Меню
Публикации
2025
2024
2023
2022
2021
2020
2019
2018
2017
2016
2015
2014
2013
2012
2011
2010
2009
2008
2007
2006
2005
2004
2003
2002
2001
Главный редактор
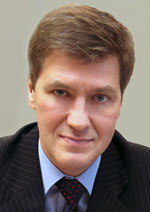
НИКИФОРОВ
Владимир Олегович
д.т.н., профессор
Партнеры
doi: 10.17586/2226-1494-2020-20-6-863-870
УДК 004.67, 66.091
ПРЕДСКАЗАНИЕ УСЛОВИЙ РЕАКЦИЙ С ПОМОЩЬЮ МЕТОДОВ ГЛУБОКОГО ОБУЧЕНИЯ
Читать статью полностью

Язык статьи - русский
Ссылка для цитирования:
Аннотация
Ссылка для цитирования:
Москалев В.Б., Путин Е.О. Предсказание условий реакций с помощью методов глубокого обучения // Научно-технический вестник информационных технологий, механики и оптики. 2020. Т. 20. № 6. С. 863-870. doi: 10.17586/2226-1494-2020-20-6-863-870
Аннотация
Предмет исследования. Исследован метод предсказания различных свойств химических реакций: тип реакции, подходящие группы растворителей, катализаторов для проведения реакции. В качестве представления реакций рассчитаны разницы молекулярных отпечатков пальцев между продуктами и реагентами с использованием химической библиотеки RdKit. Молекулярные отпечатки пальцев широко используются для предсказания различных свойств молекул. Знание условий проведения реакции необходимо для успешного планирования ретросинтеза. Методы хемоинформатики могут эффективно находить взаимосвязь между реагентами реакции и необходимыми условиями для проведения реакции. При этом уменьшаются затраты времени и ресурсов на определение набора необходимых условий для проведения реакции. Прогноз групп растворителей может значительно улучшить качество моделей и применимость подходов. Метод. В качестве моделей машинного обучения применены LightGBM и нейронная сеть с механизмом выбора признаков Deep Feature Selection. Результаты оценивались с помощью метрики F1. Для обучения и оценки моделей данные были разбиты на химически непохожие части. Для перебора параметров использовалась байесовская оптимизация. Основные результаты. Выполнены эксперименты по предсказанию типа реакции, катализаторов и групп растворителей для проведения реакции. Полученные результаты показали, что на основе разницы молекулярных отпечатков между реагентами и продуктами модели машинного обучения можно предсказывать в среднем с мерой метрики F1: тип реакции MLP = 0,99, катализатор MLP = 0,7 и группу растворителей MLP = 0,68. Работа охватывает значительное количество катализаторов и растворителей. Практическая значимость. Автоматизированное планирование ретросинтеза является одним из актуальных направлений исследований. В ходе планирования составляется последовательность необходимых реакций. Предлагаемый метод может быть применен для выработки рекомендаций возможных группы катализаторов и растворителей и позволяет уменьшить затраты ресурсов и времени на определение необходимых условий реакции.
Ключевые слова: нейронные сети, реакции, органическая химия, машинное обучение, тип реакции, катализатор, растворитель, синтез
Список литературы
Список литературы
1. Marcou G., Aires de Sousa J., Latino D.A., De Luca A., Horvath D., Rietsch V., Varnek A. Expert system for predicting reaction conditions: The Michael reaction case // Journal of Chemical Information and Modeling. 2015. V. 55. N 2. P. 239–250. doi: 10.1021/ci500698a
2. Johansson Seechurn C.C., Kitching M.O., Colacot T.J., Snieckus V. Palladium-catalyzed cross-coupling: a historical contextual perspective to the 2010 Nobel Prize // Angewandte Chemie. International Edition. 2012. V. 51. N 21. P. 5062–5085. doi: 10.1002/anie.201107017
3. Roughley S.D., Jordan A.M. The medicinal chemist’s toolbox: an analysis of reactions used in the pursuit of drug candidates // Journal of Medicinal Chemistry. 2011. V. 54. N 10. P. 3451–3479. doi: 10.1021/jm200187y
4. Biffis A., Centomo P., Del Zotto A., Zecca M. Pd metal catalysts for cross-couplings and related reactions in the 21st century: a critical review // Chemical Reviews. 2018. V. 118. N 4. P. 2249–2295. doi: 10.1021/acs.chemrev.7b00443
