Menu
Publications
2025
2024
2023
2022
2021
2020
2019
2018
2017
2016
2015
2014
2013
2012
2011
2010
2009
2008
2007
2006
2005
2004
2003
2002
2001
Editor-in-Chief
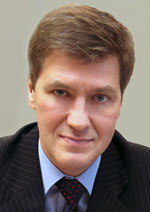
Nikiforov
Vladimir O.
D.Sc., Prof.
Partners
doi: 10.17586/2226-1494-2024-24-3-464-473
Elimination of distortions of weak images of astronomical objects on the example of Saturn, Jupiter and their satellites
Read the full article

Article in Russian
For citation:
Abstract
For citation:
Sizikov V.S., Rushchenko N.G. Elimination of distortions of weak images of astronomical objects on the example of Saturn, Jupiter and their satellites. Scientific and Technical Journal of Information Technologies, Mechanics and Optics, 2024, vol. 24, no. 3, pp. 464–473 (in Russian). doi: 10.17586/2226-1494-2024-24-3-464-473
Abstract
Methods and algorithms for restoring smeared and noisy images by numerically solving integral equations (IE) are considered. The algorithms are illustrated by the restoration of distorted images of celestial bodies using the example of images of Saturn, Jupiter and their satellites against the background of the starry sky. Images of objects may be weak, which will require increased exposure and may lead to a mismatch between the rotations of the Earth and a telescope, and then a smear image of the object will occur. The article proposes to eliminate smear by mathematical and computer processing of the distorted image. In this case, the type and the parameters of a smear may be practically unknown or known inaccurately. The novelty of the proposed solution lies in the fact that the type and the parameters of a distortion, and therefore the kernel of an IE or the point spread function (PSF), are determined by the original “spectral method”. In the direct problem, modeling the smear and noise in receivers (telescopes) is performed by calculating convolutiontype integrals. In the inverse problem, image smearing is performed by IE solving with the Wiener parametric filtering method using the new “spectral method” for determining the kernel of the IE as well as filtering the noise by the Tukey median filter and the new modified filter. Error estimates for each operation are obtained. A technique has been proposed that makes it possible to eliminate, through the use of mathematical and software tools, images of planets, natural and instrumental noise, image smear, and also to obtain the clear images of Saturn, Jupiter and their satellites. Undistorted images of Saturn and Jupiter with their satellites were taken from astronomical catalogs. By modeling, we have obtained a distorted (smeared and noisy) image of Saturn with given distortion parameters (smear angle θ and smear value Δ) and a truly distorted image of Jupiter with unknown distortion parameters determined by the spectral method. Next, the image of Saturn with its satellites was restored by solving the IE. Image processing of Jupiter was also carried out, in which, to eliminate image smear by solving the integral equation, the “spectral method” was used to determine the smear parameters, and therefore the PSF and the kernel of the integral equation. The performance of the proposed method is determined both by visual assessment of the reconstructed image and by calculating the reconstruction error. The proposed technique makes it possible to eliminate in images of various space objects, in particular, Saturn and Jupiter, the natural or instrumental noise, as well as image smear, and to highlight faint objects (satellites, etc.) against the background of stars.
Keywords: image smearing and noising, determination of image distortion parameters, smear removal, noise filtering
Acknowledgements. The work was supported by a grant from MFKTU ITMO, project No. 620164 (Artificial intelligence methods for cyberphysical systems).
References
Acknowledgements. The work was supported by a grant from MFKTU ITMO, project No. 620164 (Artificial intelligence methods for cyberphysical systems).
References
- Egoshkin N.A., Eremeev V.V. Correction of the blurred images in the Earth space observation systems. Digital Signal Processing. 2010, no. 4, pp. 28–32. (in Russian)
- Yagola A.G., Koshev N.A. Restoration of smeared and defocused color images. Numerical Methods and Programming, 2008, vol. 9, pp. 207–212. (in Russian)
- Tikhonov A.N., Goncharskii A.V., Stepanov V.V., Iagola A.G. Numerical methods for solving ill-defined problems. Moscow, Nauka Publ., 1990, 232 p. (in Russian)
- Gruzman I.S., Kirichuk V.S., Kosykh V.P., Peretiagin G.I., Spektor A.A. Digital image processing in information systems. Novosibirsk, NSTU Publ., 2002, 352 p. (in Russian)
- Sizikov V.S. Spectral method for estimating the point-spread function in the task of eliminating image distortions. Journal of Optical Technology, 2017, vol. 84, no. 2, pp. 95–101. https://doi.org/10.1364/JOT.84.000095
- Sizikov V.S., Sergienko A.A., Kondulukova D.A. Spectral method for stable estimating the distortion parameters in inverse problem of image restoration. Journal of Instrument Engineering, 2019, vol. 62, no. 4, pp. 379–386. (in Russian). https://doi.org/10.17586/0021-3454-2019-62-4-379-386
- Sizikov V.S. Direct and inverse problems of image reconstruction, spectroscopy and tomography when using MatLab. St. Petersburg, Lan’ Publ., 2017, 412 p. (in Russian).
