Меню
Публикации
2025
2024
2023
2022
2021
2020
2019
2018
2017
2016
2015
2014
2013
2012
2011
2010
2009
2008
2007
2006
2005
2004
2003
2002
2001
Главный редактор
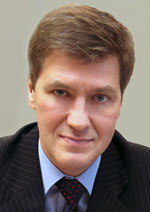
НИКИФОРОВ
Владимир Олегович
д.т.н., профессор
Партнеры
doi: 10.17586/2226-1494-2020-20-2-163-176
УДК 004.932.2
АНАЛИТИЧЕСКИЙ ОБЗОР МЕТОДОВ РАСПОЗНАВАНИЯ ЭМОЦИЙ ПО ВЫРАЖЕНИЯМ ЛИЦА ЧЕЛОВЕКА
Читать статью полностью

Язык статьи - русский
Ссылка для цитирования:
Аннотация
Ссылка для цитирования:
Рюмина Е.В., Карпов А.А. Аналитический обзор методов распознавания эмоций по выражениям лица человека // Научно-технический вестник информационных технологий, механики и оптики. 2020. Т. 20. № 2. С. 163–176. doi: 10.17586/2226-1494-2020-20-2-163-176
Аннотация
Распознавание эмоций человека по мимике его лица является важной научно-исследовательской проблемой, которая охватывает множество областей и дисциплин, такие как компьютерное зрение, искусственный интеллект, медицина, психология и безопасность. В статье приведен аналитический обзор баз видеоданных выражений лица и подходов к распознаванию эмоций по выражениям лица, которые включают в себя три основных этапа анализа изображений, такие как предварительная обработка, извлечение признаков и классификация. Представлены как традиционные подходы к распознаванию эмоций человека по визуальным признакам лица, так и подходы, основанные на глубоком обучении с использованием глубоких нейронных сетей. Приведены современные результаты некоторых существующих алгоритмов. При обзоре научно-технической литературы упор главным образом делался на источники, содержащие теоретическую и исследовательскую информацию рассматриваемых методов, а также сравнение традиционных методов и методов на основе глубоких нейронных сетей, которые подкреплены экспериментальными исследованиями. Анализ научно-технической литературы, описывающий методы и алгоритмы анализа и распознавания выражений лица, а также результаты мировых научных исследований показали, что традиционные методы классификации выражений лица уступают по скорости и точности искусственным нейронным сетям. Основные вклады данного обзора заключаются в следующем: предоставление общего понимания современных подходов к распознаванию выражений лиц, которое позволит новым исследователям понять основные компоненты и тенденции в области распознавания выражений лиц; сравнение результатов мировых научных исследований показало, что комбинирование традиционных подходов и подходов, основанных на глубоких нейронных сетях показывают лучшую точность классификации, однако лучшими методами классификации являются искусственные нейронные сети. Статья может быть полезна специалистам и исследователям в области компьютерного зрения.
Ключевые слова: предварительная обработка изображений, классификация, распознавание выражений лица, извлечение признаков, глубокие нейронные сети, компьютерная паралингвистика
Благодарности. Исследование выполнено при поддержке Российского научного фонда РНФ (проект № 18-11-00145).
Список литературы
Благодарности. Исследование выполнено при поддержке Российского научного фонда РНФ (проект № 18-11-00145).
