Menu
Publications
2025
2024
2023
2022
2021
2020
2019
2018
2017
2016
2015
2014
2013
2012
2011
2010
2009
2008
2007
2006
2005
2004
2003
2002
2001
Editor-in-Chief
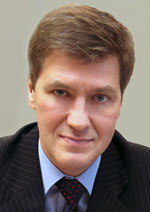
Nikiforov
Vladimir O.
D.Sc., Prof.
Partners
doi: 10.17586/2226-1494-2024-24-2-335-338
Modeling perceiving of recommendations provided by clinical decision support system based on predictive modeling within dental preventive screening
Read the full article

Article in Russian
For citation:
Abstract
For citation:
Soldatov A.N., Soldatov I.K., Kovalchuk S.V. Modeling perceiving of recommendations provided by clinical decision support system based on predictive modeling within dental preventive screening. Scientific and Technical Journal of Information Technologies, Mechanics and Optics, 2024, vol. 24, no. 2, pp. 335–338 (in Russian). doi: 10.17586/2226-1494-2024-24-2-335-338
Abstract
The results of a current study of the perception of clinical decision support systems (CDSS) in the framework of preventive screening by dentists in schools of the Russian Ministry of Defense (cadet corps) are presented. Using the example of the scenario under consideration, a prototype of the CDSS based on machine learning was evaluated. To assess perception, a survey was conducted demonstrating the results of the prototype and assessing the perceived characteristics of the provided predictive modeling results. A model was built based on a Bayesian network to evaluate the considered indicators, which demonstrated an increase in the quality of prediction of perceived indicators, taking into account the influence of latent states of the operator’s subjective perception. The proposed approach is planned to be used in the future to increase the efficiency of doctor-CDSS interaction.
Keywords: decision support systems, human-computer interaction, predictive modeling, machine learning, caries, preventive screening
References
References
- Kuzmina E.M., Yanushevich O.O., Kuzmina I.N., Lapatina A.V. Tendency in the prevalence of dental caries among the Russian population over a 20-year period. Dental Forum, 2020, no. 3(78), pp. 2–8. (in Russian)
- Voloshina I.M., Belikova E.V. High risk dental caries and patient compliance. Endodontics Today, 2020, vol. 18, no. 2, pp. 41–44. (in Russian). https://doi.org/10.36377/1683-2981-2020-18-2-41-44
- Soldatov A.N. et al. Predicting the intensity of caries damage to the teeth of students in educational institutions of the Russian Ministry of Defense using machine learning algorithms. Kremlin Medicine Journal, 2024, in press. (in Russian)
- Kovalchuk S.V., Kopanitsa G.D., Derevitskii I.V., Matveev G.A., Savitskaya D.A. Three-stage intelligent support of clinical decision making for higher trust, validity, and explainability. Journal of Biomedical Informatics, 2022, vol. 27, pp. 104013. https://doi.org/10.1016/j.jbi.2022.104013
- Abdul A., Vermeulen J., Wang D., Lim B.Y., Kankanhalli M. Trends and trajectories for explainable, accountable and intelligible systems: an HCI research agenda. Proc. of the 2018 CHI Conference on Human Factors in Computing Systems, 2018, pp. 1–18. https://doi.org/10.1145/3173574.3174156
- Soldatov A.N., Soldatov I.K., Grebnev G.A., Kovalchuk S.V. Database of the dental health state of students in educational institutions of the Russian Ministry of Defense. Database registration certificate RU2023622392, 13.07.2023. (in Russian)
- Presseau J., Johnston M., Francis J.J., Hrisos S., Stamp E., Steen N., Hawthorne G., Grimshaw J.M., Elovainio M., Hunter M., Eccles M.P. Theory-based predictors of multiple clinician behaviors in the management of diabetes. Journal of Behavioral Medicine, 2014, vol. 37, no. 4, pp. 607–620. https://doi.org/10.1007/s10865-013-9513-x
- Dillon A. Human Acceptance of Information Technology. International Encyclopedia of Ergonomics and Human Factors, 2006, vol. 27, no. 3, pp. 425–478.