Menu
Publications
2025
2024
2023
2022
2021
2020
2019
2018
2017
2016
2015
2014
2013
2012
2011
2010
2009
2008
2007
2006
2005
2004
2003
2002
2001
Editor-in-Chief
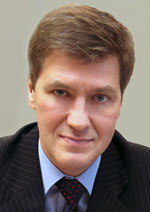
Nikiforov
Vladimir O.
D.Sc., Prof.
Partners
doi: 10.17586/2226-1494-2023-23-4-676-684
Application of Raman spectroscopy to study the inactivation process of bacterial microorganisms
Read the full article

Article in Russian
For citation:
Abstract
For citation:
Matveeva K.I., Kundalevich A.A., Kapitunova A.I., Zozulya A.S., Sukhikh S.A., Tsibulnikova A.V., Zyubin A.Yu., Samusev I.G. Application of Raman spectroscopy to study the inactivation process of bacterial microorganisms. Scientific and Technical Journal of Information Technologies, Mechanics and Optics, 2023, vol. 23, no. 4, pp. 676–684 (in Russian). doi: 10.17586/2226-1494-2023-23-4-676-684
Abstract
Raman spectroscopy (RS) is one of the promising approaches for structural and functional studies of various biological objects, including bacterial microorganisms. Both traditional biochemical tests and genetic methods which require expensive reagents, consumables and are time-consuming are used for bacterial analysis. Spectroscopic methods are positioned as noninvasive, highly sensitive, and requiring minimal sample preparation. In this work we investigated the possibility of using the RS method using optical sensors based on gold anisotropic nanoparticles. The applicability of the method was demonstrated by studying the effect of a broad-spectrum cephalosporin antibiotic and an extract of Viburnum opulus L (VO) on Escherichia coli (E. Coli) colonies. The studies were performed by Raman spectroscopy using a Virsa spectrometer (Renishaw). Raman signal amplification was carried out using two original optical sensors proposed by the authors. To create sensors, we used a chemical method of depositing gold nanostars on APTES-modified quartz glasses and a physical method for creating sensors based on anodizing titanium surfaces. The results of the study showed the high sensitivity and information content of the proposed method. The possibility of using the RS method for studying the inactivation of bacterial microorganisms is shown. Spectral Raman bands of E. Coli were determined and identified before and after exposure to VO extract and antibiotic as a control. A decrease in the intensity of spectral modes corresponding to amino acids and purine metabolites was found in the average Raman spectrum of E. Coli after exposure to VO extract. For the first time, a study of the antimicrobial effect of an aqueous extract of VO fruits was carried out by the method of Raman scattering. It has been shown that the use of plant extracts, including VO fruit extracts, to inactivate the vital activity of bacterial colonies is a promising approach to the search for new alternative antibacterial agents. The results obtained are in good agreement with the already known scientific studies and confirm the effectiveness of the proposed method.
Keywords: plasmon resonance, nanostars, SERS, Viburnum opulus L, Escherichia coli, antibiotics
Acknowledgements. The work was supported by the Ministry of Education and Science of the Russian Federation State Assignment no. FZWM-2020-0003 “Research of new materials and methods of plasma and phototherapy of cancer, dermatitis, and septic complications” 2020-2023.
References
Acknowledgements. The work was supported by the Ministry of Education and Science of the Russian Federation State Assignment no. FZWM-2020-0003 “Research of new materials and methods of plasma and phototherapy of cancer, dermatitis, and septic complications” 2020-2023.
References
- Giridhara P.K., Mishra C., Venkataramana R.K., Bukhari S.S., Dengel A.R. A study of various text augmentation techniques for relation classification in free text. Proc. of the 8th International Conference on Pattern Recognition Applications and Methods, 2019, pp. 360–367 https://doi.org/10.5220/0007311003600367
- Papadaki M. Data Augmentation Techniques for Legal Text Analytics. A thesis submitted to Athens University of Economics and Business in fulfillment of the requirements for the degree of Master in Data Science, 2017, 33 p.
