Menu
Publications
2025
2024
2023
2022
2021
2020
2019
2018
2017
2016
2015
2014
2013
2012
2011
2010
2009
2008
2007
2006
2005
2004
2003
2002
2001
Editor-in-Chief
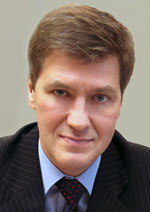
Nikiforov
Vladimir O.
D.Sc., Prof.
Partners
doi: 10.17586/2226-1494-2024-24-2-214-221
RuPersonaChat: a dialog corpus for personalizing conversational agents
Read the full article

Article in Russian
For citation:
Abstract
For citation:
Apanasovich K.S., Makhnytkina O.V., Kabarov V.I., Dalevskaya O.P. RuPersonaChat: a dialog corpus for personalizing conversational agents. Scientific and Technical Journal of Information Technologies, Mechanics and Optics, 2024, vol. 24, no. 2, pp. 214–221 (in Russian). doi: 10.17586/2226-1494-2024-24-2-214-221
Abstract
Personalization is one of the keyways to improve the performance of conversational agents. It improves the quality of user interaction with a conversational agent and increases user satisfaction by increasing the consistency and specificity of responses. The dialogue with the agent becomes more consistent, the inconsistency of responses is reduced, and the responses become more specific and interesting. Training and testing personalized conversational agents requires specific datasets containing facts about a persona and texts of persona’s dialogues where replicas use those facts. There are several datasets in English and Chinese containing an average of five facts about a persona where the dialogues are composed by crowdsourcing users who repeatedly imitate different personas. This paper proposes a methodology for collecting an original dataset containing an extended set of facts about a persona and natural dialogues between personas. The new RuPersonaChat dataset is based on three different recording scenarios: an interview, a short conversation, and a long conversation. This is the first dataset for dialogue agent personalization collected which includes both natural dialogues and extended persona’s descriptions. Additionally, in the dataset, the persona’s replicas are annotated with the facts about the persona from which they are generated. The methodology for collecting an original corpus of test data proposed in this paper allows for testing language models for various tasks within the framework of personalized dialogue agent development. The collected dataset includes 139 dialogues and 2608 replicas. This dataset was used to test answer and question generation models and the best results were obtained using the Gpt3-large model (perplexity is equal to 15.7). The dataset can be used to test the personalized dialogue agents’ ability to talk about themselves to the interlocutor, to communicate with the interlocutor utilizing phatic speech and taking into account the extended context when communicating with the user.
Keywords: data collection methodology, dialog data, conversational agents, personalization, question and answer generation
Acknowledgements. This study was funded by a grant from the Russian Science Foundation (22-11-00128, https://www.rscf.ru/ project/22-11-00128/).
References
Acknowledgements. This study was funded by a grant from the Russian Science Foundation (22-11-00128, https://www.rscf.ru/ project/22-11-00128/).
