Меню
Публикации
2025
2024
2023
2022
2021
2020
2019
2018
2017
2016
2015
2014
2013
2012
2011
2010
2009
2008
2007
2006
2005
2004
2003
2002
2001
Главный редактор
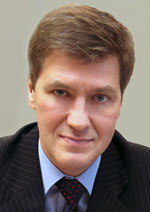
НИКИФОРОВ
Владимир Олегович
д.т.н., профессор
Партнеры
doi: 10.17586/2226-1494-2021-21-4-535-544
УДК 004.85
Байесовские функции потерь для моделирования гомоскедастичной алеаторной неопределенности в задаче детекции пыльцы на изображениях
Читать статью полностью

Язык статьи - русский
Ссылка для цитирования:
Аннотация
Ссылка для цитирования:
Ханжина Н.Е. Байесовские функции потерь для моделирования гомоскедастичной алеаторной неопределенности в задаче детекции пыльцы на изображениях // Научно-технический вестник информационных технологий, механики и оптики. 2021. Т. 21, № 4. С. 535–544. doi: 10.17586/2226-1494-2021-21-4-535-544
Аннотация
Предмет исследования. Представлены результаты моделирования гомоскедастичной алеаторной неопределенности для детекции пыльцевых зерен на изображениях. Приведено описание новых разработанных функций потерь моделирования неопределенности, основанных на фокусной и сглаженной L1 функциях потерь. Актуальность и отличие от существующих функций состоит в том, что базовые фокусная и сглаженная L1 функции, являясь передовыми для решения задачи детекции объектов на изображении, не позволяют оценить алеаторную неопределенность. Разработанные функции позволяют дать такую оценку и представить более точное решение задачи детекции пыльцы. Метод. Предложены функции потерь для обучения нейронной сети RetinaNet, моделирующие гомоскедастичную алеаторную неопределенность, для детекции объектов на изображении. Функции получены с помощью байесовского вывода и позволяют использовать его в существующих нейросетевых детекторах, основанных на архитектуре RetinaNet. Преимущества функций потерь продемонстрированы на задаче детекции изображений пыльцы. Основные результаты. С помощью новых функций потерь удалось повысить точность детекции пыльцы, а именно локализации и классификации, на изображениях в среднем на 2,76 %. Показано, что моделирование гомоскедастичной алеаторной неопределенности в процессе обучения нейронной сети позволяет отделять шум, присущий данным, от сигнала, тем самым повышая точность решения задач. Практическая значимость. Предложенные функции потерь позволяют значительно повысить точность детекции пыльцы на изображениях, что имеет решающее значение для распознавания пыльцы в целом. Результаты работы дадут возможность автоматизировать процесс определения пыльцы аллергенов в воздухе и сократить время информирования больных поллинозами для предупреждения симптомов аллергии. Разработанные функции могут быть применены для обучения нейронной сети для детекции на любых других наборах данных изображений.
Ключевые слова: байесовское глубокое обучение, байесовский вывод, алеаторная неопределенность, распознавание пыльцы, детекция объектов на изображении, оценка неопределенности, байесовское моделирование
Благодарности. Исследование выполнено при финансовой поддержке Национального центра когнитивных разработок Университета ИТМО. Автор выражает благодарность А.С. Лапенку, Н.В. Минаевой, Л.В. Новоселовой, Г.А. Заморину, Т.А. Полевой, А.А. Фильченкову, Е.Б. Замятиной, И.В. Харисовой, Ю. Пинаевой, Е.А. Цымбалову за неоценимую помощь в исследовании.
Список литературы
Благодарности. Исследование выполнено при финансовой поддержке Национального центра когнитивных разработок Университета ИТМО. Автор выражает благодарность А.С. Лапенку, Н.В. Минаевой, Л.В. Новоселовой, Г.А. Заморину, Т.А. Полевой, А.А. Фильченкову, Е.Б. Замятиной, И.В. Харисовой, Ю. Пинаевой, Е.А. Цымбалову за неоценимую помощь в исследовании.
