abramov M.V., Stoliarova V.F. Exploring the possibility of predicting users’ career guidance preferences based on analysis of community topics and the gender in the online social network users’ profiles. Scientific and Technical Journal of Information Technologies, Mechanics and Optics, 2023, vol. 23, no. 3, pp. 564–574 (in Russian). doi: 10.17586/2226-1494-2023-23-3-564-574
Abstract
The possibility of using digital traces of online social network users, using community themes as an example, to support decision-making in career guidance diagnostics is investigated. Statistical analysis was performed: descriptive statistics, z-criterion for comparing two groups, and regression analysis. The themes of users’ subscriptions to various communities available in the social network as well as the gender of the respondent and the number of friends in the social network indicated in the profile were analysed as digital user traces. The socio-professional orientation of the personality was assessed based on the results of the Holland test (edited by G.V. Rezapkina). The correlation between users’ digital traces expressed by the themes of subscriptions, and key indicators of socio-professional orientation reflected in the results of the Holland test was analyzed based on the pilot study conducted through an online social networking application. The statistical analysis confirmed the hypothesis that user interests, in the form of community themes, are related to the results of the Holland test. The hypotheses of existing differences in the groups of men and women in the studied attributes (the results of the Holland test and the leading themes of community subscriptions) were proved. By means of regression analysis among the group of women the correlation was found between the prevalence of the community theme “Education” and the key indicators: A (Artistic), E (Enterprising), I (Intellectual); prevalence of the theme “Lifestyle” and severity of the indicators: C (Conventional), I, A, E; “Mass Media” and indicator C. Among the group of men, a correlation was found between the prevalence of “Sports” subject matter and indicator E. The results of the work expanded the space of potential predictors of users’ vocational orientation. A foundation for large-scale research in quantifying and constructing predictive models of key occupational indicators based on users’ subscription topics has been obtained. The results are useful in the direction of developing an integrated approach to creating a recommendation system for user career guidance.
Keywords: career guidance, Holland theory, RIASEC, vocational personality types, career path, online social networks, community analysis, intellectual system, digital footprint analysis
Acknowledgements. This work was carried out within the framework of the project under the state assignment of SPC RAS SPIIRAS No. FFZF-2022-0003 (performing statistical analysis using Z-criterion to compare two groups, regression analysis); with the financial support of St. Petersburg State University, project No. 75254082 (statement of the research problem and hypotheses, review of relevant papers, descriptive statistics).
References
Acknowledgements. This work was carried out within the framework of the project under the state assignment of SPC RAS SPIIRAS No. FFZF-2022-0003 (performing statistical analysis using Z-criterion to compare two groups, regression analysis); with the financial support of St. Petersburg State University, project No. 75254082 (statement of the research problem and hypotheses, review of relevant papers, descriptive statistics).
References
-
Xie D., Cobb C.L. Career and vocational interests and abilities, assessment of. The Wiley Encyclopedia of Personality and Individual Differences: Models and Theories, 2020, pp. 493–497. https://doi.org/10.1002/9781118970843.ch152
-
Malik-Liévano B.,Álvarez-González B., Sánchez-García M.F., Irving B.A. International Perspectives on Research in Educational and Career Guidance. Springer Cham, 2020, 173 p. https://doi.org/10.1007/978-3-030-26135-1
-