Список литературы
-
Varma S., Shinde M., Chavan S.S. Analysis of PCA and LDA features for facial expression recognition using SVM and HMM classifiers // Techno-Societal 2018. Proc. 2nd International Conference on Advanced Technologies for Societal Applications. V. 1. 2020. P. 109–119. doi: 10.1007/978-3-030-16848-3_11
-
Yin D.B.M., Mukhlas A.A., Chik R.Z.W., Othman A.T., Omar S. A proposed approach for biometric-based authentication using of face and facial expression recognition // Proc. IEEE 3rd International Conference on Communication and Information Systems (ICCIS 2018). Singapore. 2018. P. 28–33. doi: 10.1109/ICOMIS.2018.8644974
-
Dino H.I., Abdulrazzaq M.B. Facial expression classification based on SVM, KNN and MLP classifiers // Proc. International Conference on Advanced Science and Engineering (ICOASE 2019). Zakho-Duhok, Iraq. 2019. P. 70–75. doi: 10.1109/ICOASE.2019.8723728
-
Tripathi A., Pandey S. Efficient facial expression recognition system based on geometric features using neural network // Lecture Notes in Networks and Systems. 2018. V. 10. P. 181–190. doi: 10.1007/978-981-10-3920-1_18
-
Greche L., Es-Sbai N., Lavendelis E. Histogram of oriented gradient and multi layer feed forward neural network for facial expression identification // Proc. International Conference on Control, Automation and Diagnosis (ICCAD 2017). Hammamet, Tunisia. 2017. P. 333–337. doi: 10.1109/CADIAG.2017.8075680
-
Целикова С.О., Горожанкин Я.П., Иванов А.О., Миронов А.А., Ахремчик Я.В. Использование нейросетевых технологий в задаче автоматического распознавания эмоций // Молодой ученый. 2019. № 26. С. 59–61 [Электронный ресурс]. URL: https://moluch.ru/archive/264/61173/, свободный. Яз. рус. (дата обращения:12.12.2019).
-
Степанова О.А., Ивановский Л.И., Хрящев В.В. Использование глубокого обучения и сверточных нейронных сетей для анализа выражения лица // DSPA: Вопросы применения цифровой обработки сигналов. 2018. Т. 8. № 4. С. 170–173.
-
Talegaonkar I., Joshi K., Valunj S., Kohok R., Kulkarni A. Real time facial expression recognition using deep learning // Proc. of International Conference on Communication and Information Processing (ICCIP). 2019 [Электронный ресурс]. URL: https://ssrn.com/abstract=3421486, свободный. Яз. англ. (дата обращения:13.12.2019). doi: 10.2139/ssrn.3421486
-
Jumani S.Z., Ali F., Guriro S., Kandhro I.A., Khan A., Zaidi A. Facial expression recognition with histogram of oriented gradients using CNN // Indian Journal of Science and Technology. 2019. V. 12. N 24. P. 1–8. doi: 10.17485/ijst/2019/v12i24/145093
-
Babu D.R., Shankar R.S., Mahesh G., Murthy K.V.S.S. Facial expression recognition using bezier curves with hausdorff distance // Proc. IEEE International Conference on IoT and Application (ICIOT 2017). Nagapattinam, India. 2017. P. 8073622. doi: 10.1109/ICIOTA.2017.8073622
-
Cao H., Cooper D.G., Keutmann M.K., Gur R.C., Nenkova A., Verma R.CREMA-D: Crowd-sourced emotional multimodal actors dataset // IEEE Transactions on Affective Computing. 2014. V. 5. N 4. P. 377–390.doi:10.1109/TAFFC.2014.2336244
-
Perepelkina O., Kazimirova E., Konstantinova М.RAMAS: Russian multimodal corpus of dyadic interaction for affective computing // Lecture Notes in Computer Science (including subseries Lecture Notes in Artificial Intelligence and Lecture Notes in Bioinformatics). 2018. V. 11096. Р. 501–510.doi:10.1007/978-3-319-99579-3_52
-
Livingstone S.R., Russo F.A. The Ryerson audio-visual database of emotional speech and song (RAVDESS): A dynamic, multimodal set of facial and vocal expressions in North American English // PLoS ONE. 2018. V. 13. N 5. P. e0196391. doi: 10.1371/journal.pone.0196391
-
Viola P., Jones M.J. Robust real-time face detection // International Journal of Computer Vision. 2004. V. 57. N 2. P. 137–154. doi: 10.1023/B:VISI.0000013087.49260.fb
-
Liu W., Anguelov D., Erhan D., Szegedy C., Reed S., Fu C.-Y., Berg A.C. SSD: single shot multibox detector // Lecture Notes in Computer Science (including subseries Lecture Notes in Artificial Intelligence and Lecture Notes in Bioinformatics). 2016. V. 9905. Р. 21–37. doi:10.1007/978-3-319-46448-0_2
-
Déniz O., Bueno G., Salido J., De la Torre F. Face recognition using histograms of oriented gradients // Pattern Recognition Letters. 2011. V. 32. N 12. Р. 1598–1603. doi:10.1016/j.patrec.2011.01.004
-
King D.E. Max-margin object detection [Электронный ресурс]. URL: https://arxiv.org/pdf/1502.00046.pdf, свободный. Яз. англ.(дата обращения:13.12.2019).
