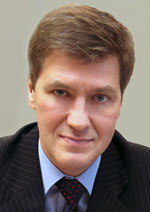
Nikiforov
Vladimir O.
D.Sc., Prof.
doi: 10.17586/2226-1494-2021-21-4-553-561
The Fourier method of IR spectroscopy in the study of parechyma of the normal testicles and ejaculate and the ones with varying degrees of pathology
Read the full article

For citation: Korobeynikov A. G. Application of BIG DATA methods for INTERMAGNET data preprocessing. Journal of Instrument Engineering. 2023. Vol. 66, N 7. P. 533—538 (in Russian). DOI: 10.17586/0021-3454-2023-66-7-533-538.
Abstract
A comparative study of 9 samples of testicular parenchyma of men of different age categories and of normal ejaculate as well as the one with varying degrees of pathology was performed using Fourier IR spectroscopy. It is shown that it is possible to diagnose pronounced testicular tissue pathology by the presence of bands in the ejaculate spectrum in the range of 1575–1578 cm-1 caused by the absorption of peptide formations as a result of partial destruction of protein structures. Spectral visualization of changes in the optical properties of the parenchyma while its quality is deteriorated allowed us to observe an analogy with changes in the optical properties of the ejaculate when the signs of incipient pathology appear and when it is developed. Primary manifestation of incipient pathology in testicular tissue are disorders in its carbohydrate system (1175–750 cm-1), entailing sequential changes in lipid and, as a result, in protein structures. The study of parenchymal samples for the extended age range (47–90 years) allowed us to note that age-related changes in the carbohydrate system of the parenchyma have a completely different character and, if the system is healthy, can occur without noticeable structural changes in the lipid and protein systems. The data obtained confirm the possibilities of the Fourier method of IR spectroscopy as an additional diagnostic tool in the clinical practice of urology and andrology.
Acknowledgements. Исследование выполнено при финансовой поддержке Российского фонда фундаментальных исследований в рамках научного проекта (грант № 19-08-00865 А).
References
1. Zeigarnik V.A., Bogomolov L.M. & Novikov V.A. Izvestiya, Physics of the Solid Earth, 2022, vol. 58, pр. 30–58. 2. https://www.adilet.zan.kz/rus/docs/D18C0000897/. (in Russ.) 3. https://www.adilet.zan.kz/rus/docs/P2300000785/. (in Russ.) 4. https://www.almatydc.kz/press/news/programma_rus/. (in Russ.) 5. https://www.gov.kz/memleket/entities/emer-almaty/documents/details/534612?directionId=4560&lang=ru/. (in Russ.) 6. Pilz M., Abakanov T., Abdrakhmatov K. Annals of Geophysics, 2015, no. 1(58), pp. 7–14, DOI: 10.4401/ag-6662. 7. Sadykova A.B., Silacheva N.V., Stepanenko N.P. Izvestiya NAN RK. Seriya geologii i tekhnicheskikh nauk, 2021, no. 1(445), pp. 20–27, DOI: 10.32014/2021.2518-170X.18. (in Russ.) 8. https://www.tengrinews.kz/kazakhstan_news/raspolojenyi-seysmicheskie-razlomyi-almatyi-podrobnaya-karta-524230/. 9. https://www.darmen.app/. 10. Shokbarov Y. Earthquake risk mitigation policies and managment, 2021, vol. 1, рр. 1595–1600, DOI: 10.5592/ CO/1CroCEE.2021.77. 