Menu
Publications
2025
2024
2023
2022
2021
2020
2019
2018
2017
2016
2015
2014
2013
2012
2011
2010
2009
2008
2007
2006
2005
2004
2003
2002
2001
Editor-in-Chief
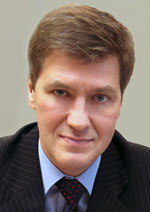
Nikiforov
Vladimir O.
D.Sc., Prof.
Partners
doi: 10.17586/2226-1494-2023-23-4-767-775
Neural network-based method for visual recognition of driver’s voice commands using attention mechanism
Read the full article

Article in Russian
For citation:
Abstract
For citation:
Axyonov A.A., Ryumina E.V., Ryumin D.A., Ivanko D.V., Karpov A.A. Neural network-based method for visual recognition of driver’s voice commands using attention mechanism. Scientific and Technical Journal of Information Technologies, Mechanics and Optics, 2023, vol. 23, no. 4, pp. 767–775 (in Russian). doi: 10.17586/2226-1494-2023-23-4-767-775
Abstract
Visual speech recognition or automated lip-reading systems actively apply to speech-to-text translation. Video data proves to be useful in multimodal speech recognition systems, particularly when using acoustic data is difficult or not available at all. The main purpose of this study is to improve driver command recognition by analyzing visual information to reduce touch interaction with various vehicle systems (multimedia and navigation systems, phone calls, etc.) while driving. We propose a method of automated lip-reading the driver’s speech while driving based on a deep neural network of 3DResNet18 architecture. Using neural network architecture with bi-directional LSTM model and attention mechanism allows achieving higher recognition accuracy with a slight decrease in performance. Two different variants of neural network architectures for visual speech recognition are proposed and investigated. When using the first neural network architecture, the result of voice recognition of the driver was 77.68 %, which was lower by 5.78 % than when using the second one the accuracy of which was 83.46 %. Performance of the system which is determined by a real-time indicator RTF in the case of the first neural network architecture is equal to 0.076, and the second — RTF is 0.183 which is more than two times higher. The proposed method was tested on the data of multimodal corpus RUSAVIC recorded in the car. Results of the study can be used in systems of audio-visual speech recognition which is recommended in high noise conditions, for example, when driving a vehicle. In addition, the analysis performed allows us to choose the optimal neural network model of visual speech recognition for subsequent incorporation into the assistive system based on a mobile device.
Keywords: driver’s voice commands, visual speech recognition, automatic lip reading, machine learning, CNN, LSTM, attention mechanisms
Acknowledgements. The study was supported by the Russian Foundation for Basic Research (project no. 19-29-09081-mk), the leading scientific school of the Russian Federation (grant no. NSh-17.2022.1.6) and at the expense of state funding, topic FFZF-2022-0005.
References
Acknowledgements. The study was supported by the Russian Foundation for Basic Research (project no. 19-29-09081-mk), the leading scientific school of the Russian Federation (grant no. NSh-17.2022.1.6) and at the expense of state funding, topic FFZF-2022-0005.
