Menu
Publications
2025
2024
2023
2022
2021
2020
2019
2018
2017
2016
2015
2014
2013
2012
2011
2010
2009
2008
2007
2006
2005
2004
2003
2002
2001
Editor-in-Chief
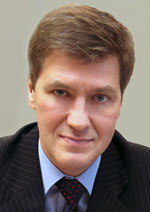
Nikiforov
Vladimir O.
D.Sc., Prof.
Partners
doi: 10.17586/2226-1494-2024-24-3-474-482
Method for generating information sequence segments using the quality functional of processing models
Read the full article

Article in Russian
For citation:
Abstract
For citation:
Tikhonov D.D., Lebedev I.S. Method for generating information sequence segments using the quality functional of processing models. Scientific and Technical Journal of Information Technologies, Mechanics and Optics, 2024, vol. 24, no. 3, pp. 474–482 (in Russian). doi: 10.17586/2226-1494-2024-24-3-474-482
Abstract
The constantly emerging need to increase the efficiency of solving classification problems and predicting the behavior of objects under observation necessitates improving data processing methods. This article proposes a method for improving the quality indicators of machine learning models in regression and forecasting problems. The proposed processing of information sequences involves the use of input data segmentation. As a result of data division, segments with different properties of observation objects are formed. The novelty of the method lies in dividing the sequence into segments using the quality functional of processing models on data subsamples. This allows you to apply the best quality models on various data segments. The segments obtained in this way are separate subsamples to which the best quality models and machine learning algorithms are assigned. To assess the quality of the proposed solution, an experiment was performed using model data and multiple regression. The obtained values of the quality indicator RMSE for various algorithms on an experimental sample and with a different number of segments demonstrated an increase in the quality indicators of individual algorithms with an increase in the number of segments. The proposed method can improve RMSE performance by an average of 7 % by segmenting and assigning models that have the best performance in individual segments. The results obtained can be additionally used in the development of models and data processing methods. The proposed solution is aimed at further improving and expanding ensemble methods. The formation of multi-level model structures that process, analyze incoming information flows and assign the most suitable model for solving the current problem makes it possible to reduce the complexity and resource intensity of classical ensemble methods. The impact of the overfitting problem is reduced, the dependence of processing results on the basic models is reduced, the efficiency of setting up basic algorithms in the event of transformation of data properties is increased, and the interpretability of the results is improved.
Keywords: information sequence of data, multi-level data processing model, data segmentation, improving quality indicators
References
References
- Marques H.O., Swersky L., Sander J., Campello R., Zimek A. On the evaluation of outlier detection and one-class classification: a comparative study of algorithms, model selection, and ensembles. Data Mining and Knowledge Discovery, 2023, vol. 37, no. 4, pp. 1473–1517. https://doi.org/10.1007/s10618-023-00931-x
- Mishra S., Shaw K., Mishra D., Patil S., Kotecha K., Kumar S., Bajaj S. Improving the accuracy of ensemble machine learning classification models using a novel bit-fusion algorithm for healthcare AI systems. Frontiers in Public Health, 2022, vol. 10, pp. 1–17. https://doi.org/10.3389/fpubh.2022.858282
- Ren J., Tapert S., Fan C.C., Thompson W.K. A semi-parametric Bayesian model for semi-continuous longitudinal data. Statistics in Medicine, 2022, vol. 41, no. 13, pp. 2354–2374. https://doi.org/10.1002/sim.9359
- Zhang Y., Liu J., Shen W. A review of ensemble learning algorithms used in remote sensing applications. Applied Sciences, 2022, vol. 12, no. 17, pp. 8654. https://doi.org/10.3390/app12178654
- Bellman R. On the approximation of curves by line segments using dynamic programming. Communications of the ACM, 1961, vol. 4, no. 6, pp. 284–301. https://doi.org/10.1145/366573.366611
- Page E. A test for a change in a parameter occurring at an unknown point. Biometrika, 1955, vol. 42, no. 3/4, pp. 523–527. https://doi.org/10.2307/2333401
- Fisher W.D. On grouping for maximum homogeneity. Journal of the American Statistical Association, 1958, vol. 53, no. 284, pp. 789–798. https://doi.org/10.1080/01621459.1958.10501479
- Melnyk I., Banerjee A. A spectral algorithm for inference in hidden semi-Markov models. Journal of Machine Learning Research, 2017, vol. 18, no. 35, pp. 1–39.
