Меню
Публикации
2025
2024
2023
2022
2021
2020
2019
2018
2017
2016
2015
2014
2013
2012
2011
2010
2009
2008
2007
2006
2005
2004
2003
2002
2001
Главный редактор
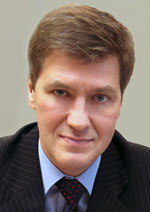
НИКИФОРОВ
Владимир Олегович
д.т.н., профессор
Партнеры
doi: 10.17586/2226-1494-2022-22-5-982-991
УДК 004.052.42
Генерация слабейших предусловий программ с динамической памятью в символьном исполнении
Читать статью полностью

Язык статьи - русский
Ссылка для цитирования:
Аннотация
Ссылка для цитирования:
Мисонижник А.В., Костюков Ю.О., Костицын М.П., Мордвинов Д.А., Кознов Д.В. Генерация слабейших предусловий программ с динамической памятью в символьном исполнении // Научно-технический вестник информационных технологий, механики и оптики. 2022. Т. 22, № 5. С. 982–991. doi: 10.17586/2226-1494-2022-22-5-982-991
Аннотация
Предмет исследования. Символьное исполнение — широко известный метод систематического исследования путей исполнения программ. Метод позволяет решить ряд важных задач, связанных с контролем корректности: поиск ошибок и уязвимостей, автоматическая генерация тестов и др. Основная идея символьного исполнения — порождение и использование символьных логических выражений при анализе программ в прямом порядке, т. е. от входной точки к точкам интереса. Хорошо известен метод обратного символьного исполнения, когда условия попадания в точку интереса распространяются ко входной точке программы за счет итеративного вычисления слабейших предусловий. Реализовать этот метод, как правило, намного труднее, чем прямое символьное исполнение, так что даже артефакты последнего не удается использовать при реализации. Метод. Исследована связь между прямым и обратным символьными исполнениями на основе вычисления слабейших предусловий. В частности показано, как обратное исполнение может быть реализовано на базе прямого. Основные результаты. Приведено формальное описание процедуры символьного исполнения с ленивой инициализацией для программ с динамической памятью. Предложен алгоритм вычисления слабейших предусловий для произвольных ветвей исполнения программы. Механизм ленивой инициализации и алгоритм вычисления слабейших предусловий реализованы в символьной виртуальной машине KLEE, работающей на базе широко известной платформы LLVM. Практическая значимость. Представленный метод позволяет выполнять обратный символьный анализ при помощи прямого символьного исполнения. Это важно для реализации двунаправленного исполнения программ, которое может быть применено для верификации программ и автоматического порождения тестовых покрытий.
Ключевые слова: слабейшие предусловия, символьное исполнение, обратный символьный анализ, двунаправленный анализ, автоматическая генерация тестов
Благодарности. Данное исследование было поддержано грантом РНФ № 22-21-00697.
Список литературы
Благодарности. Данное исследование было поддержано грантом РНФ № 22-21-00697.
Список литературы
-
Baldoni R., Coppa E., D'Elia D.C., Demetrescu C., Finocchi I. A survey of symbolic execution techniques // ACM Computing Surveys. 2018. V. 51. N 3. https://doi.org/10.1145/3182657
-
Cadar C., Dunbar D., Engler D. KLEE: unassisted and automatic generation of high-coverage tests for complex systems programs // Proc. of the 8th USENIX Conference on Operating Systems Design and Implementation. 2008. P. 209–224.
-
Wang F., Shoshitaishvili Y. ANGR – the next generation of binary analysis // Proc. of the IEEE Cybersecurity Development (SecDev). 2017. P. 8–9. https://doi.org/10.1109/SecDev.2017.14
-
Burnim J., Sen K. Heuristics for scalable dynamic test generation // Proc. of the ASE 2008 - 23rd IEEE/ACM International Conference on Automated Software Engineering. 2008. P. 443–446. https://doi.org/10.1109/ASE.2008.69
-
Tillmann N., Halleux J. Pex-white box test generation for .NET // Lecture Notes in Computer Science (including subseries Lecture Notes in Artificial Intelligence and Lecture Notes in Bioinformatics). 2008. V. 4966. P. 134–153. https://doi.org/10.1007/978-3-540-79124-9_10
-
Pǎsǎreanu C.S., Rungta N. Symbolic PathFinder: symbolic execution of Java bytecode // Proc. of the 25th IEEE/ACM International Conference on Automated Software Engineering. 2010. P. 179–180. https://doi.org/10.1145/1858996.1859035
-
Mossberg M., Manzano F., Hennenfent E., Groce A., Grieco G., Feist J., Brunson T., Dinaburg A. Manticore: A user-friendly symbolic execution framework for binaries and smart contracts // Proc. of the 34th IEEE/ACM International Conference on Automated Software Engineering (ASE). 2019. P. 1186–1189. https://doi.org/10.1109/ASE.2019.00133
-
Godefroid P., Klarlund N., Sen K. DART: Directed automated random testing // Proc. of the 2005 ACM SIGPLAN conference on Programming Language Design and Implementation (PLDI). 2005. P. 213–223.