References
- Lin S.C., Hsu C.H., Talamonti W., Zhang Y., Oney S., Mars J., Tang L. Adasa: A conversational in-vehicle digital assistant for advanced driver assistance features. Proc. of the 31st Annual ACM Symposium on User Interface Software and Technology, 2018, pp. 531–542. https://doi.org/10.1145/3242587.3242593
- Lee B., Hasegawa-Johnson M., Goudeseune C., Kamdar S., Borys S., Liu M., Huang T. AVICAR: Audio-visual speech corpus in a car environment. Proc. of the 8th International Conference on Spoken Language Processing, 2004, pp. 2489–2492. https://doi.org/10.21437/Interspeech.2004-424
- Ivanko D., Ryumin D., Kashevnik A., Axyonov A., Karpov A. Visual speech recognition in a driver assistance system. Proc. of the 30th European Signal Processing Conference (EUSIPCO), 2022, pp. 1131–1135. https://doi.org/10.23919/EUSIPCO55093.2022.9909819
- Xu B., Wang J., Lu C., Guo Y. Watch to listen clearly: Visual speech enhancement driven multi-modality speech recognition. Proc. of the IEEE/CVF Winter Conference on Applications of Computer Vision (WACV), 2020, pp. 1637–1646. https://doi.org/10.1109/wacv45572.2020.9093314
- Afouras T., Chung, J.S., Senior A., Vinyals O., Zisserman A. Deep audio-visual speech recognition. IEEE Transactions on Pattern Analysis and Machine Intelligence, 2022, vol. 44, no. 12, pp. 8717–8727. https://doi.org/10.1109/TPAMI.2018.2889052
- Kukharev G.A., Matveev Yu.N., Oleinik A.L. Mutual image transformation algorithms for visual information processing and retrieval. Scientific and Technical Journal of Information Technologies, Mechanics and Optics, 2017, vol. 17, no. 1, pp. 62–74. (in Russian). https://doi.org/10.17586/2226-1494-2017-17-1-62-74
- Shi B., Hsu W.N., Mohamed A. Robust self-supervised audio-visual speech recognition. Proc. of the International Conference INTERSPEECH, 2022, pp. 2118–2122. https://doi.org/10.21437/interspeech.2022-99
- Chand H.V., Karthikeyan J. CNN based driver drowsiness detection system using emotion analysis. Intelligent Automation & Soft Computing, 2022, vol. 31, no. 2, pp. 717–728. https://doi.org/10.32604/iasc.2022.020008
- Ivanko D., Kashevnik A., Ryumin D., Kitenko A., Axyonov A., Lashkov I., Karpov A. MIDriveSafely: Multimodal interaction for drive safely. Proc. of the 2022 International Conference on Multimodal Interaction (ICMI), 2022, pp. 733–735. https://doi.org/10.1145/3536221.3557037
- Biswas A., Sahu P.K., Chandra M. Multiple cameras audio visual speech recognition using active appearance model visual features in car environment. International Journal of Speech Technology, 2016, vol. 19, no. 1, pp. 159–171. https://doi.org/10.1007/s10772-016-9332-x
- Nambi A.U., Bannur S., Mehta I., Kalra H., Virmani A., Padmanabhan V.N., Bhandari R., Raman B. HAMS: Driver and driving monitoring using a smartphone. Proc. of the 24th Annual International Conference on Mobile Computing and Networking, 2018, pp. 840–842. https://doi.org/10.1145/3241539.3267723
- Kashevnik A., Lashkov I., Gurtov A. Methodology and mobile application for driver behavior analysis and accident prevention. IEEE Transactions on Intelligent Transportation Systems, 2020, vol. 21, no. 6, pp. 2427–2436. https://doi.org/10.1109/TITS.2019.2918328
- Jang S.W., Ahn B. Implementation of detection system for drowsy driving prevention using image recognition and IoT. Sustainability, 2020, vol. 12, no. 7, pp. 3037. https://doi.org/10.3390/su12073037
- Mishra R.K., Urolagin S., Jothi J.A.A., Gaur P. Deep hybrid learning for facial expression binary classifications and predictions. Image and Vision Computing, 2022, vol. 128, pp. 104573. https://doi.org/10.1016/j.imavis.2022.104573
- Sunitha G., Geetha K., Neelakandan S., Pundir A.K.S., Hemalatha S., Kumar V. Intelligent deep learning based ethnicity recognition and classification using facial images. Image and Vision Computing, 2022, vol. 121, pp. 104404. https://doi.org/10.1016/j.imavis.2022.104404
- Yuan Y., Tian C., Lu X. Auxiliary loss multimodal GRU model in audio-visual speech recognition. IEEE Access, 2018, vol. 6, pp. 5573–5583. https://doi.org/10.1109/ACCESS.2018.2796118
- Hou J.C., Wang S.S., Lai Y.H., Tsao Y., Chang H.W., Wang H.M. Audio-visual speech enhancement using multimodal deep convolutional neural networks. IEEE Transactions on Emerging Topics in Computational Intelligence, 2018, vol. 2, no. 2, pp. 117–128. https://doi.org/10.1109/TETCI.2017.2784878
- Chan Z.M., Lau C.Y., Thang K.F. Visual speech recognition of lips images using convolutional neural network in VGG-M model. Journal of Information Hiding and Multimedia Signal Processing, 2020, vol. 11, no. 3, pp. 116–125.