Bogacheva E., Tatarenko F., Smetannikov I. Predicting vocational personality type from socio-demographic features using machine learning methods. CCRIS '20: Proc. of the 2020 1st International Conference on Control, Robotics and Intelligent System, 2020, pp. 93–98. https://doi.org/10.1145/3437802.3437819
-
Serbina G.N., Matsuta V.V., Goiko V.L. Analysis of the relationship between VK users’ psychological characteristics and subscriptions to communities with deviant content. Tomsk State University Journal, 2021, no. 467, pp. 164–169. (in Russian). https://doi.org/10.17223/15617793/467/20
-
Matcuta V.V., Goiko V.L., Feshchenko A.V., Uliakov M.A., Petrov E.Iu. Platform for psychological diagnostics with authorization of respondents in the social network "VKontakte". Certificate of the state computer program registration 2021612933. 2021. (in Russian)
-
Dağıstanlı Ö., Erbay H., Kör H., Yurttakal A.H. Reflection of people’s professions on social media platforms. Neural Computing and Applications, 2023,vol. 35, no. 7, pp. 5575–5586. https://doi.org/10.1007/s00521-022-07987-8
-
Van Huizen P., Mason R., Williams B. Exploring paramedicine student preferences using Holland's vocational theory: a cross‐sectional study. Nursing & Health Sciences, 2021, vol. 23, no. 4, pp. 818–824. https://doi.org/10.1111/nhs.12870
-
Ding Y.,Wang Q., Hourieh N., Yu Q. Vocational personality types in college engineering students in relation to academic achievement. Journal of Employment Counseling, 2020, vol. 57, no. 1, pp. 27–47. https://doi.org/10.1002/joec.12136
-
Earl J., Iskandar F., Elizondo F. Take a job, any job: Exploring the importance of matched interests to career paths and work satisfaction. Journal of Employment Counseling, 2019, vol. 56, no. 1, pp. 33–45. https://doi.org/10.1002/joec.12101
-
Gunawardena A.D.A., Meyer R.R., Kularatna T., Samaranayake S., Gunawardena R.H. Interest-aligned system for college degree planning and career path selection. SN Computer Science, 2022, vol. 3, no. 3, pp. 221. https://doi.org/10.1007/s42979-022-01107-y
-
Su J.-H., Liao Y.-W., Xu J.-Z., Zhao Y.-W. A personality-driven recommender system for cross-domain learning based on Holland code assessments. Sustainability, 2021, vol. 13, no. 7, pp. 3936. https://doi.org/10.3390/su13073936
-
Shminan A.S., Choi L.J., Barawi M.H., Hashim W.N.W., Andy H. InVesa 1.0: The conceptual framework of interactive virtual academic advisor system based on psychological profiles. Proc. of the 13th International Conference on Information & Communication Technology and System (ICTS), 2021, pp. 112–117. https://doi.org/10.1109/ICTS52701.2021.9608182
-
Usslepp N., Hübner N., Stoll G., Spengler M., Trautwein U., Nagengast B. RIASEC interests and the Big Five personality traits matter for life success—But do they already matter for educational track choices? Journal of Personality, 2020, vol. 88, no. 5, pp. 1007–1024. https://doi.org/10.1111/jopy.12547
-
Cioca L.-I., Bratu M.L. Sustainability of youth careers in Romania—Study on the correlation of students’ personal interests with the selected university field of study. Sustainability, 2020, vol. 13, no. 1, pp. 229. https://doi.org/10.3390/su13010229
-
Ethan Daphne C., Stanley M., Augustine V., Anggreainy M.S., Setiawan A.S. Developing an application to recommend major based on personality test using waterfall method. Proc. of the 5th International Conference of Computer and Informatics Engineering (IC2IE), 2022, pp. 220–224. https://doi.org/10.1109/IC2IE56416.2022.9970189
-
Rocconi L.M., Liu X., Pike G.R. The impact of person-environment fit on grades, perceived gains, and satisfaction: an application of Holland’s theory. Higher Education,2020, vol. 80, no. 5, pp. 857–874. https://doi.org/10.1007/s10734-020-00519-0
-
Sharma S., Behl R. Analysing the impact of social media on students’ academic performance: A comparative study of extraversion and introversion personality. Psychological Studies, 2022, vol. 67, no. 4, pp. 549–559. https://doi.org/10.1007/s12646-022-00675-6
-
Gonçalves A.P., Martins G.H., Salvador A.P., Machado G.M., Carvalho L.D.F., Ambiel R.A.M. Career adaptability and associations with personality traits and pathological traits utilizing network analysis. International Journal for Educational and Vocational Guidance, 2021, vol. 21, no. 2, pp. 379–394. https://doi.org/10.1007/s10775-020-09448-x
