Меню
Публикации
2025
2024
2023
2022
2021
2020
2019
2018
2017
2016
2015
2014
2013
2012
2011
2010
2009
2008
2007
2006
2005
2004
2003
2002
2001
Главный редактор
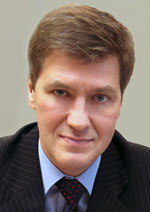
НИКИФОРОВ
Владимир Олегович
д.т.н., профессор
Партнеры
doi: 10.17586/2226-1494-2022-22-3-528-537
УДК 004.932.2
Облачная интеллектуальная система мониторинга для обнаружения нарушений маски и выдачи предупреждений
Читать статью полностью

Язык статьи - английский
Ссылка для цитирования:
Аннотация
Ссылка для цитирования:
Комал Венугопал В., Лалит М., Арун Кумар Т., Джаяшри Д., Виджаяшри Д. Облачная интеллектуальная система мониторинга для обнаружения нарушений ношения маски и выдачи предупреждений // Научно-технический вестник информационных технологий, механики и оптики. 2022. Т. 22, № 3. С. 528–537 (на англ. яз.). doi: 10.17586/2226-1494-2022-22-3-528-537
Аннотация
Важность ношения маски в общественных местах стала очевидна, когда из-за коронавируса началась пандемия COVID-19. Для строгого контроля за распространением вируса ношение маски является обязательным. В общественных местах нет возможности проверить каждого человека на ношение маски. Предложен способ обнаружения маски на лице человека с помощью методов глубокого обучения и сверточных нейронных сетей (Convolutional Neural Network, CNN). Облачный подход основан на глубоком обучении и используется для выявления лиц, нарушающих правила. Набор данных, примененный в работе, заимствован из научных исследований, таких как Prajnasb/observations и набор данных Kaggle Face Mask Detection. Данные содержат изображения людей в масках и без них. Лица на изображениях обнаруживаются и выделяются с помощью специального обученного детектора лиц. Обнаружение маски осуществлено с помощью детектора и CNN. Входное изображение направляется в сеть, а выходные данные представляются в двоичном формате независимо от того, обнаружена маска или нет. В работе использованы слои CNN Max Pooling и Average Pooling. Результат исследования предложенного метода показал, что метод позволяет достичь 98 % точности на максимальном объеме тестовых изображений.
Ключевые слова: сверточные нейронные сети, CNN, PyTorch, глубокое обучение, облако
Список литературы
Список литературы
-
Nagrath P., Jain R., Madan A., Arora R., Kataria P., Hemanth J. SSDMNV2: A real time DNN-based face mask detection system using single shot multibox detector and MobileNetV2 // Sustainable Cities and Society. 2021. V. 66. P. 102692. https://doi.org/10.1016/j.scs.2020.102692
-
Matrajt L., Leung T. Evaluating the effectiveness of social distancing interventions to delay or flatten the epidemic curve of coronavirus disease // Emerging Infectious Diseases. 2020. V. 26. N 8. P. 1740–1748. https://doi.org/10.3201/eid2608.201093
-
Fu C., Liu W., Ranga A., Tyagi A., Berg A. DSSD: deconvolutional single shot detector model // arXiv. 2017. arXiv:1701.06659. https://doi.org/10.48550/arXiv.1701.06659
-
Lin T.Y., Dollár P., Girshick R., He K., Hariharan B., Belongie S. Future pyramid networks for object detection // Proc. of the 30th IEEE Conference Proceedings on Computer Vision and Pattern Recognition (CVPR). 2017. P. 936–944. https://doi.org/10.1109/CVPR.2017.106
-
Sandler M., Howard A., Zhu M., Zhmoginov A., Chen L.-C. MobileNetV2: Inverted residuals and linear bottlenecks // Proc. of the 31st Meeting of the IEEE/CVF Conference on Computer Vision and Pattern Recognition (CVPR). 2018. P. 4510–4520. https://doi.org/10.1109/CVPR.2018.00474
-
Farfade S.S., Saberian M.J., Li L. Multi-view face detection using deep convolutional neural networks // Proc. of the 5th ACM International Conference on Multimedia Retrieval (ICMR). 2015. P. 643–650. https://doi.org/10.1145/2671188.2749408
-
Chen S., Zhang C., Dong M., Le J., Rao M. Using ranking-CNN for age estimation // Proc. of the 30th IEEE Conference on Computer Vision and Pattern Recognition (CVPR). 2017. P. 742–751. https://doi.org/10.1109/CVPR.2017.86
-
Hinton G., Vinyals O., Dean J. Distilling the knowledge in a neural network // arXiv. 2015. arXiv:1503.02531. https://doi.org/10.48550/arXiv.1503.02531
-
Bandaru A., Bhadani A., Sinha A. A facemask detector using machine learning and image processing techniques // Engineering Science and Technology an International Journal. 2020.