- Zhu X., Cheng D., Zhang Z., Lin S., Dai J. An empirical study of spatial attention mechanisms in deep networks. Proc. of the IEEE/CVF International Conference on Computer Vision, 2019, pp. 6688–6697. https://doi.org/10.1109/iccv.2019.00679
- Bhaskar S., Thasleema T.M. LSTM model for visual speech recognition through facial expressions. Multimedia Tools and Applications, 2023, vol. 82, no. 4, pp. 5455–5472. https://doi.org/10.1007/s11042-022-12796-1
- Hori T., Cho J., Watanabe S. End-to-end Speech recognition with word-based RNN language models. Proc. of the 2018 IEEE Spoken Language Technology Workshop (SLT), 2018, pp. 389–396. https://doi.org/10.1109/SLT.2018.8639693
- Serdyuk D.D., Braga O.P.F., Siohan O. Transformer-based video front-ends for audio-visual speech recognition for single and multi-person video. Proc. of the INTERSPEECH, 2022, pp. 2833–2837. https://doi.org/10.21437/interspeech.2022-10920
- Chen C.F.R., Fan Q., Panda R. CrossViT: Cross-attention multi-scale vision transformer for image classification. Proc. of the IEEE/CVF International Conference on Computer Vision (ICCV), 2021, pp. 347–356. https://doi.org/10.1109/iccv48922.2021.00041
- Pan S.J., Yang Q. A survey on transfer learning. IEEE Transactions on Knowledge and Data Engineering, 2010, vol. 22, no. 10, pp. 1345–1359. https://doi.org/10.1109/tkde.2009.191
- Romanenko A.N., Matveev Yu.N., Minker W. Knowledge transfer for Russian conversational telephone automatic speech recognition. Scientific and Technical Journal of Information Technologies, Mechanics and Optics, 2018, vol. 18, no. 2, pp. 236–242 (in Russian). https://doi.org/10.17586/2226-1494-2018-18-2-236-242
- Sui C., Bennamoun M., Togneri R. Listening with your eyes: towards a practical visual speech recognition system using deep boltzmann machines. Proc. of the IEEE International Conference on Computer Vision (ICCV), 2015, pp. 154–162. https://doi.org/10.1109/iccv.2015.26
- Ahmed N., Natarajan T., Rao K.R. Discrete cosine transform. IEEE Transactions on Computers, 1974, vol. C-23, no. 1, pp. 90–93. https://doi.org/10.1109/T-C.1974.223784
- Xanthopoulos P., Pardalos P.M., Trafalis T.B. Linear discriminant analysis. Robust Data Mining, Springer New York, 2013, pp. 27–33. https://doi.org/10.1007/978-1-4419-9878-1_4
- Tomashenko N.A., Khokhlov Yu.Yu., Larcher A., Estève Ya., Matveev Yu.N. Gaussian mixture models for adaptation of deep neural network acoustic models in automatic speech recognition systems. Scientific and Technical Journal of Information Technologies, Mechanics and Optics, 2016, vol. 16, no. 6, pp. 1063–1072. (in Russian). https://doi.org/10.17586/2226-1494-2016-16-6-1063-1072
- Ma P., Petridis S., Pantic M. End-to-end audio-visual speech recognition with conformers. Proc. of the IEEE International Conference on Acoustics, Speech and Signal Processing (ICASSP), 2021, pp. 7613–7617. https://doi.org/10.1109/ICASSP39728.2021.9414567
- Ryumin D., Ivanko D., Ryumina E. Audio-visual speech and gesture recognition by sensors of mobile devices. Sensors, 2023, vol. 23, no. 4, pp. 2284. https://doi.org/10.3390/s23042284
- Huang J., Kingsbury B. Audio-visual deep learning for noise robust speech recognition. Proc. of the IEEE International Conference on Acoustics, Speech and Signal Processing, 2013, pp. 7596–7599. https://doi.org/10.1109/ICASSP.2013.6639140
- Ivanko D., Ryumin D., Kashevnik A., Axyonov A., Kitenko A., Lashkov I., Karpov A. DAVIS: Driver’s audio-visual speech recognition. Proc. of the International Conference INTERSPEECH, 2022, pp. 1141–1142.
