Menu
Publications
2024
2023
2022
2021
2020
2019
2018
2017
2016
2015
2014
2013
2012
2011
2010
2009
2008
2007
2006
2005
2004
2003
2002
2001
Editor-in-Chief
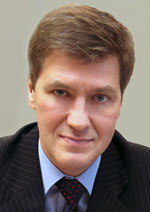
Nikiforov
Vladimir O.
D.Sc., Prof.
Partners
doi: 10.17586/2226-1494-2023-23-3-575-584
Blindness detection in diabetic retinopathy using Bayesian variant-based connected component algorithm in Keras and TensorFlow
Read the full article

Article in English
For citation:
Abstract
For citation:
Anantha Babu S., Murali S., Vijayan E., Anand M., Ramanathan L. Blindness detection in diabetic retinopathy using Bayesian variant-based connected component algorithm in Keras and TensorFlow. Scientific and TechnicalJournal of Information Technologies, Mechanics and Optics, 2023, vol. 23, no. 3, pp. 575–584. doi: 10.17586/2226-1494-2023-23-3-575-584
Abstract
The neuro-degenerative eye disease glaucoma is caused by an increase in eye pressure inside the retina. As the second- leading cause of blindness in the world, if an early diagnosis is not obtained, this can cause total blindness. Regarding this fundamental problem, there is a huge need to create a system that can function well without a lot of equipment, highly qualified medical personnel, and takes less time. The proposed modeling consists of three stages: pre-training, fine-tuning and inference. The probabilistic based pixel identification (Bayesian variant) predicts the severity of Diabetic Retinopathy (DR) which is diagnosed by the presence of visual cues, such as abnormal blood vessels, hard exudates, and cotton wool spots. The article combines machine learning, deep learning, and methods for image processing to predict the diagnosis images. The input picture is validated using Bayesian variant connected component architecture, and the brightest spot algorithm is applied to detect the Region of Interest (ROI). Moreover, the training sample calculated optic disc and optic cup are segmented with fundus photography ranges 0 to 4 using VGGNet16 architecture and SMOTE algorithm to detect DR stages of images and the proposed model using ensemble based ResNet with Efficient Net produces the excellent accuracy score of 93 % and predicted image Kappa coefficient (p < 0.01) 0.755 of the fundus retina image dataset.
Keywords: Bayesian variant, Keras and TensorFlow, ensemble learning, EfficientNet, ResNet
References
References
-
Stitt A.W., Curtis T.M., Chen M., Medina R.J., McKay G.J., Jenkins A., Gardiner T.A., Lyons T.J., Hammes H.-P., Simó R.,Lois N. The progress in understanding and treatment of diabetic retinopathy. Progress in Retinal and Eye Research,2016, vol. 51,pp. 156–186.https://doi.org/10.1016/j.preteyeres.2015.08.001
-
Mahmoud M.H., Alamery S., Fouad H., Altinawi A., Youssef A.E. An automatic detection system of diabetic retinopathy using a hybrid inductive machine learning algorithm. Personal and Ubiquitous Computing, 2021, pp. 1–15.https://doi.org/10.1007/s00779-020-01519-8
-
Dai L., Wu L., Li H., Cai C., Wu Q., Kong H., Liu R., Wang X., Hou X., Liu Y., Long X., Wen Y., Lu L., Shen Y., Chen Y., Shen D., Yang X., Zou H., Sheng B.,Jia W. A deep learning system for detecting diabetic retinopathy across the disease spectrum. Nature Communications,2021, vol. 12,no. 1, pp. 3242.https://doi.org/10.1038/s41467-021-23458-5
-
Leung D.Y.L., Tham C.C. Normal‐tension glaucoma: Current concepts and approaches‐A review. Clinical & Experimental Ophthalmology, 2022, vol. 50,no. 2,pp. 247–259.https://doi.org/10.1111/ceo.14043
-
French J.A., Lawson J.A., Yapici Z., Ikeda H., Polster T., Nabbout R.,Curatolo P., de Vries P.J., Dlugos D.J., Berkowitz N., Voi M., Peyrard S., Pelov D.,Franz D.N. Adjunctive everolimus therapy for treatment-resistant focal-onset seizures associated with tuberous sclerosis (EXIST-3): a phase 3, randomised, double-blind, placebo-controlled study. The Lancet,2016, vol. 388, no. 10056,pp. 2153–2163.https://doi.org/10.1016/s0140-6736(16)31419-2
-
Nazir T., Nawaz M., Rashid J., Mahum R., Masood M., Mehmood A., Ali F., Kim J., Kwon H.-Y.,Hussain A. Detection of diabetic eye disease from retinal images using a deep learning based CenterNet model. Sensors, 2021, vol. 21, no. 16, pp. 5283.https://doi.org/10.3390/s21165283
-
Barros D., Moura J.C., Freire C.R., Taleb A.C., Valentim R.A., Morais P.S. Machine learning applied to retinal image processing for glaucoma detection: review and perspective.BioMedical Engineering OnLine, 2020, vol. 19,no. 1,pp. 20.https://doi.org/10.1186/s12938-020-00767-2
