Menu
Publications
2025
2024
2023
2022
2021
2020
2019
2018
2017
2016
2015
2014
2013
2012
2011
2010
2009
2008
2007
2006
2005
2004
2003
2002
2001
Editor-in-Chief
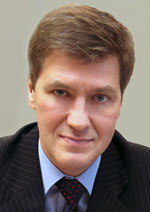
Nikiforov
Vladimir O.
D.Sc., Prof.
Partners
doi: 10.17586/2226-1494-2024-24-4-608-614
Flexible and tractable modeling of multivariate data using composite Bayesian networks
Read the full article

Article in Russian
For citation:
Abstract
For citation:
Deeva I.Yu., Shakhkyan K.A., Kaminsky Yu.K. Flexible and tractable modeling of multivariate data using composite Bayesian networks. Scientific and Technical Journal of Information Technologies, Mechanics and Optics, 2024, vol. 24, no. 4, pp. 608–614 (in Russian). doi: 10.17586/2226-1494-2024-24-4-608-614
Abstract
The article presents a new approach to modeling nonlinear dependencies called composite Bayesian networks. The main emphasis is on integrating machine learning models into Bayesian networks while maintaining their fundamental principles. The novelty of the approach is that it allows us to solve the problem of data inconsistency with traditional assumptions about dependencies. The presented method consists in selecting a variety of machine learning models at the stage of training composite Bayesian networks. This allows you to flexibly customize the nature of the dependencies in accordance with the requirements and dictated characteristics of the modeled object. The software implementation is made in the form of a specialized framework that describes all the necessary functionality. The results of experiments to evaluate the effectiveness of modeling dependencies between features are presented. Data for the experiments was taken from the bnlearn repository for benchmarks and from the UCI repository for real data. The performance of composite Bayesian networks was validated by comparing the likelihood and F1 score with classical Bayesian networks trained with the Hill-Climbing algorithm, demonstrating high accuracy in representing multivariate distributions. The improvement in benchmarks is insignificant since they contain linear dependencies that are well modeled by the classical algorithm. An average 30 % improvement in likelihood was obtained on real UCI datasets. The obtained data can be applied in areas that require modeling complex dependencies between features, for example, in machine learning, statistics, data analysis, as well as in specific subject areas.
Keywords: Bayesian networks, probabilistic graph models, parameter learning, machine learning models, genetic algorithm
Acknowledgements. The research was carried out within the state assignment of the Ministry of Science and Higher Education of the Russian Federation (project No. FSER-2024-0004).
References
Acknowledgements. The research was carried out within the state assignment of the Ministry of Science and Higher Education of the Russian Federation (project No. FSER-2024-0004).
References
- Handbook of Graphical Models. Ed. by M. Maathuis, M. Drton, S. Lauritzen, M. Wainwright. CRC Press, 2018, 554 p. https://doi.org/10.1201/9780429463976
- Mascaro S., Nicholso A.E., Korb K.B. Anomaly detection in vessel tracks using Bayesian networks. International Journal of Approximate Reasoning, 2014, vol. 55, no. 1, pp. 84–98. https://doi.org/10.1016/j.ijar.2013.03.012
- McLachlan S., Dube K., Hitman G.A., Fenton N.E., Kyrimi E. Bayesian networks in healthcare: Distribution by medical condition. Artificial Intelligence in Medicine, 2020, vol. 107, pp. 101912. https://doi.org/10.1016/j.artmed.2020.101912
- Friedman N., Goldszmidt M. Learning Bayesian networks with local structure. NATO ASI Series, 1998, vol. 89, pp. 421–459. https://doi.org/10.1007/978-94-011-5014-9_15
- Grzegorczyk M. An introduction to gaussian bayesian networks. Methods in Molecular Biology, 2010, vol. 662, pp. 121–147. https://doi.org/10.1007/978-1-60761-800-3_6
- Lerner U., Segal E., Koller D. Exact inference in networks with discrete children of continuous parents. arXiv, 2013, arXiv:1301.2289. https://doi.org/10.48550/arXiv.1301.2289
- Pérez A., Larrañaga P., Inza I. Bayesian classifiers based on kernel density estimation: Flexible classifiers. International Journal of Approximate Reasoning, 2009, vol. 50, no. 2, pp. 341–362. https://doi.org/10.1016/j.ijar.2008.08.008
- Ickstadt K., Bornkamp B., Grzegorczyk M., Wieczorek J., Sheriff M.R., Grecco H.E., Zamir E. Nonparametric Bayesian networks. Bayesian Statistics 9, 2011, pp. 283–316. https://doi.org/10.1093/acprof:oso/9780199694587.003.0010
- Deeva I., Bubnova A., Kalyuzhnaya A.V. Advanced approach for distributions parameters learning in Bayesian networks with gaussian mixture models and discriminative models. Mathematics, 2023, vol. 11, no. 2, pp. 343. https://doi.org/10.3390/math11020343
- Langseth H., Nielsen T.D., Rumí R., Salmerón A. Mixtures of truncated basis functions. International Journal of Approximate Reasoning, 2012, vol. 53, no. 2, pp. 212–227. https://doi.org/10.1016/j.ijar.2011.10.004
- Atienza D., Larrañaga P., Bielza C. Hybrid semiparametric Bayesian networks. TEST, 2022, vol. 31, no. 2, pp. 299–327. https://doi.org/10.1007/s11749-022-00812-3
- Sloman S. Causal Models: How People Think about the World and Its Alternatives. Oxford University Press, 2005, 211 p. https://doi.org/10.1093/acprof:oso/9780195183115.001.0001
- Larrañaga P., Karshenas H., Bielza C., Santana R. A review on evolutionary algorithms in Bayesian network learning and inference tasks. Information Sciences, 2013, vol. 233, pp. 109–125. https://doi.org/10.1016/j.ins.2012.12.051
- Gámez J.A., Mateo J.L., Puerta J.M. Learning Bayesian networks by hill climbing: efficient methods based on progressive restriction of the neighborhood. Data Mining and Knowledge Discovery, 2011, vol. 22, no. 1-2, pp. 106–148. https://doi.org/10.1007/s10618-010-0178-6
- Behjati S., Beigy H. Improved K2 algorithm for Bayesian network structure learning. Engineering Applications of Artificial Intelligence, 2020, vol. 91, pp. 103617. https://doi.org/10.1016/j.engappai.2020.103617
- Lerner B., Malka R. Investigation of the K2 algorithm in learning Bayesian network classifiers. Applied Artificial Intelligence, 2011, vol. 25, no. 1, pp. 74–96. https://doi.org/10.1080/08839514.2011.529265