-
Gurav O. Face Mask Detection Dataset. 2020 [Электронный ресурс]. URL: https://www.kaggle.com/omkargurav/face-mask-dataset(дата обращения: 25.12.2021).
-
Ren S., He K., Girshick R., Sun J. Faster R-CNN: Towards real-time object detection with region proposal networks // IEEE Transactions on Pattern Analysis and Machine Intelligence. 2017. V. 39. N 6. P. 1137–1149. https://doi.org/10.1109/TPAMI.2016.2577031.
-
Viola P., Jones M. Rapid object detection using a boosted cascade of simple features // Proc. of the 2001 IEEE Computer Society Conference on Computer Vision and Pattern Recognition (CVPR). V. 1. 2001. P. I511–I518. https://doi.org/10.1109/CVPR.2001.990517
-
Oro D., Fernández C., Saeta J.R., Martorell X., Hernando J. Real-time GPU-based face detection in HD video sequences // Proc. of the IEEE International Conference on Computer Vision Workshops (ICCV). 2011. P. 530–537. https://doi.org/10.1109/ICCVW.2011.6130288
-
Glass R.J., Glass L.M., Beyeler W.E., Min H.J. Targeted social distancing designs for pandemic influenza // Emerging Infectious Diseases. 2006. V. 12. N 11. P. 1671–1681. https://doi.org/10.3201/eid1211.060255
-
Masita K.L., Hasan A.N., Satyakama P. Pedestrian detection using R-CNN object detector // IEEE Latin American Conference on Computational Intelligence (LA-CCI). 2018. P. 8625210. https://doi.org/10.1109/LA-CCI.2018.8625210
-
Girshick R. Fast R-CNN // Proc. of the 15th IEEE International Conference on Computer Vision (ICCV). 2015. P. 1440–1448.https://doi.org/10.1109/ICCV.2015.169
-
Zhu X., Ramanan D. Face detection, pose estimation, and landmark localization in the wild // Proc. of the IEEE Conference on Computer Vision and Pattern Recognition (CVPR). 2012. P. 2879–2886. https://doi.org/10.1109/CVPR.2012.6248014
-
Howard A.G., Zhu M., Chen B., Kalenichenko D., Wang W., Weyand T., Andreetto M., Adam H. MobileNets: Efficient convolutional neural networks for mobile vision applications // arXiv. arXiv:1704.04861. 2017. https://doi.org/10.48550/arXiv.1704.04861
-
Nagrath P., Jain R., Madan A., Arora R., Kataria P., Hemanth J. SSDMNV2: A real time DNN-based face mask detection system using single shot multibox detector and MobileNetV2 // Sustainable Cities and Society. 2021. V. 66. P. 102692. https://doi.org/10.1016/j.scs.2020.102692
-
Goodfellow I., Bengio Y., Courville A. Deep Learning. MIT Press, 2016. 800 p.
-
Jiang M., Fan X., Yan H. Retina Mask: A Face Mask detector // arXiv. 2020. arXiv:2005.03950v2. https://doi.org/10.48550/arXiv.2005.03950
-
Jiang X., Gao T., Zhu Z., Zhao Y. Real-Time Face Mask Detection Method Based on YOLOv3 // Electronics. 2021. V. 10. N 7. P. 837. https://doi.org/10.3390/electronics10070837
-
Liu S., Again S.S. COVID-19 face mask detection in a crowd using multi-model based on YOLOv3 and hand-crafted features // Proceedings of SPIE. 2021. V. 11734. P. 117340–117340. https://doi.org/10.1117/12.2586984
-
Sen S., Sawant K. Face mask detection for covid_19 pandemic using pytorch in deep learning // IOP Conference Series: Materials Science and Engineering. 2021. V. 1070. P. 012061. https://doi.org/10.1088/1757-899X/1070/1/012061
-
Sethi S., Kathuria M., Kaushik T. A real-time integrated face mask detector to curtail spread of coronavirus // Computer Modeling in Engineering & Sciences. 2021. V. 127. N. 2. P. 389–409. https://doi.org/1032604/cmes.2021.014478
-
Srivastava P., Khan R. A review paper on cloud computing // International Journal of Advanced Research in Computer Science and Software Engineering. 2018. V. 8. N 6. P. 17. https://doi.org/10.23956/ijarcsse.v8i6.711
-
Susanto S., Putra F.A., Analia R., Suciningtyas I.K.L.N. The face mask detection for preventing the spread of COVID-19 at Politeknik Negeri Batam // Proc. of the 3rd International Conference on Applied Engineering (ICAE). 2020. P. 9350556. https://doi.org/10.1109/ICAE50557.2020.9350556
-
Wang Z., Wang G., Huang B., Xiong Z., Hong Q., Wu H., Yi P., Jiang K., Wang N., Pei Y., Chen H., Miao Y., Huang Z., Liang J. Masked face recognition dataset and application // arXiv. 2020. arXiv:2003.09093v2. https://doi.org/10.48550/arXiv.2003.09093