Menu
Publications
2025
2024
2023
2022
2021
2020
2019
2018
2017
2016
2015
2014
2013
2012
2011
2010
2009
2008
2007
2006
2005
2004
2003
2002
2001
Editor-in-Chief
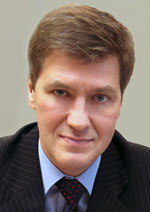
Nikiforov
Vladimir O.
D.Sc., Prof.
Partners
doi: 10.17586/2226-1494-2024-24-6-991-998
Russian parametric corpus RuParam
Read the full article

Article in Russian
For citation:
Abstract
For citation:
Grashchenkov P.V., Pasko L.I., Studenikina K.A., Tikhomirov M.M. Russian parametric corpus RuParam. Scientific and Technical Journal of Information Technologies, Mechanics and Optics, 2024, vol. 24, no. 6, pp. 991–998 (in Russian). doi: 10.17586/2226-1494-2024-24-6-991-998
Abstract
The main function of large language models is to simulate the behavior of native speakers in the most correct way. Hence, it is necessary to have assessment datasets to track progress in solving this problem as well as regularly compare competing models with each other. There are some datasets of this type, the so-called linguistic acceptability corpora. The hypothesis that underlies the creation of these corpora assumes that large language models, like native speakers, should be able to distinguish correct, grammatical sentences from the ungrammatical ones that violate the grammar of the target language. The paper presents the parametric corpus for Russian, RuParam. Our corpus contains 9.5 thousand minimal pairs of sentences that differ in grammaticality — each correct sentence corresponds to a minimally different erroneous one. The source of ungrammaticality in each pair is supplied with the linguistic markup provided by experts. RuParam consists of two parts: the first part uses a totally new data source for the task of testing large language models — lexical and grammatical tests on Russian as a foreign language. The second part consists of (modified and tagged) examples from real texts that represent grammatical phenomena, not included in the RFL teaching program due to their complexity. As have shown our experiments with different Large Language Models, the highest results are achieved by those models that have been trained on Russian most carefully at all stages, from data preparation and tokenization to writing instructions and reinforcement learning (these are first of all YandexGPT and GigaChat). Multilingual models, which usually receive little or no emphasis on Russian, showed significantly lower results. Still, even the best models results are far from the assessors who completed the task with almost 100 % accuracy. The models ranking obtained during the experiment shows that our corpus reflects actual degree of proficiency in Russian. The resulting rating can be helpful when choosing a model for natural language processing task requiring grammar knowledge: for example, building morphological and syntactic parsers. Thereafter, the proposed corpus can be used to test your own models.
Keywords: corpora, Russian, LLM, L2, natural language processing, acceptability judgements, universal grammar
Acknowledgements. This work was done with the support of MSU Program of Development, Project No. 23-SCH02-10 “Linguistic competence of natural language speakers and neural network models”. We also thank the students of the Department of Theoretical and Applied Linguistics of Lomonosov Moscow State University — Maria Kravchuk and Daniil Burmistrov — for their significant help in markup. We also want to express our gratitude to the ABC Elementary crowdsourcing platform (https://elementary.center/) for the gratuitous provision of resources for obtaining human assessments.
References
Acknowledgements. This work was done with the support of MSU Program of Development, Project No. 23-SCH02-10 “Linguistic competence of natural language speakers and neural network models”. We also thank the students of the Department of Theoretical and Applied Linguistics of Lomonosov Moscow State University — Maria Kravchuk and Daniil Burmistrov — for their significant help in markup. We also want to express our gratitude to the ABC Elementary crowdsourcing platform (https://elementary.center/) for the gratuitous provision of resources for obtaining human assessments.
References
- Warstadt A., Singh A., Bowman S.R. Neural network acceptability judgments. Transactions of the Association for Computational Linguistics, 2019, vol. 7, pp. 625–641. https://doi.org/10.1162/tacl_a_00290
- Warstadt A., Parrish A., Liu H., Mohananey A., Peng W., Wang S.-F., Bowman S.R. BLiMP: The benchmark of linguistic minimal pairs for English. Transactions of the Association for Computational Linguistics, 2020, vol. 8, pp. 377–392. https://doi.org/10.1162/tacl_a_00321
- Mikhailov V., Shamardina T., Ryabinin M., Pestova A., Smurov I., Artemova E. RuCoLA: Russian Corpus of Linguistic Acceptability. Proc. of the 2022 Conference on Empirical Methods in Natural Language Processing, 2022, pp. 5207–5227. https://doi.org/10.18653/v1/2022.emnlp-main.348
- Taktasheva E., Bazhukov M., Koncha K., Fenogenova A., Artemova E., Mikhailov V. RuBLiMP: Russian benchmark of linguistic minimal pairs. Proc. of the 2024 Conference on Empirical Methods in Natural Language Processing, 2024, pp. 9268–9299.
- Volodina E., Mohammed Y.A., Klezl J. DaLAJ – a dataset for linguistic acceptability judgments for Swedish. Proc. of the 10th Workshop on Natural Language Processing for Computer Assisted Language Learning (NLP4CALL 2021), 2021, pp. 28–37.
- Jentoft M., Samuel D. NoCoLA: The Norwegian corpus of linguistic acceptability. Proc. of the 24th Nordic Conference on Computational Linguistics (NoDaLiDa), 2023, pp. 610–617.
- Grashchenkov P.V. RuConst: A Treebank for Russian. Lomonosov Philology Journal. Series 9. Philology, 2024, vol. 3, pp. 94–112. (in Russian). https://doi.org/10.55959/MSU0130-0075-9-2024-47-03-7
- Ross J.R. Constraints on variables in syntax: PhD thesis. Massachusetts Institute of Technology. 1967, 523 p.
- Belova D.D., Voznesenskaia A.Iu., Gerasimova A.A. et al. Russian Islands in the Light of the Experimental Data. Moscow, Buki Vedi Publ., 2021, 412 p. (in Russian)
- Chomsky N. Lectures on Government and Binding: The Pisa Lectures. Dordrecht, Walter de Gruyter GmbH & Company KG, 1981, 371 p.
- Schütze C., Sprouse J. Judgment data. Research Methods in Linguistics. Cambridge, Cambridge University Press, 2014, pp. 27–50.
- Wang G., Cheng S., Zhan X., Li X., Song S., Liu Y. OpenChat: Advancing open-source language models with mixed-quality data. arXiv, 2023, arXiv:2309.11235. https://doi.org/10.48550/arXiv.2309.11235
- Grattafiori A., Dubey A., Jauhri A., Pandey A. et al. The Llama 3 Herd of Models. arXiv, 2024, arXiv:2407.21783. https://doi.org/10.48550/arXiv.2407.21783
- Devine P. Tagengo: A multilingual chat dataset. Proc. of the Fourth Workshop on Multilingual Representation Learning (MRL 2024), 2024, pp. 106–113.
- Nikolich A., Korolev K., Shelmanov A. Vikhr: The family of open-source instruction-tuned large language models for Russian. arXiv, 2024, arXiv:2405.13929v2. https://doi.org/10.48550/arXiv.2405.13929