-
Александров А.А., Кирпичников А.П., Ляшева С.А., Шлеймович М.П. Анализ эмоционального состояния человека на изображении // Вестник технологического университета. 2019. Т. 22. № 8. С. 120–123.
-
Voronov V., Strelnikov V., Voronova L., Trunov A., Vovik A. Faces 2D-recognition аnd identification using the HOG descriptors method // Proc. 24th Conference of Open Innovations Association FRUCT. 2019. Р. 783–789.
-
Mohan P.G., Prakash C., Gangashetty S.V. Bessel transform for image resizing // Proc. 18th International Conference on Systems, Signals and Image Processing (IWSSIP 2011). Sarajevo, Bosnia-Herzegovina. 2011. Р. 75–78.
-
Owusu E., Abdulai J.-D., Zhan Y. Face detection based on multilayer feed‐forward neural network and Haar features // Software: Practice and Experience. 2019. V. 49. N 1. Р. 120–129. doi:10.1002/spe.2646
-
Su J., Gao L., Li W., Xia Y., Cao N., Wang R. Fast face tracking-by-detection algorithm for secure monitoring // Applied Sciences. 2019. V. 9. N 18. Р. 3774. doi:10.3390/app9183774
-
Lowe D.G. Distinctive image features from scale-invariant keypoints // International Journal of Computer Vision. 2004. V. 60. N 2. Р. 91–110. doi:10.1023/B:VISI.0000029664.99615.94
-
Hernandez-Matamoros A., Bonarini A., Escamilla-Hernandez E., Nakano-Miyatake M., Perez-Meana H. A facial expression recognition with automatic segmentation of face regions // Communications in Computer and Information Science. 2015. V. 532. Р. 529–540. doi: 10.1007/978-3-319-22689-7_41
-
Naz S., Ziauddin S., Shahid A.R. Driver fatigue detection using mean intensity, SVM, and SIFT // International Journal of Interactive Multimedia and Artificial Intelligence. 2019. V. 5. N 4. Р. 86–93. doi: 10.9781/ijimai.2017.10.002
-
Priya R.V. Emotion recognition from geometric fuzzy membership functions // Multimedia Tools and Applications. 2019. V. 78. N 13. Р. 17847–17878. doi: 10.1007/s11042-018-6954-9
-
Wang X., Chen L. Contrast enhancement using feature-preserving bi-histogram equalization // Signal Image and Video Processing. 2018. V. 12. N 4. Р. 685–692. doi: 10.1007/s11760-017-1208-2
-
Mustapha A., Oulefki A., Bengherabi M., Boutellaa E., Algaet M.A. Towards nonuniform illumination face enhancement via adaptive contrast stretching // Multimedia Tools and Applications. 2017. V. 76. N 21. P. 21961–21999. doi: 10.1007/s11042-017-4665-2
-
Oloyede M., Hancke G., Myburgh H., Onumanyi A. A new evaluation function for face image enhancement in unconstrained environments using metaheuristic algorithms // EURASIP Journal on Image and Video Processing. 2019. N 1. P. 27. doi: 10.1186/s13640-019-0418-7
-
Gao Y., Leung M.K.H. Face recognition using line edge map // IEEE Transactions on Pattern Analysis and Machine Intelligence. 2002. V. 24. N 6. Р. 764–779. doi: 10.1109/TPAMI.2002.1008383
-
Fawwad Hussain M., Wang H., Santosh K.C. Gray level face recognition using spatial features // Communications in Computer and Information Science. 2019. V. 1035. P. 216–229. doi: 10.1007/978-981-13-9181-1_20
-
Cootes T.F., Taylor C.J., Cooper D.H., Graham J. Active shape models-their training and application // Computer Vision and Image Understanding. 1995. V. 61. N 1. P. 38–59. doi: 10.1006/cviu.1995.1004
-