Список литературы
-
Pawankar R., Canonica G.W., Holgate S., Lockey R. WAO White Book on Allergy. USA: World Allergy Organization, 2013. 242 p.
-
Bousquet P.J., Chinn S., Janson C., Kogevinas M., Burney P., Jarvis D. Geographical variation in the prevalence of positive skin tests to environmental aeroallergens in the European Community Respiratory Health Survey I // Allergy. 2007. V. 62. N 3. P. 301–309. https://doi.org/10.1111/j.1398-9995.2006.01293.x
-
D'Amato G., Cecchi L., Liccardi G. Thunderstorm-related asthma: not only grass pollen and spores // Journal of Allergy and Clinical Immunology. 2008. V. 121. N 2. P. 537–539. https://doi.org/10.1016/j.jaci.2007.10.046
-
De Weger L.A., Bergmann K.C., Rantio-Lehtimäki A., Dahl A., Buters J., Déchamp C., Belmonte J., Thibaudon M., Cecchi L., Besancenot J.-P., Galán C., Waisel Y. Impact of Pollen // Allergenic Pollen: A Review of the Production, Release, Distribution and Health Impacts. Springer, 2013. P. 161–215. https://doi.org/10.1007/978-94-007-4881-1_6
-
Jaeger S. The trouble with threshold values for allergy forecasts // Aerobiological Monographs. Towards a comprehensive vision / ed. by B. Clot, P. Comtois, B. Escamilla-Garcia. 2006. P. 233–245.
-
Caillaud D.M., Martin S., Segala C., Besancenot J.-P., Clot B., Thibaudon M., Nonlinear short-term effects of airborne Poaceae levels on hay fever symptoms // Journal of Allergy and Clinical Immunology. 2012. V. 130. N 3. P. 812–814. https://doi.org/10.1016/j.jaci.2012.04.034
-
Committee for Medicinal Products for Human Use. European Medicines Agency Committee for Medicinal Products for Human Use (CHMP) guideline on the evaluation of anticancer medicinal products in man. London, UK: European Medicines Agency, 2006.
-
Sikoparija B., Skjøth C.A., Celenk S. et al. Spatial and temporal variations in airborne Ambrosia pollen in Europe // Aerobiologia. 2017. V. 33. N 2. P. 181–189. https://doi.org/10.1007/s10453-016-9463-1
-
Novoselova L.V., Minaeva N. Pollen monitoring in Perm Krai (Russia)-experience of 6 years // Acta Agrobotanica. 2015. V. 68. N 4. P. 343–348. https://doi.org/10.5586/aa.2015.042
-
Pfaar O., Bastl K., Berger U., Buters J., Calderon M.A., Clot B., Darsow U., Demoly P., Durham S.R., Gala’n C., Gehrig R., Gerth van Wijk R., Jacobsen L., Klimek L., Sofiev M., Thibaudon M., Bergmann K.C. Definition von Pollenexpositionszeiten für klinische Studien zur Allergen-Immuntherapie bei polleninduzierter Rhinokonjunktivitis–ein EAACI-Positionspapier // Allergologie. 2018. V. 41. N 9. P. 386–389. (in German). https://doi.org/10.5414/ALX02053
-
Holt K.A., Bennett K.D. Principles and methods for automated palynology // New Phytologist. 2014. V. 203. N 3. P. 735–742. https://doi.org/10.1111/nph.12848
-
Flenley J.R. The problem of pollen recognition // Problems in Picture Interpretation / ed. by M.B. Clowes, J.P. Penny. Canberra: CSIRO, 1968. P. 141–145.
