Menu
Publications
2024
2023
2022
2021
2020
2019
2018
2017
2016
2015
2014
2013
2012
2011
2010
2009
2008
2007
2006
2005
2004
2003
2002
2001
Editor-in-Chief
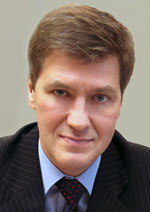
Nikiforov
Vladimir O.
D.Sc., Prof.
Partners
doi: 10.17586/2226-1494-2024-24-2-230-240
Guarantee structural anomaly detection in streaming data using the RRCF model: selection of detector parameters and its stabilization under additive noise conditions
Read the full article

Article in русский
For citation:
Abstract
For citation:
Timofeev A.V. Guarantee structural anomaly detection in streaming data using the RRCF model: selection of detector parameters and its stabilization under additive noise conditions. Scientific and Technical Journal of Information Technologies, Mechanics and Optics, 2024, vol. 24, no. 2, pp. 230–240 (in Russian). doi: 10.17586/2226-1494-2024-24-2-230-240
Abstract
A method for stabilizing structural anomaly detection under additive noise conditions as well as an algorithm for formal selection of the parameters of the solver rule in the structural anomaly detector based on the Robust Random Cut Forest (RRCF) method are proposed. In the framework of the developed approach, in order to stabilize the process of structural anomaly detection under the influence of additive noise, it is proposed to feed to the input of the RRCF-detector a data stream which is pre-processed by one of the digital filtering methods. In this case, the decision rule for anomaly detection is strictly formalized and transparently interpreted. The selection of parameters of the RRCF-based anomaly detector stabilized by pre-filtering methods of the input data stream is formalized. The RRCF-detector parameters choice within the proposed scheme guarantees a predetermined upper bound for the false alarm probability when deciding to detect a structural anomaly. This property is rigorously proved and formalized as a theorem. The performance of the stabilized RRCF-detector is investigated numerically. The achieved results confirm the performance of the proposed approach provided that the detection threshold is selected in the way proposed in this paper. An example of practical application of the proposed method is presented. The developed approach is promising for the detection of structural anomalies in conditions of observation additive noise, in a situation where it is important to guarantee an upper bound for the probability of false alarm. In particular, the approach can find application in monitoring technological regimes of liquid pumping in pipeline systems or in systems for detecting pre-failure states of technological equipment.
Keywords: Robust Random Cut Forest, structural anomaly detection, streaming data processing, guaranteed anomaly detection
References
References
- Gomes H.M., Read J., Bifet A. Streaming random patches for evolving data stream classification. Proc. of the IEEE International Conference on Data Mining (ICDM), 2019, pp. 240–249. https://doi.org/10.1109/ICDM.2019.00034
- Pang Z., Cen J., Yi M. Unsupervised concept drift detection method based on robust random cut forest. International Journal of Machine Learning and Cybernetics, 2023, vol. 14, no. 12, pp. 4207–4222. https://doi.org/10.1007/s13042-023-01890-x
- Zheng M., Geng L., Zuo B., Nakata T. A dynamic thresholds based anomaly detection algorithm in energy consumption process of industrial equipment. Proc. of the 2023 7th International Conference on Big Data and Internet of Things, 2023, pp. 201–209. https://doi.org/10.1145/3617695.3617706
- Marathe A. LRZ convolution: An algorithm for automatic anomaly detection in time-series data. Proc. of the 32nd International Conference on Scientific and Statistical Database Management, 2020, pp. 1–12. https://doi.org/10.1145/3400903.3400904
- Bohlke-Schneider M., Kapoor S., Januschowski T. Resilient neural forecasting systems. Proc. of the Fourth International Workshop on Data Management for End-to-End Machine Learning, 2022, pp. 1–5. https://doi.org/10.1145/3399579.3399869
- Timofeev A.V. Detection of randomly shaped signals under nonparametric a priori uncertainty about the distribution of observations. Izvestija vuzov. Radiojelektronika, 1991, no. 7, pp. 64–68. (in Russian)
- Timofeev A.V., Denisov V.M. Multimodal heterogeneous monitoring of super-extended objects: modern view. recent advances in systems safety and security. Studies in Systems, Decision and Control, 2016, vol. 62, pp. 97–116. https://doi.org/10.1007/978-3-319-32525-5_6
- Gomes H., Read J., Bifet A., Barddal J., Gama J. Machine learning for streaming data: state of the art, challenges, and opportunities. ACM SIGKDD Explorations Newsletter, 2019, vol. 21, no. 2, pp. 6–22. https://doi.org/10.1145/3373464.3373470
- Tatbul N., Lee T., Zdonik S., Alam M., Gottschlich J. Precision and recall for time series. Advances in Neural Information Processing Systems, 2018, vol. 31, pp. 1924–1934.
- Siddiqui M., Fern A., Dietterich T., Wright R., Theriault A., Archer D. Feedback-guided anomaly discovery via online optimization. Proc. of the 24th ACM SIGKDD International Conference on Knowledge Discovery & Data Mining, 2018, pp. 2200–2209. https://doi.org/10.1145/3219819.3220083
- Hariri S., Kind M. Batch and online anomaly detection for scientific applications in a Kubernetes environment. Proc. of the 9th Workshop on Scientific Cloud Computing, 2018, pp. 1–7. https://doi.org/10.1145/3217880.3217883
- Salehi M., Rashidi L. A survey on anomaly detection in evolving data. ACM SIGKDD Explorations Newsletter, 2018, vol. 20, no. 1, pp. 13–23. https://doi.org/10.1145/3229329.3229332
- Guha S., Mishra N., Roy G., Schrijvers O. Robust random cut forest based anomaly detection on streams. Proceedings of Machine Learning Research, 2016, vol. 46, pp. 2712–2721.
- Breiman L. Bagging predictors. Machine Learning, 1996, vol. 24, no. 2, pp. 123–140. https://doi.org/10.1007/bf00058655
- Putina A., Rossi D. Online anomaly detection leveraging stream-based clustering and real-time telemetry. IEEE Transactions on Network and Service Management, 2021, vol. 18, no. 1, pp. 839–854. https://doi.org/10.1109/TNSM.2020.3037019
- Vardhan H., Sztipanovits J. Reduced robust random cut forest for out-of-distribution detection in machine learning models. ArXiv, 2022, arXiv:2206.09247. https://doi.org/10.48550/arXiv.2206.09247
- Arce G.R. Nonlinear Signal Processing: A Statistical Approach. Wiley, 2005, 480 p.
- Savitzky A., Golay M.J.E. Smoothing and differentiation of data by simplified least squares procedures. Analytical Chemistry, 1964, vol. 36, no. 8, pp. 1627–1639. https://doi.org/10.1021/ac60214a047
- Timofeev A.V., Maksimov P.N., Groznov D.I. Application of fiber optic technology for monitoring the mine water drainage pipeline system in the permafrost zone. The Hydrotechnika, 2023, no. 3, pp. 34–43. (in Russian). https://doi.org/10.55326/22278400_2023_3_34