- Bardwell L., Fearnhead P. Bayesian detection of abnormal segments in multiple time series. Bayesian Analysis, 2017, vol. 12, no. 1, pp. 193–218. https://doi.org/10.1214/16-ba998
- Chung F.-L., Fu T.-C., Ng V., Luk R.W.P. An evolutionary approach to pattern-based time series segmentation. IEEE Transactions on Evolutionary Computation, 2004, vol. 8, no. 5, pp. 471–489. https://doi.org/10.1109/tevc.2004.832863
- Levchenko O., Kolev B., Yagoubi D.E., Akbarinia R., Masseglia F., Palpanas T., Shasha D., Valduriez P. BestNeighbor: efficient evaluation of kNN queries on large time series databases. Knowledge and Information Systems, 2020, vol. 63, no. 2, pp. 349–378. https://doi.org/10.1007/s10115-020-01518-4
- Nikolaou A., Gutiérrez P.A., Durán A., Dicaire I., Fernández-Navarro F., Hervás-Martínez C. Detection of early warning signals in paleoclimate data using a genetic time series segmentation algorithm. Climate Dynamics, 2015, vol. 44, no. 7, pp. 1919–1933. https://doi.org/10.1007/s00382-014-2405-0
- Liu N., Zhao J. Streaming data classification based on hierarchical concept drift and online ensemble. IEEE Access, 2023, vol. 11, pp. 126040–126051. https://doi.org/10.1109/access.2023.3327637
- Zhong G., Shu T., Huang G., Yan X. Multi-view spectral clustering by simultaneous consensus graph learning and discretization. Knowledge-Based Systems, 2022, vol. 235, pp. 107632. https://doi.org/10.1016/j.knosys.2021.107632
- Liakos P., Papakonstantinopoulou K., Kotidis Y. Chimp: efficient lossless floating point compression for time series databases. Proceedings of the VLDB Endowment, 2022, vol. 15, no. 11, pp. 3058–3070. https://doi.org/10.14778/3551793.3551852
- Lebedev I. Dataset segmentation considering the information about impact factors. Information and Control Systems, 2021, no. 3(112), pp. 29–38. (in Russian). https://doi.org/10.31799/1684-8853-2021-3-29-38
- Maltsev G.N., Yakimov V.L. Approach to the generalized parameters formation of the complex technical systems technical condition using neural network structures. Scientific and Technical Journal of Information Technologies, Mechanics and Optics, 2023, vol. 23, no. 4, pp. 828–835. (in Russian). https://doi.org/10.17586/2226-1494-2023-23-4-828-835
- Shili H. Clustering in big data analytics: a systematic review and comparative analysis (review article). Scientific and Technical Journal of Information Technologies, Mechanics and Optics, 2023, vol. 23, no. 5, pp. 967–979. https://doi.org/10.17586/2226-1494-2023-23-5-967-979
- Lebedev I.S., Sukhoparov M.E. Adaptive Learning and integrated use of information flow forecasting methods. Emerging Science Journal, 2023, vol. 7, no. 3, pp. 704–723. https://doi.org/10.28991/esj-2023-07-03-03
- Tong W., Wang Y., Liu D. An adaptive clustering algorithm based on local-density peaks for imbalanced data without parameters. IEEE Transactions on Knowledge and Data Engineering, 2023, vol. 35, no. 4, pp. 3419–3432. https://doi.org/10.1109/tkde.2021.3138962
- Silva R.P., Zarpelão B.B., Cano A., Junior S.B. Time series segmentation based on stationarity analysis to improve new samples prediction. Sensors, 2021, vol. 21, no. 21, pp. 7333. https://doi.org/10.3390/s21217333
- Barzegar V., Laflamme S., Hu C., Dodson J. Multi-time resolution ensemble LSTMs for enhanced feature extraction in high-rate time series. Sensors, 2021, vol. 21, no. 6, pp. 1954. https://doi.org/10.3390/s21061954
- Huang W., Ding N. Privacy-preserving support vector machines with flexible deployment and error correction. Lecture Notes in Computer Science, 2021, vol. 13107, pp. 242–262. https://doi.org/10.1007/978-3-030-93206-0_15
- Zhang X., Wang M. Weighted random forest algorithm based on Bayesian algorithm. Journal of Physics: Conference Series, 2021, vol. 1924, pp. 012006. https://doi.org/10.1088/1742-6596/1924/1/012006
- Di Franco G., Santurro M. Machine learning, artificial neural networks and social research. Quality & Quantity, 2021, vol. 55, no. 3, pp. 1007–1025. https://doi.org/10.1007/s11135-020-01037-y
- Si S., Zhao J., Cai Z., Dui H. Recent advances in system reliability optimization driven by importance measures. Frontiers of Engineering Management, 2020, vol. 7, no. 3, pp. 335–358. https://doi.org/10.1007/s42524-020-0112-6
- Xu S., Song Y., Hao X. A comparative study of shallow machine learning models and deep learning models for landslide susceptibility assessment based on imbalanced data. Forests, 2022, vol. 13, no. 11, pp. 1908. https://doi.org/10.3390/f13111908