Cootes T.F., Edwards G.J., Taylor C.J. Active appearance models // IEEE Transactions on Pattern Analysis and Machine Intelligence. 2001. V. 23. N 6. P. 681–685. doi: 10.1109/34.927467
-
Iqtait M., Mohamad F.S., Mamat M. Feature extraction for face recognition via active shape model (ASM) and active appearance model (AAM) // IOP Conference Series: Materials Science and Engineering. 2018. V. 332. N 1. P. 012032. doi:10.1088/1757-899X/332/1/012032
-
Бобе А.С., Конышев Д.В., Воротников С.А. Система распознавания базовых эмоций на основе анализа двигательных единиц лица // Инженерный журнал: наука и инновации. 2016. № 9. С. 7. doi: 10.18698/2308-6033-2016-9-1530
-
Candès E., Demanet L., Donoho D., Ying L. Fast discrete curvelet transforms // Multiscale Modeling & Simulation. 2006. V. 5. N 3. P. 861–899. doi: 10.1137/05064182X
-
Fu X., Fu K., Zhang Y., Zhou Q., Fu X. Facial expression recognition based on Curvelet transform and sparse representation // Proc. 14th International Conference on Natural Computation, Fuzzy Systems and Knowledge Discovery (ICNN-FSKD 2018). Huangshan, China. 2018. P. 257–263. doi: 10.1109/FSKD.2018.8686989
-
Ahsan T., Jabid T., Chong U.P. Facial expression recognition using local transitional pattern on Gabor filtered facial images // IETE Technical Review. 2013. V. 30. N 1. P. 47–52. doi: 10.4103/0256-4602.107339
-
Shan C., Gong S., McOwan P.W. Facial expression recognition based on Local Binary Patterns: A comprehensive study // Image and Vision Computing. 2009. V. 27. N 6. P. 803–816. doi: 10.1016/j.imavis.2008.08.005
-
Fan J., Tie Y., Qi L. Facial expression recognition based on multiple feature fusion in video // Proc. International Conference on Computing and Pattern Recognition (ICCPR 2018). Shenzhen, China. 2018. P. 86–92. doi: 10.1145/3232829.3232839
-
Li S., Gong D., Yuan Y. Face recognition using Weber local descriptors // Neurocomputing. 2013. V. 122. P. 272–283. doi: 10.1016/j.neucom.2013.05.038
-
Revina I.M., Emmanuel W.R.S. Face expression recognition using weber local descriptor and F-RBFNN // Proc. 2nd International Conference on Intelligent Computing and Control Systems (ICICCS 2018). Madurai, India. 2018. P. 196–199. doi: 10.1109/ICCONS.2018.8662891
-
Addison P.S. The illustrated wavelet transform handbook: introductory theory and applications in science, engineering, medicine and finance. CRC Press, 2017. 464 p.doi: 10.1201/9781315372556
-
Nigam S., Singh R., Misra A.K. Efficient facial expression recognition using histogram of oriented gradients in wavelet domain // Multimedia Tools and Applications. 2018. V. 77. N 21. P. 28725–28747. doi: 10.1007/s11042-018-6040-3
-
Martínez A.M., Kak A.C. PCA versus LDA // IEEE Transactions on Pattern Analysis and Machine Intelligence. 2001. V. 23. N 2. P. 228–233. doi: 10.1109/34.908974
-
Negahdaripour S. Revised definition of optical flow: Integration of radiometric and geometric cues for dynamic scene analysis // IEEE Transactions on Pattern Analysis and Machine Intelligence. 1998. V. 20. N 9. P. 961–979. doi: 10.1109/34.713362
-
Zhao J., Mao X., Zhang J. Learning deep facial expression features from image and optical flow sequences using 3D CNN // Visual Computer. 2018. V. 34. N 10. P. 1461–1475. doi: 10.1007/s00371-018-1477-y