- Zhou P., Yang W., Chen W., Wang Y., Jia J. Modality attention for end-to-end audio-visual speech recognition. Proc. of the IEEE International Conference on Acoustics, Speech and Signal Processing (ICASSP), 2019, pp. 6565–6569. https://doi.org/10.1109/ICASSP.2019.8683733
- Ivanko D., Axyonov A., Ryumin D., Kashevnik A., Karpov A. RUSAVIC Corpus: Russian audio-visual speech in cars. Proc. of the 13th Language Resources and Evaluation Conference (LREC), 2022, pp. 1555–1559.
- Kashevnik A., Lashkov I., Axyonov A., Ivanko D., Ryumin D., Kolchin A., Karpov A. Multimodal corpus design for audio-visual speech recognition in vehicle cabin. IEEE Access, 2021, vol. 9, pp. 34986–35003. https://doi.org/10.1109/ACCESS.2021.3062752
- Lugaresi C., Tang J., Nash H., McClanahan C., Uboweja E., Hays M., Zhang F., Chang C.-L., Yong M., Lee J., Chang W.-T., Hua W., Georg M., Grundmann M. MediaPipe: A framework for perceiving and processing reality. Proc. of the 3rd Workshop on Computer Vision for AR/VR at IEEE Computer Vision and Pattern Recognition (CVPR), 2019, vol. 2019, pp. 1–4.
- Zhang H., Cisse M., Dauphin Y.N., Lopez-Paz D. MixUp: Beyond empirical risk minimization. Proc. of the ICLR Conference, 2018, pp. 1–13.
- Feng D., Yang S., Shan S. An efficient software for building LIP reading models without pains. Proc. of the IEEE International Conference on Multimedia & Expo Workshops (ICMEW), 2021, pp. 1–2. https://doi.org/10.1109/ICMEW53276.2021.9456014
- Kim M., Hong J., Park S.J., Ro Y.M. Multi-modality associative bridging through memory: speech sound recollected from face video. Proc. of the IEEE/CVF International Conference on Computer Vision (ICCV), 2021, pp. 296–306. https://doi.org/10.1109/iccv48922.2021.00036
- Zhong Z., Lin Z.Q., Bidart R., Hu X., Daya I.B., Li Z., Zheng W., Li J., Wong A. Squeeze-and-attention networks for semantic segmentation. Proc. of the IEEE/CVF Conference on Computer Vision and Pattern Recognition (CVPR), 2020, pp. 13065–13074. https://doi.org/10.1109/cvpr42600.2020.01308
- Axyonov A.A., Ryumin D.A., Kashevnik A.M., Ivanko D.V., Karpov A.A. Method for visual analysis of driver's face for automatic lip-reading in the wild. Computer Optic, 2022, vol. 46, no. 6, pp. 955–962. (in Russian). https://doi.org/10.18287/2412-6179-CO-1092