Menu
Publications
2024
2023
2022
2021
2020
2019
2018
2017
2016
2015
2014
2013
2012
2011
2010
2009
2008
2007
2006
2005
2004
2003
2002
2001
Editor-in-Chief
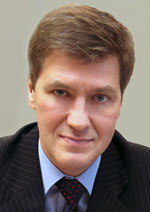
Nikiforov
Vladimir O.
D.Sc., Prof.
Partners
doi: 10.17586/2226-1494-2023-23-5-1030-1040
Confidence Lipschitz classifiers: an instrument of guaranteed reliability
Read the full article

Article in Russian
For citation:
Abstract
For citation:
Timofeev A.V. Confidence Lipschitz classifiers: an instrument of guaranteed reliability. Scientific and Technical Journal of Information Technologies, Mechanics and Optics, 2023, vol. 23, no. 5, pp. 1030–1040 (in Russian). doi: 10.17586/2226-1494-2023-23-5-1030-1040
Abstract
A new method of guaranteed solution for multiclass classification problem of stochastic objects is proposed. Within the framework of the proposed approach, the classification result is a finite set of class indices which with a predetermined confidence coefficient contains the index of the class to which the object being classified corresponds. In this case, the classification itself is realized on the basis of using a classifier of the new type which is called a confidence Lipschitz classifier. The definition of the confidence Lipschitz classifier is given and its main properties have been studied. Among them, the property of guaranteed reliability of the classification which is expressed in the construction of a confidence set of limited size containing the index of the true class with a predetermined coefficient of confidence, has been studied. The case of the assembly of Lipschitz classifiers, the properties of which are formalized in the form of a theorem, is considered. We consider a practically important example of using the proposed approach in the problems of compensation of the noise process dynamics in the channels of the fiber-optic monitoring system. The proposed approach is promising for use in those classification tasks in which the number of classes has an order higher than the second, including large-scale biometric identification systems as well as multi-channel systems for monitoring extended objects.
Keywords: confidence Lipschitz classifier, machine learning, guaranteed reliability, fiber optic monitoring system
References
References
- Korotaev V.V., Denisov V.M., Timofeev A.V. Analysis of seismoacoustic activity based on using optical fiber classifier. Proc. of the Latin America Optics and Photonics Conference, OSA, 2014, pp. LM4A.22. https://doi.org/10.1364/LAOP.2014.LM4A.22
- Aktas M., Akgun T., Demircin M.U., Buyukaydin D. Deep learning based multi-threat classification for phase-OTDR fiber optic distributed acoustic sensing applications. Proceedings of SPIE, 2017, vol. 10208, pp. 102080G. https://doi.org/10.1117/12.2262108
- Korotaev V.V., Denisov V.M., Rodrigues J.J.P.C., Serikova M.G., Timofeev A. Monitoring of deep-sea industrial facilities using fiber optic cable. Proceedings of SPIE, 2015, vol. 9525, pp. 95253Q. https://doi.org/10.1117/12.2184741
- Timofeev A.V., Denisov V.M. Multimodal heterogeneous monitoring of super-extended objects: modern view. Studies in Systems, Decision and Control, 2016, vol. 62, pp. 97–116. https://doi.org/10.1007/978-3-319-32525-5_6
- Timofeev A.V., Groznov D.I. Classification of seismoacoustic emission sources in fiber optic systems for monitoring extended objects. Optoelectronics, Instrumentation and Data Processing, 2020, vol. 56, no. 1, pp. 50–60. https://doi.org/10.3103/s8756699020010070
- Timofeev A.V. The guaranteed estimation of the Lipschitz classifier accuracy: Confidence set approach. Journal of the Korean Statistical Society, 2012, vol. 41, no. 1, pp. 105–114. https://doi.org/10.1016/j.jkss.2011.07.005
- Von Luxburg U., Bousquet O. Distance-based classification with Lipschitz functions. Journal of Machine Learning Research, 2009, vol. 5, pp. 669–695.
- Fazlyab M., Robey A., Hassani H., Morari M., Pappas G.J. Efficient and accurate estimation of Lipschitz constants for deep neural networks. NIPS'19: Proc. of the 33rd International Conference on Neural Information Processing Systems, 2019, pp. 11427–11438.
- Latorre F., Rolland P., Cevher V. Lipschitz constant estimation of Neural Networks via sparse polynomial optimization. Proc. of the 8th International Conference on Learning Representations (ICLR 2020).
- Vapnik V.N., Chervonenkis A.Ia. Pattern Recognition Theory: Statistical Problems of Learning. Moscow, Nauka Publ., 1974, 415 p. (in Russian)
- Advances in Large Margin Classifiers. Ed. by A. Smola, P. Barlett, B. Scholkopf, D. Schuurmans. MIT Press, 2000, 412 p.
- Hein M., Bousquet O., Schölkopf B. Maximal margin classification for metric spaces. Journal of Computer and System Sciences, 2005, vol. 71, no. 3, pp. 333–359. https://doi.org/10.1016/j.jcss.2004.10.013
- Bennett K.P., Bredensteiner E.J. Duality and geometry in SVM classifiers. Proc. of the International Conference on Machine Learning (ICML), 2000, pp. 57–64.
- Arens R., Eells J. On embedding uniform and topological spaces. Pacific Journal of Mathematics, 1956, vol. 6, no. 3, pp. 397–403. https://doi.org/10.2140/pjm.1956.6.397
- Hein M., Bousquet O. Maximal margin classification for metric space. Lecture Notes in Computer Science, 2003, vol. 2777, pp. 72–86. https://doi.org/10.1007/978-3-540-45167-9_7
- Breiman L. Bagging predictors. Machine Learning, 1996, vol. 24, no. 2, pp. 123–140. https://doi.org/10.1007/bf00058655
- Rangel P., Lozano F., García E. Boosting of support vector machines with application to editing. Proc. of the 4nd International Conference on Machine Learning and Applications (ICMLA’05), 2005. https://doi.org/10.1109/icmla.2005.13