Menu
Publications
2024
2023
2022
2021
2020
2019
2018
2017
2016
2015
2014
2013
2012
2011
2010
2009
2008
2007
2006
2005
2004
2003
2002
2001
Editor-in-Chief
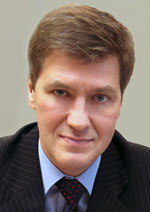
Nikiforov
Vladimir O.
D.Sc., Prof.
Partners
doi: 10.17586/2226-1494-2024-24-2-322-329
Censoring training samples using regularization of connectivity relations of class objects
Read the full article

Article in Russian
For citation:
Abstract
For citation:
Ignatev N.A., Tursunmurotov D.X. Censoring training samples using regularization of connectivity relations of class objects. Scientific and Technical Journal of Information Technologies, Mechanics and Optics, 2024, vol. 24, no. 2, pp. 322–329 (in Russian). doi: 10.17586/2226-1494-2024-24-2-322-329
Abstract
The censoring of training datasets is considered taking into account the specific implementation of the nearest neighbor method algorithms. The censoring process is associated with the use of a set of boundary objects of classes according to a given metric for the purpose of: searching and removing noise objects and analyzing the cluster structure of the training sample in relation to connectivity. Special conditions for removing noise objects and forming a precedent base for training algorithms are explored. Recognition of objects using such a database should provide higher accuracy with minimal computational resources relative to the original dataset. Necessary and sufficient conditions for selecting noise objects from a set of boundary ones have been developed. The necessary condition for a boundary object to belong to the noise set is specified in the form of a restriction (threshold) on the ratio of the distances to the nearest object from its class and its complement. The search for the minimum coverage of the training dataset with standards is carried out based on the analysis of the cluster structure. The standards are represented by sample objects. The structure of the connectivity relations of objects according to the hypersphere system is used to group them. The composition of the groups is formed from centers (dataset objects) for hyperspheres the intersection of which contains boundary objects. The value of the compactness measure is calculated as the average number of objects in the training dataset, excluding noise, pulled in by one standard of minimum coverage. An analysis is carried out of the connection between the generalizing ability of algorithms in machine learning and the value of the compactness measure. The presence of a connection is justified by a criterion (regularizer) for selecting the number and composition of a set of noise objects. Optimal regularization coefficients are defined as threshold values for removing noise objects. The relationship between the value of the training dataset compactness measure and the generalizing ability of recognition algorithms is shown. The connection was identified using the standards of minimum sample coverage from which the precedent base was formed. It was found that the recognition accuracy using the precedent base is higher than that using the original dataset. The minimum composition of the precedent base includes descriptions of standards and parameters of local metrics. When using data normalization procedures, additional parameters are required. Analysis of the values of the compactness measure is in demand to detect overfitting of algorithms associated with the dimension of the feature space. Recognition based on precedents minimizes the cost of computing resources using nearest neighbor algorithms. Recommendations are given for the development of models in the field of information security for processing and interpreting sociological research data. For use in information security, a precedent base is being formed to identify DDOS attacks. It is proposed to obtain new knowledge from the field of sociology through the analysis of the values of indicators of noise objects and the interpretation of the results of dividing respondents into non-overlapping groups in relation to the connectedness of objects. The configurations of groups in relation to connectivity are not initially known. There is no point in calculating their centers which can be located outside the configurations. To explain the contents of groups, it is proposed to use standards of minimum coverage.
Keywords: compactness measures, precedent base, regularization coefficients, minimum coverage with standards, noise objects
Acknowledgements. The work was carried out within the framework of the scientific research plan of the Department of Artificial Intelligence of the National University of Uzbekistan.
References
Acknowledgements. The work was carried out within the framework of the scientific research plan of the Department of Artificial Intelligence of the National University of Uzbekistan.
References
- Borisova I.A., Kutnenko O.A. Outliers detection in datasets with misclassified objects. Machine Learning and Data Analysis, 2015, vol. 1, no. 11, pp. 1632–1641. (in Russian)
- Zagoruiko N.G., Kutnenko O.A. Training dataset censoring. Tomsk State University Journal of Control and Computer Science, 2013, no. 1(22), pp. 66–73. (in Russian)
- Kutnenko O.A., Plyasunov A.V. NP-hardness of some data cleaning problem. Journal of Applied and Industrial Mathematics, 2021, vol. 15, no. 2, pp. 285–291. https://doi.org/10.1134/S1990478921020095
- Borisova I.A., Kutnenko O.A. The problem of correction diagnostic errors in the target attribute with the function of rival similarity. Mathematical Biology and Bioinformatics, 2018, vol. 13, no. 1, pp. 38–49. (in Russian). https://doi.org/10.17537/2018.13.38
- Ignatyev N.A. Structure choice for relations between objects in metric classification algorithms. Pattern Recognition and Image Analysis, 2018, vol. 28, no. 4, pp. 695–702. https://doi.org/10.1134/s1054661818040132
- Rudakov K.V. On some factorizations of semi-metric cones and quality estimates of heuristic metrics in data analysis problems. Doklady Mathematics, 2020, vol. 101, no. 3, pp. 257–258. https://doi.org/10.1134/S1064562420030230
- Zukhba A.V. Computational complexity estimation of the problems of selecting reference objects and features. Dissertation for the degree of candidate of physical and mathematical sciences. Moscow, 2018, 113 p. (in Russian)
- Ignatev N.A., Rahimova M.A. Formation and analysis of sets of informative features of objects by pairs of classes. Scientific and Technical Information Processing, 2022, vol. 49, no. 6, pp. 439–445. https://doi.org/10.3103/S0147688222060053