-
Guo B., Lam K.-M., Siu W.-C., Yang S. Human face recognition using a spatially weighted Hausdorff distance // Proc. IEEE International Symposium on Circuits and Systems (ISCAS 2001). Hong Kong, China. 2001. V. 2. P. 145–148. doi: 10.1109/ISCAS.2001.921027
-
Meftah I.T., Le Thanh N., Amar C.B. Emotion recognition using KNN classification for user modeling and sharing of affect states // Lecture Notes in Computer Science (including subseries Lecture Notes in Artificial Intelligence and Lecture Notes in Bioinformatics). 2012. V. 7663. P. 234–242. doi: 10.1007/978-3-642-34475-6_29
-
Greche L., Akil M., Kachouri R., Es-Sbai N. A new pipeline for the recognition of universal expressions of multiple faces in a video sequence // Journal of Real-Time Image Processing. 2019. in press. doi: 10.1007/s11554-019-00896-5
-
Abdulrahman M., Eleyan A. Facial expression recognition using support vector machines // Proc. 23nd Signal Processing and Communications Applications Conference (SIU 2015). Malatya, Turkey. 2015. P. 276–279. doi: 10.1109/SIU.2015.7129813
-
Aleksic P.S., Katsaggelos A.K. Automatic facial expression recognition using facial animation parameters and multistream HMMs // IEEE Transactions on Information Forensics and Security. 2006. V. 1. N 1. P. 3–11. doi: 10.1109/TIFS.2005.863510
-
Safavian S.R., Landgrebe D. A survey of decision tree classifier methodology // IEEE Transactions on Systems, Man and Cybernetics. 1991. V. 21. N 3. P. 660–674. doi: 10.1109/21.97458
-
Burkert P., Trier F., Afzal M.Z., Dengel A., Liwicki M. Dexpression: Deep convolutional neural network for expression recognition [Электронный ресурс]. URL: https://arxiv.org/abs/1509.05371.pdf, свободный. Яз. англ. (дата обращения: 13.12.2019).
-
Svozil D., Kvasnička V., Pospichal J. Introduction to multi-layer feed-forward neural networks // Chemometrics and Intelligent Laboratory Systems. 1997. V. 39. N 1. P. 43–62. doi: 10.1016/S0169-7439(97)00061-0
-
An F., Liu Z. Facial expression recognition algorithm based on parameter adaptive initialization of CNN and LSTM // Visual Computer. 2020. V. 36. N 3. P. 483–498. doi:10.1007/s00371-019-01635-4
-
Ахремчик Я.В., Горожанкин Я.П., Иванов А.О., Миронов А.А., Целикова С.О. Распознавание и извлечение 3D-моделей по двумерным изображениям // Молодой ученый. 2019. № 26. С. 25–28 [Электронный ресурс]. URL:https://moluch.ru/archive/264/61169/, свободный. Яз. англ. (дата обращения: 02.01.2020).
-
Cao T., Li M. Facial Expression Recognition Algorithm Based on the Combination of CNN and K-Means // Proc. 11th International Conference on Machine Learning and Computing (ICMLC 2019). 2019. P. 400–404. doi: 10.1145/3318299.3318344
-
Karadeniz A.S., Karadeniz M.F., Weber G.W., Husein I. Improving CNN features for facial expression recognition // Zero: Jurnal Sains, Matematika dan Terapan. 2019. V. 3. N 1. P. 1–11. doi: 10.30829/zero.v3i1.5881
-
Kasraoui S., Lachiri Z., Madani K. Tandem modelling based emotion recognition in videos // Lecture Notes in Computer Science (including subseries Lecture Notes in Artificial Intelligence and Lecture Notes in Bioinformatics). 2019. V. 11507. P. 325–336. doi: 10.1007/978-3-030-20518-8_28