References
- Posokhov P., Apanasovich K., Matveeva A., Makhnytkina O., Matveev A. Personalizing dialogue agents for Russian: retrieve and refine. Proc. of the 31st Conference of Open Innovations Association (FRUCT), 2022, pp. 245–252. https://doi.org/10.23919/fruct54823.2022.9770895
- Matveev Y., Makhnytkina O., Posokhov P., Matveev A., Skrylnikov S. Personalizing hybrid-based dialogue agents. Mathematics, 2022, vol. 10, no. 24, pp. 4657. https://doi.org/10.3390/math10244657
- Zhang S., Dinan E., Urbanek J., Szlam A., Kiela D., Weston J. Personalizing Dialogue Agents: I have a dog, do you have pets too? Proc. of the 56th Annual Meeting of the Association for Computational Linguistics. V. 1, 2018, pp. 2204–2213. https://doi.org/10.18653/v1/p18-1205
- Dinan E., Logacheva V., Malykh V., Miller A., Shuster K., Urbanek J., Kiela D., Szlam A., Serban I., Lowe R., Prabhumoye S., Black A.W., Rudnicky A., Williams J., Pineau J., Burtsev M., Weston J. The second conversational intelligence challenge (ConvAI2). The NeurIPS'18 Competition. Springer, Cham, 2020, pp. 187–208. https://doi.org/10.1007/978-3-030-29135-8_7
- Rashkin H., Smith E.M., Li M., Boureau Y-L. Towards empathetic open-domain conversation models: A new benchmark and dataset. Proc. of the 57th Annual Meeting of the Association for Computational Linguistics, 2019, pp. 5370–5381. https://doi.org/10.18653/v1/p19-1534
- Smith E.M., Williamson M., Shuster K., Weston J., Boureau Y-L. Can you put it all together: evaluating conversational agents’ ability to blend skills. Proc. of the 58th Annual Meeting of the Association for Computational Linguistics, 2020, pp. 2021–2030. https://doi.org/10.18653/v1/2020.acl-main.183
- Xu J., Szlam A., Weston J. Beyond goldfish memory: Long-term open-domain conversation. Proc. of the 60th Annual Meeting of the Association for Computational Linguistics. V. 1, 2022, pp. 5180–5197. https://doi.org/10.18653/v1/2022.acl-long.356
- Xu X., Gou Z., Wu W., Niu Z-Y., Wu H., Wang H., Wang S. Long time no see! open-domain conversation with long-term persona memory. Findings of the Association for Computational Linguistics: ACL 2022, 2022, pp. 2639–2650. https://doi.org/10.18653/v1/2022.findings-acl.207
- Kuchaiev O., Li J., Nguyen H., Hrinchuk O., Leary R., Ginsburg B., Kriman S., Beliaev S., Lavrukhin V., Cook J., Castonguay P., Popova M., Huang J., Cohen J.M. NeMo: A toolkit for building ai applications using neural modules. arXiv, 2019. arXiv:1909.09577. https://doi.org/10.48550/arXiv.1909.09577
- Gulati A., Qin J., Chiu C.-C., Parmar N., Zhang Y., Yu J., Han W., Wang S., Zhang Z., Wu Y., Pang R. Conformer: Convolution-augmented transformer for speech recognition. Proc. of the Interspeech 2020, pp. 5036–5040. https://doi.org/10.21437/interspeech.2020-3015
- Vaswani A., Shazeer N., Parmar N., Uszkoreit J., Jones L., Gomez A.N., Kaiser Ł., Polosukhin I. Attention is all you need. Advances in Neural Information Processing Systems, 2017, vol. 30.
- Raffel C., Shazeer N., Roberts A., Lee K., Narang S., Matena M., Zhou Y., Li W., Liu P.J. Exploring the limits of transfer learning with a unified text-to-text transformer. Journal of Machine Learning Research, 2020, vol. 21, pp. 140.
- Brown T., Mann B., Ryder N., Subbiah M., Kaplan J.D., Dhariwal P., Neelakantan A., Shyam P., Sastry G., Askell A., Agarwal S., Herbert-Voss A., Krueger G., Henighan T., Child R., Ramesh A., Ziegler D., Wu J., Winter C., Hesse C., Chen M., Sigler E., Litwin M., Gray S., Chess B., Clark J., Berner C., McCandlish S., Radford A., Sutskever I., Amodei D. Language models are few-shot learners. Advances in Neural Information Processing Systems, 2020, vol. 33, pp. 1877–1901.
- Jelinek F., Mercer R.L., Bahl L.R., Baker J.K. Perplexity – a measure of the difficulty of speech recognition tasks. The Journal of the Acoustical Society of America, 1977, vol. 62, no. S1, pp. S63–S63. https://doi.org/10.1121/1.2016299
- Papineni K., Roukos S., Ward T., Zhu W.-J. BLEU: a method for automatic evaluation of machine translation. Proc. of the 40th Annual Meeting on Association for Computational Linguistics, 2002, pp. 311–318. https://doi.org/10.3115/1073083.1073135