Menu
Publications
2024
2023
2022
2021
2020
2019
2018
2017
2016
2015
2014
2013
2012
2011
2010
2009
2008
2007
2006
2005
2004
2003
2002
2001
Editor-in-Chief
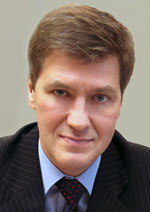
Nikiforov
Vladimir O.
D.Sc., Prof.
Partners
doi: 10.17586/2226-1494-2024-24-3-483-489
Smartphone video motion deblur order model
Read the full article

Article in English
For citation:
Abstract
For citation:
Sallama R.A. Smartphone video motion deblur order model. Scientific and Technical Journal of Information Technologies, Mechanics and Optics, 2024, vol. 24, no. 3, pp. 483–489. doi: 10.17586/2226-1494-2024-24-3-483-489
Abstract
A method has been proposed to eliminate slight motion blur in the image. The method is implemented in three stages. Blur estimation is achieved by prior information on the distribution image gradient. The Gaussian Orientation Filter (GOF) fits the prior information to find the regression coefficients. Order combines different estimate GOF parameters to generate a removal blur filter. Estimation parameters are fixed and set blur on the image to produce an image without boosting the noise and unwanted. The proposed model optimization solves the problem by minimizing the loss function. The suggested method applies to outdoor and indoor video acquired by modern smartphones. The experiment result display is accurate for the full regression motion blur model. The suggested model example on video dataset conditions has 23 s video time long and 228 MP dataset size. Measurement evaluation established on time consumer, Structural Similarity Index Measure and Peak Signal-to-Noise Ratio. Experimental results show that the image artifact phase is less consuming computational time. The proposed model has a minimized cost function and generates image quality.
Keywords: smartphone platform, motion blur, gaussian orientation, blur filter, loss function
References
References
- Baptiste M., Behrang M., Cédric M. A shock filter for image deblurring and enhancement with oriented hourglass tensor. Proc. of the 11th International Symposium on Image and Signal Processing and Analysis (ISPA), 2019, pp. 111–116. https://doi.org/10.1109/ispa.2019.8868552
- Lai W.-S., Huang J.-B., Hu Z., Ahuja N., Yang M.-H. A comparative study for single image blind deblurring. Proc. of the IEEE Conference on Computer Vision and Pattern Recognition (CVPR), 2016, pp. 1701–1709. https://doi.org/10.1109/cvpr.2016.188
- Zhang K., Luo W., Zhong Y., Ma L., Stenger B., Liu W., Li H. Deblurring by realistic blurring. Proc. of the IEEE/CVF Conference on Computer Vision and Pattern Recognition (CVPR), 2020, pp. 2734–2743. https://doi.org/10.1109/cvpr42600.2020.00281
- Wieschollek P., Hirsch M., Scholkopf B., Lensch H. Learning blind motion deblurring. Proc. of the IEEE International Conference on Computer Vision (ICCV), 2017, pp. 231–240. https://doi.org/10.1109/iccv.2017.34
- Pan J., Sun D., Pfister H., Yang M.-H. Deblurring images via dark channel prior. IEEE Transactions on Pattern Analysis and Machine Intelligence, 2018, vol. 40, no. 10, pp. 2315–2328. https://doi.org/10.1109/tpami.2017.2753804
- Chen L., Fang F., Wang T., Zhang G. Blind image deblurring with local maximum gradient prior. Proc. of the IEEE/CVF Conference on Computer Vision and Pattern Recognition (CVPR), 2019, pp. 1742–1750. https://doi.org/10.1109/cvpr.2019.00184
- Guo Q., Feng W., Gao R., Liu Y., Wang S. Exploring the effects of blur and deblurring to visual object tracking. IEEE Transactions on Image Processing, 2021, vol. 30, pp. 1812–1824. https://doi.org/10.1109/tip.2020.3045630
- Whang J., Delbracio M., Talebi H., Saharia C., Dimakis A.G., Milanfar P. Deblurring via stochastic refinement. Proc. of the IEEE/CVF Conference on Computer Vision and Pattern Recognition (CVPR), 2022, pp. 16272–16282. https://doi.org/10.1109/cvpr52688.2022.01581
- Carbajal G., Vitoria P., Lezama J., Musé P. Blind motion deblurring with pixel-wise kernel estimation via kernel prediction networks. IEEE Transactions on Computational Imaging, 2023, vol. 9, pp. 928–943. https://doi.org/10.1109/tci.2023.3322012
- Zhang R., Isola P., Efros A.A., Shechtman E., Wang O. The unreasonable effectiveness of deep features as a perceptual metric. Proc. of the IEEE/CVF Conference on Computer Vision and Pattern Recognition, 2018, pp. 586–595. https://doi.org/10.1109/cvpr.2018.00068
- Niklaus S., Mai L., Liu F. Video frame interpolation via adaptive separable convolution. Proc. of the IEEE International Conference on Computer Vision (ICCV), 2017, pp. 261–270. https://doi.org/10.1109/iccv.2017.37
- Ge X., Liu J., Hu D., Tan J. An extended sparse model for blind image deblurring. Signal, Image and Video Processing, 2024, vol. 18, no. 2, pp. 1863–1877. https://doi.org/10.1007/s11760-023-02888-2
- Fergus R., Singh B., Hertzmann A., Roweis S.T., Freeman W.T. Removing camera shake from a single photograph. ACM Transactions on Graphics, 2006, vol. 25, no. 3, pp. 787–794. https://doi.org/10.1145/1141911.1141956
- Delbraico M., Garcia-Dorado I., Choi S., Kelly D., Milanfar P. Polyblur: Removing mild blur by polynomial reblurring. IEEE Transactions on Computational Imaging, 2021, vol. 7, pp. 837–848. https://doi.org/10.1109/tci.2021.3100998