5. Heck R.F., Nolley J.P.,Jr. Palladium-catalyzed vinylic hydrogen substitution reactions with aryl, benzyl, and styryl halides // Journal of Organic Chemistry. 1972. V. 37. N 14. P. 2320–2322. doi: 10.1021/jo00979a024
6. Christmann U., Vilar R. Monoligated palladium species as catalysts in cross-coupling reactions // Angewandte Chemie. International Edition. 2005. V. 44. N 3. P. 366–374. doi: 10.1002/anie.200461189
7. Beletskaya I.P., Cheprakov A.V. Copper in cross-coupling reactions: The post-Ullmann chemistry // Coordination Chemistry Reviews. 2004. V. 248. P. 2337–2364. doi: 10.1016/j.ccr.2004.09.014
8. Proutiere F., Schoenebeck F. Solvent effect on palladium-catalyzed cross-coupling reactions and implications on the active catalytic species // Angewandte Chemie. International Edition. 2011. V. 50. N 35. P. 8192–8195. doi: 10.1002/anie.201101746
9. Sherwood J., Clark J.H., Fairlamb I.J., Slattery J.M. Solvent effects in palladium catalysed cross-coupling reactions // Green Chemistry. 2019. V. 21. N 9. P. 2164–2213. doi: 10.1039/C9GC00617F
10. Lima C.F., Rodrigues A.S., Silva V.L., Silva A.M., Santos L.M. Role of the base and control of selectivity in the Suzuki–Miyaura cross-coupling reaction // ChemCatChem. 2014. V. 6. N 5. P. 1291–1302. doi: 10.1002/cctc.201301080
11. Goodman J. Computer Software Review: Reaxys // Journal of Chemical Information and Modeling. 2009. V. 49. N 12. P. 2897–2898. doi: 10.1021/ci900437n
12. Gilmer J., Schoenholz S.S., Riley P.F., Vinyals O., Dahl G.E. Neural message passing for quantum chemistry // Proc. of the 34th International Conference on Machine Learning (ICML 2017). 2017. P. 1263–1272.
13. Kornblith S., Shlens J., Le Q.V. Do better imagenet models transfer better? // Proc. of the IEEE Conference on Computer Vision And Pattern Recognition. 2019. P. 2656–2666. doi: 10.1109/CVPR.2019.00277
14. Kumar A., Irsoy O., Ondruska P., Iyyer M., Bradbury J., Gulrajani I., Zhong V., Paulus R., Socher R. Ask me anything: Dynamic memory networks for natural language processing // Proc. 33rd International Conference on Machine Learning (ICML 2016). 2016. P. 2068–2078.
15. Hershey S., Chaudhuri S., Ellis D.P.W., Gemmeke J.F., Jansen A., Moore R.C., Plakal M., Platt D., Saurous R.A., Seybold B., Slaney M., Weiss R.J., Wilson K. CNN architectures for large-scale audio classification // Proc. of the IEEE International Conference on Acoustics, Speech and Signal Processing (ICASSP 2017). 2017. P. 131–135. doi: 10.1109/ICASSP.2017.7952132
16. Schwaller P., Laino T., Gaudin T., Bolgar P., Hunter C.A., Bekas C., Lee A.A. Molecular transformer: A model for uncertainty-calibrated chemical reaction prediction // ACS Central Science. 2019. V. 5. N 9. P. 1572–1583. doi: 10.1021/acscentsci.9b00576
17. Lowe D. Chemical reactions from US patents (1976-Sep2016). 2017 [Электронный ресурс]. URL: https://figshare.com/articles/Chemical_reactions_from_US_patents_1976-Sep2016_/5104873 (дата обращения: 27.02.2020).
18. Schreck J.S., Coley C.W., Bishop K.J. Learning retrosynthetic planning through simulated experience // ACS Central Science. 2019. V. 5. N 6. P. 970–981. doi: 10.1021/acscentsci.9b00055
19. Ahneman D.T., Estrada J.G., Lin S., Dreher S.D., Doyle A.G. Predicting reaction performance in C–N cross-coupling using machine learning // Science. 2018. V. 360. N 6385. P. 186–190. doi: 10.1126/science.aar5169
20. Cutler A., Cutler D.R, Stevens J.R. Random Forests // Ensemble Machine Learning. Springer, 2012. P. 157–175. doi: 10.1007/978-1-4419-9326-7_5
21. Forero-Cortés P.A., Haydl A.M. The 25th Anniversary of the Buchwald–Hartwig amination: development, applications, and outlook // Organic Process Research & Development. 2019. V. 23. N 8. P. 1478–1483. doi: 10.1021/acs.oprd.9b00161