-
Boucher A., Hidalgo P.J., Thonnat M., Belmonte J., Galan C., Bonton P., Tomczak R. Development of a semi-automatic system for pollen recognition // Aerobiologia. 2002. V. 18. N 3-4. P. 195–201. https://doi.org/10.1023/A:1021322813565
-
Chen C., Hendriks E.A., Duin R.P.W., Reiber J.H.C., Hiemstra P.S., de Weger L.A., Stoel B.C. Feasibility study on automated recognition of allergenic pollen: grass, birch and mugwort // Aerobiologia. 2006. V. 22. N 4. P. 275–284. https://doi.org/10.1007/s10453-006-9040-0
-
Ronneberger O., Schultz E., Burkhardt H. Automated pollen recognition using 3D volume images from fluorescence microscopy // Aerobiologia. 2002. V. 18. N 2. P. 107–115. https://doi.org/10.1023/A:1020623724584
-
Chica M. Authentication of bee pollen grains in bright‐field microscopy by combining one‐class classification techniques and image processing // Microscopy Research and Technique. 2012. V. 75. N 11. P. 1475–1485. https://doi.org/10.1002/jemt.22091
-
Chudyk C., Castaneda H., Leger R., Yahiaoui I., Boochs F. Development of an automatic pollen classification system using shape, texture and aperture features // CEUR Workshop Proceedings. 2015. V. 1458. P. 65–74.
-
Khanzhina N., Putin E. Pollen recognition for allergy and asthma management using gist features // Communications in Computer and Information Science. 2016. V. 674. P. 515–525. https://doi.org/10.1007/978-3-319-49700-6_51
-
Khanzhina N., Putin E., Filchenkov A., Zamyatina E. Pollen grain recognition using convolutional neural network // Proc. 26th European Symposium on Artificial Neural Networks, Computational Intelligence and Machine Learning (ESANN). 2018. P. 409–414.
-
Daood A., Ribeiro E., Bush M. Sequential recognition of pollen grain Z-stacks by combining CNN and RNN // Proc. 31st International Florida Artificial Intelligence Research Society Conference (FLAIRS). 2018. P. 8–13.
-
Sevillano V., Holt K., Aznarte J.L. Precise automatic classification of 46 different pollen types with convolutional neural networks // PLoS ONE. 2020. V. 15. N 6. P. e0229751. https://doi.org/10.1371/journal.pone.0229751
-
Schiele J., Rabe F., Schmitt M., Glaser M., Häring F., Brunner J.O., Bauer B., Schuller B., Traidl-Hoffmann C., Damialis A. Automated classification of airborne pollen using neural networks // Proc. 41st Annual International Conference of the IEEE Engineering in Medicine and Biology Society (EMBC). 2019. P. 4474–4478. https://doi.org/10.1109/EMBC.2019.8856910
-
Northcutt C.G., Athalye A., Mueller J. Pervasive label errors in test sets destabilize machine learning benchmarks // arXiv.org. 2021. arXiv:2103.14749.
-
Kendall A., Gal Y. What uncertainties do we need in bayesian deep learning for computer vision? // Proc. 31st Annual Conference on Neural Information Processing Systems (NIPS). 2017. P. 5575–5585.
-
Cipolla R., Gal Y., Kendall A. Multi-task learning using uncertainty to weigh losses for scene geometry and semantics // Proc. 31st Meeting of the IEEE/CVF Conference on Computer Vision and Pattern Recognition (CVPR). 2018. P. 7482–7491. https://doi.org/10.1109/CVPR.2018.00781
-
Bendale A., Boult T.E. Towards open set deep networks // Proc. 29th IEEE Conference on Computer Vision and Pattern Recognition (CVPR). 2016. P. 1563–1572. https://doi.org/10.1109/CVPR.2016.173
-
Harakeh A., Smart M., Waslander S.L. BayesOD: A bayesian approach for uncertainty estimation in deep object detectors // Proc. 2020 IEEE International Conference on Robotics and Automation (ICRA). 2020. P. 87–93. https://doi.org/10.1109/ICRA40945.2020.9196544
-
Wirges S., Reith-Braun M., Lauer M., Stiller C. Capturing object detection uncertainty in multi-layer grid maps // Proc. 30th IEEE Intelligent Vehicles Symposium. 2019. P. 1520–1526. https://doi.org/10.1109/IVS.2019.8814073
-
Miller D., Nicholson L., Dayoub F., Sünderhauf N. Dropout sampling for robust object detection in open-set conditions // Proc. 2018 IEEE International Conference on Robotics and Automation (ICRA). 2018. P. 3243–3249. https://doi.org/10.1109/ICRA.2018.8460700
-
Miller D., Dayoub F., Milford M., Sünderhauf N. Evaluating merging strategies for sampling-based uncertainty techniques in object detection // Proc. 2019 International Conference on Robotics and Automation (ICRA). 2019. P. 2348–2354. https://doi.org/10.1109/ICRA.2019.8793821
-
Miller D., Sünderhauf N., Milford M., Dayoub F. Uncertainty for identifying open-set errors in visual object detection // arXiv.org. 2021. arXiv:2104.01328.
