Menu
Publications
2024
2023
2022
2021
2020
2019
2018
2017
2016
2015
2014
2013
2012
2011
2010
2009
2008
2007
2006
2005
2004
2003
2002
2001
Editor-in-Chief
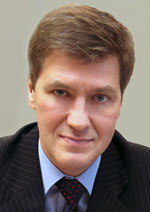
Nikiforov
Vladimir O.
D.Sc., Prof.
Partners
doi: 10.17586/2226-1494-2024-24-1-112-117
A method of storing vector data in compressed form using clustering
Read the full article

Article in Russian
For citation:
Abstract
For citation:
Tomilov N.A., Turov V.P., Babayants A.A., Platonov A.V. A method of storing vector data in compressed form using clustering. Scientific and Technical Journal of Information Technologies, Mechanics and Optics, 2024, vol. 24, no. 1, pp. 112–117 (in Russian). doi: 10.17586/2226-1494-2024-24-1-112-117
Abstract
The development of the machine learning algorithms for information search in recent years made it possible to represent text and multimodal documents in the form of vectors. These vector representations (embeddings) preserve the semantic content of documents and allow the search to be performed as the calculation of distance between vectors. Compressing embeddings can reduce the amount of memory they occupy and improve computational efficiency. The article discusses existing methods for compressing vector representations without loss of accuracy and with loss of accuracy. A method is proposed to reduce error by clustering vector representations using lossy compression. The essence of the method is in performing the preliminary clustering of vector representations, saving the centers of each cluster, and saving the coordinate value of each vector representation relative to the center of its cluster. Then, the centers of each cluster are compressed without loss of accuracy, and the resulting shifted vector representations are compressed with loss of accuracy. To restore the original vector representations, the coordinates of the center of the corresponding cluster are added to the coordinates of the displaced representation. The proposed method was tested on the fashion-mnist-784-euclidean and NYT-256-angular datasets. A comparison has been made of compressed vector representations with loss of accuracy by reducing the bit depth with vector representations compressed using the proposed method. With a slight (around 10 %) increase in the size of the compressed data, the absolute value of the error from loss of accuracy decreased by four and two times, respectively, for the tested sets. The developed method can be applied in tasks where it is necessary to store and process vector representations of multimodal documents, for example, in the development of search engines.
Keywords: vector representations, embeddings, clustering, k-means, compression of vector representations
References
References
- Grbovic M., Cheng H. Real-time personalization using embeddings for search ranking at airbnb. Proc. of the 24th ACM SIGKDD International Conference on Knowledge Discovery & Data Mining, 2018, pp. 311–320. https://doi.org/10.1145/3219819.3219885
- Berry M.W., Drmac Z., Jessup E.R. Matrices, vector spaces, and information retrieval. SIAM Review, 1999, vol. 41, no. 2, pp. 335–362. https://doi.org/10.1137/S0036144598347035
- Micikevicius P., Narang S., Alben J., Diamos G., Elsen E., Garcia D., Ginsburg B., Houston M., Kuchaiev O., Venkatesh G., Wu H. Mixed precision training. arXiv, 2017, arXiv:1710.03740. https://doi.org/10.48550/arXiv.1710.03740
- Zhang J., Yang J., Yuen H. Training with low-precision embedding tables. Systems for Machine Learning Workshop at NeurIPS, 2018, vol. 2018.
- Moghadasi M.N., Zhuang Y. Sent2Vec: A new sentence embedding representation with sentimental semantic. Proc. of the 2020 IEEE International Conference on Big Data (Big Data), 2020, pp. 4672–4680. https://doi.org/10.1109/BigData50022.2020.9378337
- Parekar P.M., Thakare S.S. Lossless data compression algorithm–a review. International Journal of Computer Science & Information Technologies, 2014, vol. 5, no. 1, pp. 276–278.
- Manro A., Sinha S., Chaturvedi B., Mohan J. Index seek versus table scan performance and implementation of RDBMS. Lecture Notes in Electrical Engineering, 2019, vol. 526, pp. 411–420. https://doi.org/10.1007/978-981-13-2553-3_40
- Kanungo T., Mount D., Netanyahu N., Piatko Ch., Silverman R., Wu A. The analysis of a simple k-means clustering algorithm. Proc. of the sixteenth annual symposium on Computational geometry, 2000, pp. 100–109. https://doi.org/10.1145/336154.336189
- Xiao H., Rasul K., Vollgraf R. Fashion-MNIST: a novel image dataset for benchmarking machine learning algorithms. arXiv, 2017, arXiv:1708.07747. https://doi.org/10.48550/arXiv.1708.07747
- Bi C., China P.R. Research and application of SQLite embedded database technology. WSEAS Transactions on Computers, 2009, vol. 8, no. 1, pp. 83–92.
- Turov V.P., Tomilov N.A., Babaiantc A.A. Development of a tool for comparing vector search algorithms. Proc. of the XI Congress of Young Scientists. V. 1. 2022, pp. 446–450. (in Russian)
- Aumüller M., Bernhardsson E., Faithfull A. ANN-benchmarks: A benchmarking tool for approximate nearest neighbor algorithms. Lecture Notes in Computer Science, 2017, vol. 10609, pp. 34–49. https://doi.org/10.1007/978-3-319-68474-1_3
- Golomb S. Run-length encodings (Corresp.). IEEE Transactions on Information Theory, 1966, vol. 12, no. 3, pp. 399–401. https://doi.org/10.1109/TIT.1966.1053907