22. Devore J.L. Probability and Statistics for Engineering and the Sciences. Cengage learning, 2011. 776 p.
23. Zahrt A.F., Henle J.J., Rose B.T., Wang Y., Darrow W.T., Denmark S.E. Prediction of higher-selectivity catalysts by computer-driven workflow and machine learning // Science. 2019. V. 363. N 6424. P. eaau5631. doi: 10.1126/science.aau5631
24. Gao H., Struble T.J., Coley C.W., Wang Y., Green W.H., Jensen K.F. Using machine learning to predict suitable conditions for organic reactions // ACS Central Science. 2018. V. 4. N 11. P. 1465–1476. doi: 10.1021/acscentsci.8b00357
25. Schneider N., Lowe D.M., Sayle R.A., Landrum G.A. Development of a novel fingerprint for chemical reactions and its application to large-scale reaction classification and similarity // Journal of Chemical Information and Modeling. 2015. V. 55. N 1. P. 39–53. doi: 10.1021/ci5006614
26. Kar R.K. Fundamentals of Organic Synthesis the Retrosynthetic Analysis. New Central Book Agency, 2014. 710 p.
27. Segler M.H., Preuss M., Waller M.P. Planning chemical syntheses with deep neural networks and symbolic AI // Nature. 2018. V. 555. N 7698. P. 604–610. doi: 10.1038/nature25978
28. Browne C.B., Powley E., Whitehouse D., Lucas S.M., Cowling P.I., Rohlfshagen P., Tavener S., Perez D., Samothrakis S., Colton S. A survey of monte carlo tree search methods // IEEE Transactions on Computational Intelligence and AI in Games. 2012. V. 4. N 1. P. 1–43. doi: 10.1109/TCIAIG.2012.2186810
29. Vaswani A., Shazeer N., Parmar N., Uszkoreit J., Jones L., Gomez A.N., Kaiser Ł., Polosukhin I. Attention is all you need // Advances in Neural Information Processing Systems. 2017. V. 30. P. 5998–6008.
30. Weininger D. SMILES, a chemical language and information system. 1. Introduction to methodology and encoding rules // Journal of Chemical Information and Computer Sciences. 1988. V. 28. N 1. P. 31–36. doi: 10.1021/ci00057a005
31. Mc Cartney D., Guiry P.J. The asymmetric Heck and related reactions // Chemical Society Reviews. 2011. V. 40. N 10. P. 5122–5150. doi: 10.1039/C1CS15101K
32. Chinchilla R., Nájera C. Recent advances in Sonogashira reactions // Chemical Society Reviews. 2011. V. 40. N 10. P. 5084–5121. doi: 10.1039/C1CS15071E
33. Amatore C., Jutand A., Le Duc G. Kinetic data for the transmetalation/reductive elimination in palladium-catalyzed Suzuki-Miyaura reactions: Unexpected triple role of hydroxide ions Used as Base // Chemistry–A European Journal. 2011. V. 17. N 8. P. 2492–2503. doi: 10.1002/chem.201001911
34. Guasch L., Sitzmann M., Nicklaus M.C. Enumeration of Ring-Chain Tautomers Based on SMIRKS Rules // Journal of Chemical Information and Modeling. 2014. V. 54. N 9. P. 2423–2432. doi: 10.1021/ci500363p
35. Ehmki E.S.R., Schmidt R., Ohm F., Rarey M. Comparing molecular patterns using the example of SMARTS: Applications and filter collection analysis // Journal of Chemical Information and Modeling. 2019. V. 59. N 6. P. 2572–2586. doi: 10.1021/acs.jcim.9b00249
36. Pedregosa F., Varoquaux G., Gramfort A., Michel V., Thirion B., Grisel O., Blondel M., Prettenhofer P., Weiss R., Dubourg V., Vanderplas J., Passos A., Cournapeau D., Brucher M., Perrot M., Duchesnay E. Scikit-learn: Machine learning in Python // Journal of Machine Learning Research. 2011. V. 12. P. 2825–2830.