-
Postels J., Ferroni F., Coskun H., Navab N., Tombari F. Sampling-free epistemic uncertainty estimation using approximated variance propagation // Proc. 17th IEEE/CVF International Conference on Computer Vision (ICCV). 2019. P. 2931–2940. https://doi.org/10.1109/ICCV.2019.00302
-
Kraus F., Dietmayer K. Uncertainty estimation in one-stage object detection // Proc. 2019 IEEE Intelligent Transportation Systems Conference (ITSC). 2019. P. 53–60. https://doi.org/10.1109/ITSC.2019.8917494
-
Le M.T., Diehl F., Brunner T., Knol A. Uncertainty estimation for deep neural object detectors in safety-critical applications // Proc. 21st International Conference on Intelligent Transportation Systems (ITSC). 2018. P. 3873–3878. https://doi.org/10.1109/ITSC.2018.8569637
-
Lin T.-Y., Goyal P., Girshick R., He K., Dollar P. Focal loss for dense object detection // Proc. 16th IEEE International Conference on Computer Vision (ICCV). 2017. P. 2999–3007. https://doi.org/10.1109/ICCV.2017.324
-
Huber P.J. Robust estimation of a location parameter // Breakthroughs in Statistics. New York, NY: Springer, 1992. P. 492–518.
-
Handbook of Mathematical Functions: With Formulas, Graphs, and Mathematical Tables / ed. by M. Abramowitz, I.A. Stegun, R.H. Romer. U.S. Government Printing Office, 1988. 1046 p.
-
Khanzhina N., Lapenok L., Filchenkov A. Towards robust object detection: Bayesian RetinaNet for homoscedastic aleatoric uncertainty modeling: preprint: submitted to the 37th Conference on Uncertainty in Artificial Intelligence (UAI 2021) [Электронный ресурс]. URL: http://genome.ifmo.ru/files/papers_files/UAI(дата обращения: 17.06.2021)
-
He K., Zhang X., Ren S., Sun J. Deep residual learning for image recognition // Proc. 29th IEEE Conference on Computer Vision and Pattern Recognition (CVPR). 2016. P. 770–778. https://doi.org/10.1109/CVPR.2016.90
-
Chollet F. et al. Keras: The python deep learning library // Astrophysics Source Code Library. 2018. P. ascl: 1806.022.
-
Kingma D.P., Ba J.L. Adam: A method for stochastic optimization // Proc. 3rd International Conference on Learning Representations (ICLR). 2015.
-
Du X., Lin T.-Y., Jin P., Ghiasi G., Tan M., Cui Y., Le Q.V., Song X. SpineNet: Learning scale-permuted backbone for recognition and localization // Proc. 2020 IEEE/CVF Conference on Computer Visionand Pattern Recognition (CVPR). 2020. P. 11589–11598. https://doi.org/10.1109/CVPR42600.2020.01161
-
Zhang S., Chi C., Yao Y., Lei Z., Li S.Z. Bridging the gap between anchor-based and anchor-free detection via adaptive training sample selection // Proc. 2020 IEEE/CVF Conference on Computer Vision and Pattern Recognition (CVPR). 2020. P. 9756–9765. https://doi.org/10.1109/CVPR42600.2020.00978