Menu
Publications
2024
2023
2022
2021
2020
2019
2018
2017
2016
2015
2014
2013
2012
2011
2010
2009
2008
2007
2006
2005
2004
2003
2002
2001
Editor-in-Chief
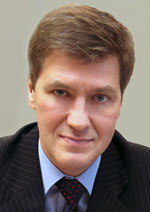
Nikiforov
Vladimir O.
D.Sc., Prof.
Partners
doi: 10.17586/2226-1494-2023-23-4-743-749
Text augmentation preserving persona speech style and vocabulary
Read the full article

Article in Russian
For citation:
Abstract
For citation:
Matveeva A.A., Makhnytkina O.V. Text augmentation preserving persona speech style and vocabulary. Scientific and Technical Journal of Information Technologies, Mechanics and Optics, 2023, vol. 23, no. 4, pp. 743–749 (in Russian). doi: 10.17586/2226-1494-2023-23-4-743-749
Abstract
Currently, various natural language processing tasks often require large data sets. However, for many tasks, collecting large datasets is quite tedious and expensive, and requires the involvement of experts. An increase in the amount of data can be achieved using methods of data augmentation, however, the use of classical approaches can lead to the inclusion of phrases in the data corpus that differ in the speech style and vocabulary of the target person, which can lead to both a change in the target class as well as the appearance of replicas with unnatural vocabulary use and lack of meaning. In this context, a new method for test data enrichment is proposed that takes into account the person’s style and vocabulary. In this article, a new method for expanding text data that preserves individual language features and vocabulary is proposed. The core of the method is to create individual templates for each person based on the analysis of syntactic trees of propositions and then to create new replicas according to the generated templates. The method was tested on the task of assessing the user’s emotional state in a dialogue. The search was carried out for data sets in English and Russian. The proposed method made it possible to improve the quality of solving these problems for both the English and Russian languages. Up to a 2 % increase in accuracy and weighted F1 metrics has been noted for various models. The results of the work can be applied to improve the accuracy and weighted F1 metrics of models designed to solve various problems for the English and Russian languages.
Keywords: text data augmentation, emotion recognition, statement valence evaluation
Acknowledgements. This research was supported by a grant from the Russian Science Foundation (22-11-00128 https://www.rscf.ru/ project/22-11-00128/).
References
Acknowledgements. This research was supported by a grant from the Russian Science Foundation (22-11-00128 https://www.rscf.ru/ project/22-11-00128/).
References
- Giridhara P.K., Mishra C., Venkataramana R.K., Bukhari S.S., Dengel A.R. A study of various text augmentation techniques for relation classification in free text. Proc. of the 8th International Conference on Pattern Recognition Applications and Methods, 2019, pp. 360–367 https://doi.org/10.5220/0007311003600367
- Papadaki M. Data Augmentation Techniques for Legal Text Analytics. A thesis submitted to Athens University of Economics and Business in fulfillment of the requirements for the degree of Master in Data Science, 2017, 33 p.
- Zhang Z., Zweigenbaum P. GNEG: Graph-based negative sampling for word2vec. Proc. of the 56th Annual Meeting of the Association for Computational Linguistics. V. 2, 2018, pp. 566–571. https://doi.org/10.18653/v1/P18-2090
- Wei J., Zou K. EDA: Easy data augmentation techniques for boosting performance on text classification tasks. Proc. of the 2019 Conference on Empirical Methods in Natural Language Processing and the 9th International Joint Conference on Natural Language Processing (EMNLP-IJCNLP), 2018, pp. 6382–6388. https://doi.org/10.18653/v1/D19-1670
- Wu X., Xia Y., Zhu J., Wu L., Xie S., Fan Y., Qin T. mixSeq: A simple data augmentation method for neural machine translation. Proc. of the 18th International Conference on Spoken Language Translation (IWSLT 2021), 2021, pp. 192–197. https://doi.org/10.18653/v1/2021.iwslt-1.23
- Kumar V., Choudhary A., Cho E. Data augmentation using pre-trained transformer models. Proc. of the 2nd Workshop on Life-long Learning for Spoken Language Systems, 2020, pp. 18–26.