37. Bemis G.W., Murcko M.A. The properties of known drugs. 1. Molecular frameworks // Journal of Medicinal Chemistry. 1996. V. 39. N 15. P. 2887–2893. doi: 10.1021/jm9602928
38. Sechidis K., Tsoumakas G., Vlahavas I. On the stratification of multi-label data // Lecture Notes in Computer Science (including subseries Lecture Notes in Artificial Intelligence and Lecture Notes in Bioinformatics). 2011. V. 6913. P. 145–158. doi: 10.1007/978-3-642-23808-6_10
39. Szymański P., Kajdanowicz T. A Network Perspective on Stratification of Multi-Label Data // Proceedings of Machine Learning Research. 2017. V. 74. P. 22–35.
40. Szymánski P., Kajdanowicz T. Scikit-multilearn: A scikit-based Python environment for performing multi-label classification // Journal of Machine Learning Research. 2019. V. 20. P. 6.
41. Ramchoun H., Idrissi M.A.J., Ghanou Y., Ettaouil M. Multilayer perceptron: Architecture optimization and training // International Journal of Interactive Multimedia and Artificial Intelligence. 2016. V. 4. N 1. P. 26–30. doi: 10.9781/ijimai.2016.415
42. Ke G., Meng Q., Finley T., Wang T., Chen W., Ma W., Ye Q., Liu T.-Y. LightGBM: A highly efficient gradient boosting decision tree // Advances in Neural Information Processing Systems. 2017. P. 3147–3155.
43. Paszke A., Gross S., Massa F., Lerer A., Bradbury J., Chanan G., Killeen T., Lin Z., Gimelshein N., Antiga L., Desmaison A., Kopf A., Yang E., DeVito Z., Raison M., Tejani A., Chilamkurthy S., Steiner B., Fang L., Bai J., Chintala S. PyTorch: An imperative style, high-performance deep learning library // Advances in Neural Information Processing Systems. 2019. V. 32.
44. Greg Landrum; Paolo Tosco; Brian Kelley; sriniker; gedeck; Riccardo Vianello; Ric; NadineSchneider; Andrew Dalke; Dan N; Brian Cole; Eisuke Kawashima; Samo Turk; Matt Swain; AlexanderSavelyev; Alain Vaucher; David Cosgrove; Maciej Wójcikowski; Daniel Probst; guillaume godin; Axel Pahl; Francois Berenger; JLVarjo; jones-gareth; strets123; JP; DoliathGavid; Gianluca Sforna; Jan Holst Jensen; Patrick Fuller. (2020, August 12). rdkit/rdkit: 2020_03_5 (Q1 2020) Release (Version Release_2020_03_5). Zenodo [Электронный ресурс]. URL: doi.org/10.5281/zenodo.3981263 (дата обращения: 27.02.2020). doi: 10.5281/zenodo.3981263
45. Nilakantan R., Bauman N., Dixon J.S., Venkataraghavan R. Topological torsion: a new molecular descriptor for SAR applications. Comparison with other descriptors // Journal of Chemical Information and Computer Sciences. 1987. V. 27. N 2. P. 82–85. doi: 10.1021/ci00054a008
46. Carhart R.E., Smith D.H., Venkataraghavan R. Atom pairs as molecular features in structure-activity studies: definition and applications // Journal of Chemical Information and Computer Sciences. 1985. V. 25. N 2. P. 64–73. doi: 10.1021/ci00046a002
47. Derczynski L. Complementarity, F-score, and NLP evaluation // Proc. of the 10th International Conference on Language Resources and Evaluation (LREC’16). 2016. P. 261–266.
48. Tim Head; MechCoder; Gilles Louppe; Iaroslav Shcherbatyi; fcharras; Zé Vinícius; cmmalone; Christopher Schröder; nel215; Nuno Campos; Todd Young; Stefano Cereda; Thomas Fan; rene-rex; Kejia (KJ) Shi; Justus Schwabedal; carlosdanielcsantos; Hvass-Labs; Mikhail Pak; SoManyUsernamesTaken; Fred Callaway; Loïc Estève; Lilian Besson; Mehdi Cherti; Karlson Pfannschmidt; Fabian Linzberger; Christophe Cauet; Anna Gut; Andreas Mueller; Alexander Fabisch. (2018, March 25). scikit-optimize/scikit-optimize: v0.5.2 (Version v0.5.2). Zenodo [Электронный ресурс]. URL: doi.org/10.5281/zenodo.1207017 (дата обращения: 27.02.2020). doi: 10.5281/zenodo.1207017