-
Kircaburun K., Alhabash S., Tosuntaş Ş.B., Griffiths M.D. Uses and gratifications of problematic social media use among university students: A simultaneous examination of the Big Five of personality traits, social media platforms, and social media use motives. International Journal of Mental Health and Addiction, 2020, vol. 18, no. 3, pp. 525–547. https://doi.org/10.1007/s11469-018-9940-6
-
Dandannavar P.S., Mangalwede S.R., Kulkarni P.M. Predicting the primary dominant personality trait of perceived leaders by mapping linguistic cues from social media data onto the big five model. Advances in Intelligent Systems and Computing,2021, vol. 1141, pp. 417–428. https://doi.org/10.1007/978-981-15-3383-9_37
-
Biswas K., Shivakumara P., Pal U., Lu T. A new ontology-based multimodal classification system for social media images of personality traits. Signal, Image and Video Processing, 2023, vol. 17, no. 2, pp. 543–551. https://doi.org/10.1007/s11760-022-02259-3
-
Hoff K.A., Chu C., Einarsdóttir S., Briley D.A., Hanna A., Rounds J. Adolescent vocational interests predict early career success: Two 12‐year longitudinal studies. Applied Psychology, 2022, vol. 71, no. 1, pp. 49–75. https://doi.org/10.1111/apps.12311
-
Oliveira Í.M., Porfeli E.J., do Céu Taveira M., Lee B. Children's career expectations and parents' jobs: intergenerational (dis)continuities. The Career Development Quarterly, 2020, vol. 68, no. 1, pp. 63–77. https://doi.org/10.1002/cdq.12213
-
Banov K., Krapic N., Kardum I. Do vocational interests matter for the selection of romantic partners? Evidence from variable‐and couple‐centered approaches. Applied Psychology, 2023, vol. 72, no. 2, pp. 697–717.https://doi.org/10.1111/apps.12396
-
Mayrand K., Sabourin S., Savard C. Vocational personality in romantic relationships: how vocational similarity contributes to couple functioning. Contemporary Family Therapy, 2023, vol. 45, no. 2, pp. 172–185. https://doi.org/10.1007/s10591-021-09620-7
-
Kim J., Park D., Shin Y.J. Friendship dynamics of career decision-making self-efficacy: a longitudinal social network approach. Current Psychology, 2022, in press. https://doi.org/10.1007/s12144-022-03887-1
-
Song Q.C., Shin H.J., Tang C., Hanna A., Behrend T. Investigating machine learning's capacity to enhance the prediction of career choices. Personnel Psychology, 2022, in press. https://doi.org/10.1111/peps.12529
-
José-García A., Sneyd A., Melro A., Ollagnier A., Tarling G., Zhang H., Stevenson M., Everson R., Arthur R. C3-IoC: A career guidance system for assessing student skills using machine learning and network visualisation. International Journal of Artificial Intelligence in Education, 2022, in press. https://doi.org/10.1007/s40593-022-00317-y
-
Holland J.L. Explorations of a theory of vocational choice and achievement: II. A four-year prediction study. Psychological Reports, 1963, vol. 12, no. 2, pp. 547–594. https://doi.org/10.2466/pr0.1963.12.2.547
-
Rezapkina G.V. Psychology and Profession Selection: Pre-Profile Training Program. Study Guide for psychologists and teachers. Moscow, Genesis Publ., 2005, 208 p. (in Russian)
-
Agresti A. Categorical Data Analysis. John Wiley & Sons, 2003.
-
Venables W.N., Ripley B.D. Modern Applied Statistics with S. Fourth Edition. New York, Springer, 2002, 498 p.
-
Korepanova A.A., Abramov M.V. Application of random forest in choosing a method of recovering the age of social network users. Scientific and Technical Information Processing, 2022, vol. 49, no. 5, pp. 317–324. https://doi.org/10.3103/s0147688222050057
-
Toropova A.V., Abramov M.V., Tulupyeva T.V. Machine learning of the bayesian belief network as a tool for evaluating the process frequency on social network data. Scientific and Technical Journal of Information Technologies, Mechanics and Optics, 2021, vol. 21, no. 5, pp. 727–737. (in Russian). https://doi.org/10.17586/2226-1494-2021-21-5-727-737
-
Khlobystova A., Abramov M., Korepanova A., Liapin N. Identification of predictors for estimation the intensity of relationships between users of online social networks. Lecture Notes in Networks and Systems, 2023, vol. 566, pp. 216–225. https://doi.org/10.1007/978-3-031-19620-1_21