- Zhang Z., Zweigenbaum P. GNEG: Graph-based negative sampling for word2vec. Proc. of the 56th Annual Meeting of the Association for Computational Linguistics. V. 2, 2018, pp. 566–571. https://doi.org/10.18653/v1/P18-2090
- Wei J., Zou K. EDA: Easy data augmentation techniques for boosting performance on text classification tasks. Proc. of the 2019 Conference on Empirical Methods in Natural Language Processing and the 9th International Joint Conference on Natural Language Processing (EMNLP-IJCNLP), 2018, pp. 6382–6388. https://doi.org/10.18653/v1/D19-1670
- Wu X., Xia Y., Zhu J., Wu L., Xie S., Fan Y., Qin T. mixSeq: A simple data augmentation method for neural machine translation. Proc. of the 18th International Conference on Spoken Language Translation (IWSLT 2021), 2021, pp. 192–197. https://doi.org/10.18653/v1/2021.iwslt-1.23
- Kumar V., Choudhary A., Cho E. Data augmentation using pre-trained transformer models. Proc. of the 2nd Workshop on Life-long Learning for Spoken Language Systems, 2020, pp. 18–26.
- Kobayashi S. Contextual augmentation: Data augmentation by words with paradigmatic relations. Proc. of the 2018 Conference of the North American Chapter of the Association for Computational Linguistics: Human Language Technologies, Volume 2 (Short Papers), 2018, pp. 452–457. https://doi.org/10.18653/v1/N18-2072
- Yu A., Dohan D., Luong M., Zhao R., Chen K., Norouzi M., Le Q. QANet: Combining local convolution with global self-attention for reading comprehension. Proc. of the ICLR Conference, 2018.
- Mehdi R., Meyer M., Goutal S. Text Data Augmentation: Towards better detection of spear-phishing emails. arXiv, 2020, arXiv:2007.02033. https://doi.org/10.48550/arXiv.2007.02033
- Edunov S., Ott M., Auli M., Grangier D. Understanding back-translation at scale. Proc. of the 2018 Conference on Empirical Methods in Natural Language Processing, 2018, pp. 489–500. https://doi.org/10.18653/v1/D18-1045
- Guo H., Mao Y., Zhang R. Augmenting data with mixup for sentence classification: An empirical study. arXiv, 2019, arXiv:1905.08941. https://doi.org/10.48550/arXiv.1905.08941
- Coulombe C. Text data augmentation made simple by leveraging NLP cloud APIs. arXiv, 2018, arXiv:1812.04718. https://doi.org/10.48550/arXiv.1812.04718
- Shen T., Lei T., Barzilay R., Jaakkola T. Style transfer from non-parallel text by cross-alignment. Advances in Neural Information Processing Systems, 2017, vol. 30.
- Yang S., Huang X., Lau J.H., Erfani S. Robust task-oriented dialogue generation with contrastive pre-training and adversarial filtering. Findings of the Association for Computational Linguistics (EMNLP 2022), 2022, pp. 1220–1234.
- Kovriguina L., Shilin I., Shipilo A., Putintseva A. Russian tagging and dependency parsing models for stanford CoreNLP natural language toolkit. Communications in Computer and Information Science, 2017, vol. 786, pp. 101–111. https://doi.org/10.1007/978-3-319-69548-8_8
- Matveev Y., Matveev A., Frolova O., Lyakso E., Ruban N. Automatic speech emotion recognition of younger school age children. Mathematics, 2022, vol. 10, no. 14, pp. 2373. https://doi.org/10.3390/math10142373
- Lyakso E., Frolova O., Matveev A., Matveev Y., Grigorev A., Makhnytkina O., Ruban N. Recognition of the emotional state of children with down syndrome by video, audio and text modalities: human and automatic. Lecture Notes in Computer Science, 2022, vol. 13721, pp. 438–450. https://doi.org/10.1007/978-3-031-20980-2_38
- Kim T., Vossen P. EmoBERTa: Speaker-Aware Emotion Recognition in Conversation with RoBERTa. arXiv, 2021, arXiv:2108.12009. https://doi.org/10.48550/arXiv.2108.12009
- Song X., Zang L., Zhang R., Hu S., Huang L. Emotionflow: Capture the dialogue level emotion transitions. Proc. of the ICASSP 2022 - 2022 IEEE International Conference on Acoustics, Speech and Signal Processing (ICASSP), 2022, pp. 8542–8546. https://doi.org/10.1109/ICASSP43922.2022.9746464
- Shen W., Chen J., Quan X., Xie Z. DialogXL: All-in-One XLNet for multi-party conversation emotion recognition. Proceedings of the AAAI Conference on Artificial Intelligence, 2021, vol. 35, no. 15, pp. 13789–13797 https://doi.org/10.1609/aaai.v35i15.17625
- Shen W., Wu S., Yang Y., Quan X. Directed acyclic graph network for conversational emotion recognition. Proc. of the 59th Annual Meeting of the Association for Computational Linguistics and the 11th International Joint Conference on Natural Language Processing (Volume 1: Long Papers), 2021, pp. 1551–1560.