- Kobayashi S. Contextual augmentation: Data augmentation by words with paradigmatic relations. Proc. of the 2018 Conference of the North American Chapter of the Association for Computational Linguistics: Human Language Technologies, Volume 2 (Short Papers), 2018, pp. 452–457. https://doi.org/10.18653/v1/N18-2072
- Yu A., Dohan D., Luong M., Zhao R., Chen K., Norouzi M., Le Q. QANet: Combining local convolution with global self-attention for reading comprehension. Proc. of the ICLR Conference, 2018.
- Mehdi R., Meyer M., Goutal S. Text Data Augmentation: Towards better detection of spear-phishing emails. arXiv, 2020, arXiv:2007.02033. https://doi.org/10.48550/arXiv.2007.02033
- Edunov S., Ott M., Auli M., Grangier D. Understanding back-translation at scale. Proc. of the 2018 Conference on Empirical Methods in Natural Language Processing, 2018, pp. 489–500. https://doi.org/10.18653/v1/D18-1045
- Guo H., Mao Y., Zhang R. Augmenting data with mixup for sentence classification: An empirical study. arXiv, 2019, arXiv:1905.08941. https://doi.org/10.48550/arXiv.1905.08941
- Coulombe C. Text data augmentation made simple by leveraging NLP cloud APIs. arXiv, 2018,arXiv:1812.04718. https://doi.org/10.48550/arXiv.1812.04718
- Shen T., Lei T., Barzilay R., Jaakkola T. Style transfer from non-parallel text by cross-alignment. Advances in Neural Information Processing Systems, 2017, vol. 30.
- Yang S., Huang X., Lau J.H., Erfani S. Robust task-oriented dialogue generation with contrastive pre-training and adversarial filtering. Findings of the Association for Computational Linguistics (EMNLP 2022), 2022, pp. 1220–1234.
- Kovriguina L., Shilin I., Shipilo A., Putintseva A. Russian tagging and dependency parsing models for stanford CoreNLP natural language toolkit. Communications in Computer and Information Science, 2017, vol. 786, pp. 101–111. https://doi.org/10.1007/978-3-319-69548-8_8
- Matveev Y., Matveev A., Frolova O., Lyakso E., Ruban N. Automatic speech emotion recognition of younger school age children. Mathematics, 2022, vol. 10, no. 14, pp. 2373. https://doi.org/10.3390/math10142373
- Lyakso E., Frolova O., Matveev A., Matveev Y., Grigorev A., Makhnytkina O., Ruban N. Recognition of the emotional state of children with down syndrome by video, audio and text modalities: human and automatic. Lecture Notes in Computer Science, 2022, vol. 13721, pp. 438–450. https://doi.org/10.1007/978-3-031-20980-2_38
- Kim T., Vossen P. EmoBERTa: Speaker-Aware Emotion Recognition in Conversation with RoBERTa. arXiv, 2021, arXiv:2108.12009. https://doi.org/10.48550/arXiv.2108.12009
- Song X., Zang L., Zhang R., Hu S., Huang L. Emotionflow: Capture the dialogue level emotion transitions. Proc. of the ICASSP 2022 - 2022 IEEE International Conference on Acoustics, Speech and Signal Processing (ICASSP), 2022, pp. 8542–8546. https://doi.org/10.1109/ICASSP43922.2022.9746464
- Shen W., Chen J., Quan X., Xie Z. DialogXL: All-in-One XLNet for multi-party conversation emotion recognition. Proceedings of the AAAI Conference on Artificial Intelligence, 2021, vol. 35, no. 15, pp. 13789–13797 https://doi.org/10.1609/aaai.v35i15.17625
- Shen W., Wu S., Yang Y., Quan X. Directed acyclic graph network for conversational emotion recognition. Proc. of the 59th Annual Meeting of the Association for Computational Linguistics and the 11th International Joint Conference on Natural Language Processing (Volume 1: Long Papers), 2021, pp. 1551–1560.