-
Ray J.S.R., Babu S.A., James J.W., Vedaiyan R. ARIMA based Time Series Analysis: Forecast COVID-19 Most Vaccinated Process and Active Cases classify using Probability Distribution Curve Rates (ARIMAPDC). Proc. of the 2nd International Conference on Smart Electronics and Communication (ICOSEC), 2021, pp. 546–551. https://doi.org/10.1109/ICOSEC51865.2021.9591774
-
Raj R.J.S., Babu Anantha S., Jegatheesan A., Arul Xavier V.M. A GAN-based triplet FaceNet detection algorithm using deep face recognition for autism child. Lecture Notes in Electrical Engineering, 2022, vol. 905, pp. 177–187. https://doi.org/10.1007/978-981-19-2177-3_18
-
Babu S.A., Joshua Samuel Raj R., Varalatchoumy M., Gopila M., Febiyola Justin B.V. Novel approach for predicting COVID-19 symptoms using ARM based APRIORI algorithm. Proc. of the 6th International Conference on Computing Methodologies and Communication (ICCMC), 2022, pp. 1577–1580. https://doi.org/10.1109/ICCMC53470.2022.9753987
-
Khojasteh P., Aliahmad B., Kumar D.K. A novel color space of fundus images for automatic exudates detection. Biomedical Signal Processing and Control, 2019, vol. 49, pp. 240–249.https://doi.org/10.1016/j.bspc.2018.12.004
-
Kouassi Nzoughet J., Guehlouz K., Leruez S., Gohier P., Bocca C., Muller J., Blanchet O., Bonneau D., Simard G., Milea D., Procaccio V., Lenaers G., de la Barca J.M.C.,Reynier P. A data mining metabolomics exploration of glaucoma. Metabolites, 2020, vol. 10, no. 2,pp. 49.https://doi.org/10.3390/metabo10020049
-
Pang R., Labisi S.A., Wang N. Pigment dispersion syndrome and pigmentary glaucoma: overview and racial disparities. Graefe's Archive for Clinical and Experimental Ophthalmology, 2023, vol. 261, no. 3, pp. 601–614.https://doi.org/10.1007/s00417-022-05817-0
-
Wang X., Zhao Y., Pourpanah F. Recent advances in deep learning. International Journal of Machine Learning and Cybernetics, 2020, vol. 11,no. 4, pp. 747–750.https://doi.org/10.1007/s13042-020-01096-5
-
Domingues I., Pereira G., Martins P., Duarte H., Santos J., Abreu P.H. Using deep learning techniques in medical imaging: a systematic review of applications on CT and PET. Artificial Intelligence Review,2020, vol. 53, no. 6,pp. 4093–4160.https://doi.org/10.1007/s10462-019-09788-3
-
Aloysius N., Geetha M. A review on deep convolutional neural networks. Proc. of the 2017 International Conference on Communication and Signal Processing (ICCSP), 2017,pp. 0588–0592.https://doi.org/10.1109/iccsp.2017.8286426
-
Yu Z., Jiang X., Zhou F., Qin J., Ni D., Chen S., Wang T. Melanoma recognition in dermoscopy images via aggregated deep convolutional features. IEEE Transactions on Biomedical Engineering, 2019, vol. 66, no. 4, pp. 1006–1016.https://doi.org/10.1109/tbme.2018.2866166
-
Ooi A.Z.H., Embong Z., Abd Hamid A.I., Zainon R., Wang S.L., Ng T.F., Hamzah R.A., Teoh S.S.,Ibrahim H. Interactive blood vessel segmentation from retinal fundus image based on canny edge detector. Sensors, 2021, vol. 21, no. 19,pp. 6380.https://doi.org/10.3390/s21196380
-
Abdulsahib A.A., Mahmoud M.A., Mohammed M.A., Rasheed H.H., Mostafa S.A., Maashi M.S. Comprehensive review of retinal blood vessel segmentation and classification techniques: intelligent solutions for green computing in medical images, current challenges, open issues, and knowledge gaps in fundus medical images. Network Modeling Analysis in Health Informatics and Bioinformatics,2021, vol. 10,no. 1,pp. 20.https://doi.org/10.1007/s13721-021-00294-7
-
Artaechevarria X., Munoz-Barrutia A., Ortiz-de-Solorzano C. Combination strategies in multi-atlas image segmentation: application to brain MR data. IEEE Transactions on Medical Imaging, 2009, vol. 28, no. 8, pp. 1266–1277.https://doi.org/10.1109/tmi.2009.2014372
-
Jia S., Jiang S., Lin Z., Li N., Xu M., Yu S. A survey: Deep learning for hyperspectral image classification with few labeled samples. Neurocomputing, 2021, vol. 448,pp. 179–204.https://doi.org/10.1016/j.neucom.2021.03.035
-
Sazak Ç., Nelson C.J., Obara B. The multiscale bowler-hat transform for blood vessel enhancement in retinal images. Pattern Recognition, 2019, vol. 88,pp. 739–750.https://doi.org/10.1016/j.patcog.2018.10.011
-
Alshaikhli T., Liu W., Maruyama Y. Automated method of road extraction from aerial images using a deep convolutional neural network. Applied Sciences, 2019, vol. 9, no. 22,pp. 4825.https://doi.org/10.3390/app9224825