- Narayanan S.A., Arumugam G., Bijlani K. Trimmed median filters for salt and pepper noise removal. International Journal of Emerging Trends & Technology in Computer Science. (IJETTCS), 2013, vol. 2, no. 1, pp. 35–40.
- Petrov Yu.P., Sizikov V.S. Well-Posed, Ill-Posed, and Intermediate Problems with Applications. Berlin, Walter de Gruyter, 2005, 234 p. https://doi.org/10.1515/9783110195309
- D’yakonov V., Abramenkova I. MATLAB: Signal and Image Processing. St. Petersburg, Piter Publ., 2002, 608 p. (in Russian).
- Tikhonov A.N., Goncharsky A.V., Stepanov V.V. Inverse problems of photoprocessing. Ill-defined problems of the natural science. Ed by A.N. Tikhonov, A.V. Goncharsky. Moscow, Moscow State University, 1987, pp. 185–195. (in Russian).
- Lim J.S. Two-dimensional signal and image processing. New Jersey, Prentice Hall PTR, 1990, 694 p.
- Gonzalez R.C., Woods R.E. Digital Image Processing. Saddle River, NJ, USA, Prentice Hall, 2002, 793 p.
- Gonsales R.C., Woods R.E., Eddins S.L Digital Image Processing Using MATLAB. Saddle River, NJ, USA, Prentice Hall, 2004, 609 p.
- Donatelli M., Estatico C., Martinelli A., Serra-Capizzano S. Improved image deblurring with anti-reflective boundary conditions and re-blurring. Inverse Problems, 2006, vol. 22, pp. 2035–2053. https://doi.org/10.1088/0266-5611/22/6/008
- Arefyeva M.V., Sysoev A.F. Fast regularization algorithms for digital image reconstruction. Vychisl. Methods Program., 1983, vol. 39, pp. 40–55. (in Russian).
- Mesarović V.Z., Galatsanos N.P., Katsaggelos A.K. Regularized constrained total least squares image restoration. IEEE Transactions on Image Processing, 1995, vol. 4. no. 8, pp. 1096–1108. https://doi.org/10.1109/83.403444
- Christiansen M., Hanke M. Deblurring methods using antireflective boundary conditions. SIAM Journal on Scientific Computing, 2008, vol. 30, no. 2. https://doi.org/10.1137/060671413
- Zhuravel I.M. Short Course in Image Processing Theory, 2008, Available at: https://hub.exponenta.ru/post/kratkiy-kurs-teorii-obrabotki-izobrazheniy734 (accessed: 31.01.2024). (in Russian)
- Hansen P.C. Discrete inverse problems: Insight and algorithms. Philadelphia, SIAM, 2010, 213 p. https://doi.org/10.1137/1.9780898718836
- Russ J.C. The Image Processing Handbook. 6th ed. Boca Raton, CRC Press, 2011, 853 p.
- Engl H.W., Hanke M., Neubauer A. Regularization of inverse problems. Dordrecht, Kluwer, 1996, 328 p. https://doi.org/10.1007/978-94-009-1740-8
- Voskoboinikov Y.E. A combined nonlinear contrast image reconstruction algorithm under inexact point-spread function. Optoelectronics, Instrumentation and Data Processing, 2007, vol. 43, no. 6, pp. 489–499. https://doi.org/10.3103/S8756699007060015
- Fergus R., Singh B., Hertzmann A., Roweis S.T., Freeman W.T. Removing camera shake from a single photograph. ACM Transactions on Graphics, 2006, vol. 25, no. 3, pp. 787–794. https://doi.org/10.1145/1179352.1141956
- Yushikov V.S. Blind Deconvolution – Automatic Restoration of the Blurred Images. Available at: https://habr.com/ru/post/175717/ (accessed: 31.01.2024). (in Russian)
- Cho S., Lee S. Fast motion deblurring. ACM Transactions on Graphics, 2009, vol. 28, no. 5, pp. 145. https://doi.org/10.1145/1618452.1618491
- Jähne B. Digital Image Processing. Berlin, Springer, 2005, 584 p. https://doi.org/10.1007/3-540-27563-0
- Ostrikov V.N., Plakhotnikov O.V. Identifying the point-spread function of an observation channel from a calibrating image by the method of least squares. Journal of Optical Technology, 2006, vol. 73, no. 2, pp. 91–95. https://doi.org/10.1364/JOT.73.000091
- Sizikov V.S., Stepanov A.V., Mezhenin A.V., Burlov D.I., Éksemplyarov R.A. Determining image-distortion parameters by spectral means when processing pictures of the earth’s surface obtained from satellites and aircraft. Journal of Optical Technology, 2018, vol. 85, no. 4, pp. 203–210. https://doi.org/10.1364/JOT.85.000203
- Sizikov V., Rushchenko N. “Spectral method” for determining a kernel of the Fredholm integral equation of the first kind of convolution type and suppressing the Gibbs effect. Mathematics, 2024, vol. 12, no. 1, pp. 13. https://doi.org/10.3390/math12010013