11. https://www.adilet.zan.kz/rus/docs/P970001286/. (in Russ.) 12. https://www.online.zakon.kz/Document/1003949326/. (in Russ.) 13. SP RK 2.03-30-2017. Svod pravil. Stroitel'stvo v seismicheskikh zonakh (SP RK 2.03-30-2017. Code of Practice. Construction in Seismic Zones), KazNIISA, Replaces SNiP RK 2.03-30-2006, introduced on 20.12.2017, Astana, 2017, 123 р. (in Russ.) 14. https://www.adilet.zan.kz/rus/docs/P2000000088#z11/. (in Russ.) 15. https://www.goszakup.gov.kz/ru/egzcontract/cpublic/show/18355651/. (in Russ.) 16. Ryzhkov S.A. GIS-LAB.INFO: Geograficheskie informatsionnye sistemy i distantsionnoe zondirovanie, 2016, http:// www.gis-lab.info/qa/rugeocoder.html. (in Russ.) 17. https://www.yandex.ru/dev/jsapi-v2-1/doc/ru/#result_map/. (in Russ.) 18. Graser A. International Journal of Geo-Information (IJGI), 2015, no. 4(4), pp. 2219–2245, DOI: 10.3390/ijgi4042219. 19. https://www.yandex.ru/dev/geocode/doc/ru/. (in Russ.) 20. Funkner A.A., Elkhovskaya L.O., Lenivtceva I.D. Procedia computer science, 2021, vol. 19, рр. 22–31, DOI: 10.1016/j. procs.2021.10.003. 21. Rahmatov S.S., Bobonazarov T.Sh., Abdurahmonov O.M. Molodoj uchenyj, 2022, no. 5(1), pp. 9–12. (in Russ.) 22. OpenStreetMap, 2024, https://www.openstreetmap.org/#map=10/43.2572/76.8329/. 23. Ehrig-Page J.C. Cartographic Perspectives, 2020, vol. 95, рр. 42–49, DOI: 10.14714/CP95.1633. 24. Herve P., Kenji O. QGIS and Applications in Water and Risks, 2018, vol. 4, рр. 2425–2444, DOI: 10.1002/9781119476726. 25. Mohammed zeki S. Baghdad Science Journal, 2021, vol. 18, рр. 631–641, DOI: 10.21123/bsj.2022.19.3.0631. 26. Cormen T.H., Leiserson C.E., Rivest R.L., Stein C. Introduction to algorithms, Massachusetts Institute of Technology, 2009, no. 4, 1312 p. 27. Chadha C., Garg S. International Conference on Innovative Computing and Communications, 2019, vol. 55, рр. 201–214, DOI: 10.1007/978-981-13-2324-9_20. 28. https://www.gis-lab.info/qa/road-graph2.html/. (in Russ.) 29. Budarova V.A. Shamsudinov V.A. Stolypinskiy vestnik, 2022, no. 1, pp. 892–904, DOI: 10.55186/27131424_2022_4_2_8. (in Russ.) 30. Debnath P. Infrastructures, 2022, vol. 7, рр. 1–13, DOI: 10.3390/infrastructures7090114. 31. https://www.docs.qgis.org/3.34/ru/docs/user_manual/processing_algs/qgis/database.html/. 32. Guo M., Han C., Guan Q., Huang Y., Xie Z. Transactions in GIS, 2020, vol. 24, рр. 1437–1826, DOI: 10.1111/ tgis.12670. 33. Mericskay B. QGIS and Applications in Territorial Planning, 2018, vol. 3, рр. 1–38, DOI: 10.1002/9781119457121. ch1. 34. QGIS Tutorials and Tips. Automating Map Creation with Print Layout Atlas (QGIS3), 2023, https://www.qgistutorials. com/en/docs/3/automating_map_creation.html/. (in Russ.) 36. QR Code Generator PRO, Denso wave incorporated, https://www.app.qr-code-generator.com/create/ new/?finishedOnboarding=1/. 37. Corel Corporation, 2024, https://www.corel.com/. 38. https://www.orda.kz/gde-perezhdat-zemletrjasenie-informacionnye-tablichki-napugali-almatincev-381264/. (in Russ.) 39. https://www.kndc.kz/index.php/home-ru/kndcnews/227-operativnaya-informatsiya-o-proizoshedshem-zemletryasenii- 23-01-2024-g/. (in Russ.) 40. https://www.gov.kz/memleket/entities/emer-almaty/press/news/details/691169?lang=ru/. (in Russ.) 35.