-
Godefroid P., Levin M.Y., Molnar D. SAGE: whitebox fuzzing for security testing // Communications of the ACM. 2012. V. 55. N 3. P. 40–44. https://doi.org/10.1145/2093548.2093564
-
Chipounov V., Kuznetsov V., Candea G. S2E: a platform for in-vivo multi-path analysis of software systems // ACM SIGPLAN Notices. 2011. V. 46. N 3. P. 265–278. https://doi.org/10.1145/1961296.1950396
-
Vishnyakov A., Fedotov A., Kuts D., Novikov A., Parygina D., Kobrin E., Logunova V., Belecky P., Kurmangaleev S. Sydr: Cutting edge dynamic symbolic execution // Proc. of the 2020 Ivannikov Ispras Open Conference (ISPRAS). 2020. P. 46–54. https://doi.org/10.1109/ISPRAS51486.2020.00014
-
Dinges P., Agha G. Targeted test input generation using symbolic-concrete backward execution // Proc. of the 29th ACM/IEEE International Conference on Automated Software Engineering. 2014. P. 31–36. https://doi.org/10.1145/2642937.2642951
-
Chandra S., Fink S.J., Sridharan M. Snugglebug: a powerful approach to weakest preconditions // ACM SIGPLAN Notices. 2009. V. 44. N 6. P. 363–374. https://doi.org/10.1145/1543135.1542517
-
Dijkstra E.W. A Discipline of Programming. Englewood Cliffs, N.J.: Prentice-Hall, 1976.XVII, 217 p.
-
Khurshid S., Pǎsǎreanu C.S., Visser W. Generalized symbolic execution for model checking and testing // Lecture Notes in Computer Science (including subseries Lecture Notes in Artificial Intelligence and Lecture Notes in Bioinformatics). 2003. P. 553–568. https://doi.org/10.1007/3-540-36577-X_40
-
Anand S., Pǎsǎreanu C.S., Visser W. Symbolic execution with abstraction // International Journal on Software Tools for Technology Transfer. 2009. V. 11. N 1. P. 53–67. https://doi.org/10.1007/s10009-008-0090-1
-
Lin Y. Symbolic execution with over-approximation: Ph.D. Thesis. The University of Melbourne, 2017.
-
Rungta N., Mercer E.G., Visser W. Efficient testing of concurrent programs with abstraction-guided symbolic execution // Lecture Notes in Computer Science (including subseries Lecture Notes in Artificial Intelligence and Lecture Notes in Bioinformatics). 2009. V. 5578. P. 174–191. https://doi.org/10.1007/978-3-642-02652-2_16
-
Berdine J., Calcagno C., O'Hearn P.W. Symbolic execution with separation logic // Lecture Notes in Computer Science (including subseries Lecture Notes in Artificial Intelligence and Lecture Notes in Bioinformatics). 2005. V. 3780. P. 52–68. https://doi.org/10.1007/11575467_5
-
Yi Q., Yang Z., Guo S., Wang C., Liu J., Zhao C. Postconditioned symbolic execution // Proc. of the 8th IEEE International Conference on Software Testing, Verification and Validation (ICST). 2015. P. 1–10. https://doi.org/10.1109/ICST.2015.7102601
-
McMillan K.L. Lazy annotation for program testing and verification // Lecture Notes in Computer Science (including subseries Lecture Notes in Artificial Intelligence and Lecture Notes in Bioinformatics). 2010. V. 6174. P. 104–118. https://doi.org/10.1007/978-3-642-14295-6_10
-
Deng X., Lee J., Robby. Efficient and formal generalized symbolic execution // Automated Software Engineering. 2012. V. 19. N 3. P. 233–301. https://doi.org/10.1007/s10515-011-0089-9
-
Hoare C.A.R. An axiomatic basis for computer programming // Communications of the ACM. 1969. V. 12. N 10. P. 576–580. https://doi.org/10.1145/363235.363259
-
Nepomniaschy V.A., Anureev I.S., Promskii A.V. Towards verification of C programs: axiomatic semantics of the C-kernel language // Programming and Computer Software. 2003. V. 29. N 6. P. 338–350. https://doi.org/10.1023/B:PACS.0000004134.24714.e5
-
Blazy S., Leroy X. Mechanized semantics for the Clight subset of the C language // Journal of Automated Reasoning. 2009. V. 43. N 3. P. 263–288. https://doi.org/10.1007/s10817-009-9148-3
-
De Moura L., Bjørner N. Z3: an efficient SMT solver // Lecture Notes in Computer Science (including subseries Lecture Notes in Artificial Intelligence and Lecture Notes in Bioinformatics). 2008. V. 4963. P. 337–340. https://doi.org/10.1007/978-3-540-78800-3_24
-
Ball T., Daniel J. Deconstructing dynamic symbolic execution // Proc. of the 2014 Marktoberdorf Summer School on Dependable Software Systems Engineering. IOS Press, 2015.