Menu
Publications
2025
2024
2023
2022
2021
2020
2019
2018
2017
2016
2015
2014
2013
2012
2011
2010
2009
2008
2007
2006
2005
2004
2003
2002
2001
Editor-in-Chief
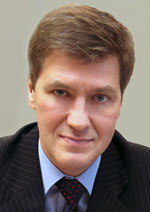
Nikiforov
Vladimir O.
D.Sc., Prof.
Partners
doi: 10.17586/2226-1494-2023-23-4-854-857
RuLegalNER: a new dataset for Russian legal named entities recognition
Read the full article

Article in English
For citation:
Abstract
For citation:
Shaheen Z., Mouromtsev D.I., Postny I. RuLegalNER: a new dataset for Russian legal named entities recognition. Scientific and Technical Journal of Information Technologies, Mechanics and Optics, 2023, vol. 23, no. 4, pp. 854–857. doi: 10.17586/2226-1494-2023-23-4-854-857
Abstract
We address the scarcity of datasets specifically tailored for legal NER in the Russian language and investigate the generalization capabilities of models towards unseen named entities. A rule-based program developed by legal experts at Tag-Consulting Company was employed to automatically annotate legal texts and create the RuLegalNER dataset. Part of the named entities only exists in the development and test splits, and they are unseen in the training set. RuBERT was utilized as the base architecture for experimental evaluation. Two different architectural extensions were explored: RuBERT with CRF and RuBERT with adapters. These architectures were used to train and evaluate NER models on the RuLegalNER dataset. Utilize RuLegalNER to train and evaluate legal NER models, enhancing performance in the legal domain and studying generalization on unseen entities. A published version of RuLegalNER is presented with detailed statistics and demonstration of the usefulness of RuLegalNER by evaluating modern architectures.
Keywords: legal named entity recognition, natural language processing, information extraction, low-resource languages, transfer learning, transformers
References
References
- Weston L., Tshitoyan V., Dagdelen J., Kononova O., Trewartha A., Persson K.A., Ceder G., Jain A.. Named entity recognition and normalization applied to large-scale information extraction from the materials science literature. Journal of Chemical Information and Modeling, 2019, vol. 59, no. 9, pp. 3692–3702. https://doi.org/10.1021/acs.jcim.9b00470
- Angelidis I., Chalkidis I., Koubarakis M. Named entity recognition, linking and generation for greek legislation. Legal Knowledge and Information Systems, 2018, vol. 313, pp. 1–10.
- Zhu Y., Ye Y., Li M., Zhang J., Wu O. Investigating annotation noise for named entity recognition. Neural Computing and Applications, 2023, vol. 35, no. 1, pp. 993–1007. https://doi.org/10.1007/s00521-022-07733-0
- Vlasova N.A., Suleymanova E.A., Trofimov I.V. Report on Russian corpus for personal name retrieval. Proceedings of Computational and Cognitive Linguistics, TEL, 2014, pp. 36–40.
- Starostin A.S., Bocharov V.V., Alexeeva S.V., Bodrova A.A., Chuchunkov A.S., Dzhumaev S.S., Efimenko I.V., Granovsky D.V., Khoroshevsky V.F., Krylova I.V., Nikolaeva M.A., Smurov I.M., Toldova S.Y. Factrueval 2016: evaluation of named entity recognition and fact extraction systems for Russian. Proc. of the International Conference “Dialogue 2016”, 2016, pp. 702–720.
- Gareev R., Tkachenko M., Solovyev V., Simanovsky A., Ivanov V. Introducing baselines for russian named entity recognition. Lecture Notes in Computer Science, 2013, vol. 7816, pp. 329–342. https://doi.org/10.1007/978-3-642-37247-6_27
- Loukachevitch N., Artemova E., Batura T., Braslavski P., Denisov I., Ivanov V., Manandhar S., Pugachev A., Tutubalina E. Nerel: A Russian dataset with nested named entities, relations and events. Proc. of the Recent Advances in Natural Language Processing, 2021, pp. 876–885 https://doi.org/10.26615/978-954-452-072-4_100
- Kuratov Y., Arkhipov M. Adaptation of deep bidirectional multilingual transformers for Russian language. Computational Linguistics and Intellectual Technologies: Proceedings of the International Conference “Dialogue 2019”, 2019.
- Houlsby N., Giurgiu A., Jastrzebski S., Morrone B., De Laroussilhe Q., Gesmundo A., Attariyan M., Gelly S. Parameter-efficient transfer learning for NLP. Proc. of the 36th International Conference on Machine Learning, 2019, pp. 2790–2799.
- Panchendrarajan R., Amaresan A. Bidirectional LSTM-CRF for named entity recognition. Proc. of the 32nd Pacific Asia Conference on Language, Information and Computation, 2018, pp. 531–540.