Меню
Публикации
2024
2023
2022
2021
2020
2019
2018
2017
2016
2015
2014
2013
2012
2011
2010
2009
2008
2007
2006
2005
2004
2003
2002
2001
Главный редактор
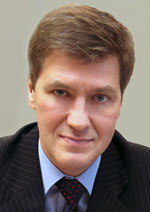
НИКИФОРОВ
Владимир Олегович
д.т.н., профессор
Партнеры
doi: 10.17586/2226-1494-2023-23-2-352-363
УДК 004.056.5
Обзор систем обнаружения сетевых вторжений, основанных на подходах глубокого обучения
Читать статью полностью

Язык статьи - английский
Ссылка для цитирования:
Аннотация
Ссылка для цитирования:
Аль-Сафар Д.В., Аль-Ясин В.Л. Обзор систем обнаружения сетевых вторжений, основанных на подходах глубокого обучения // Научно-технический вестник информационных технологий, механики и оптики. 2023. Т. 23, № 2. С. 352–363 (на англ. яз.). doi: 10.17586/2226-1494-2023-23-2-352-363
Аннотация
В настоящее время большинство ИТ-организаций отдают предпочтение среде облачных вычислений, которая имеет распределенный и масштабируемый характер. При этом гибкая и открытая архитектура среды облачных вычислений привлекает большое внимание потенциальных злоумышленников из-за киберугроз. В данном случае система обнаружения вторжений (Intrusion Detection System, IDS) играет важную роль в отслеживании вредоносных действий в облачных системах. В работе представлен системный обзор существующих IDS, основанных на различных методах, таких как интеллектуальный анализ данных, машинное обучение и методы глубокого обучения. В последнее время методы глубокого обучения широко распространены в области обнаружения вторжений при решении проблем конфиденциальности и угроз безопасности. В связи с этим важно исследовать подходы к исследованию глубокого обучения, применяемых на разных этапах процесса обнаружения вторжений. Выполнено сравнение подходов глубокого обучения и поверхностных методов машинного обучения. Приведено описание наборов данных, наиболее часто используемых в системах обнаружения вторжений.
Ключевые слова: облачные вычисления, система обнаружения вторжений, машинное обучение, глубокое обучение
Список литературы
Список литературы
-
Deshpande P., Sharma S.C., Peddoju S.K., Junaid S. HIDS: A host based intrusion detection system for cloud computing environment // International Journal of System Assurance Engineering and Management. 2018. V. 9. N 3. P. 567–576. https://doi.org/10.1007/s13198-014-0277-7
-
Shamshirband S., Fathi M., Chronopoulos A.T., Montieri A., Palumbo F., Pescapè A. Computational intelligence intrusion detection techniques in mobile cloud computing environments: Review, taxonomy, and open research issues // Journal of Information Security and Applications. 2020. V. 55. P. 102582. https://doi.org/10.1016/j.jisa.2020.102582
-
Aldweesha A., Derhabb A., Emamc A.Z. Deep learning approaches for anomaly-based intrusion detection systems: A Survey, taxonomy, and open issues // Knowledge-Based Systems. 2020. V. 189. P. 105124. https://doi.org/10.1016/j.knosys.2019.105124
-
AbdAllah E.G., Zulkernine M., Hassanein H.S. Preventing unauthorized access in information centric networking // Security and Privacy. 2018. V. 1. N 4. P. e33. https://doi.org/10.1002/spy2.33
-
Ahmad Z., Khan A.S., Shiang C.W., Abdullah J., Ahmad F.Network intrusion detection system: A systematic study of machine learning and deep learning approaches // Transactions on Emerging Telecommunications Technologies. 2021. V. 32. N 1. P. e4150. https://doi.org/10.1002/ett.4150
-
Tun H., Lupin S., Linn H.H., Lin K.N.Z. Selection the perimeter protection equipment in security systems // Proc. of the IEEE Conference of Russian Young Researchers in Electrical and Electronic Engineering (EIConRus). 2018. P. 1504–1508. https://doi.org/10.1109/eiconrus.2018.8317383
-
Saxena A., Mueller C. Intelligent intrusion detection in computer networks using swarm intelligence // International Journal of Computer Applications. 2018. V. 179. N 16. P. 1–9. https://doi.org/10.5120/ijca2018916224
-
Liu G., Zhang J. CNID: Research of network intrusion detection based on convolutional neural network // Discrete Dynamics in Nature and Society. 2020. V. 2020. P. 1–11. https://doi.org/10.1155/2020/4705982
-
Wu Y., Wei D., Feng J. Network attacks detection methods based on deep learning techniques: A survey // Security and Communication Networks. 2020. V. 2020. P. 1–17. https://doi.org/10.1155/2020/8872923
-
Vincent P., Larochelle H., Bengio Y., Manzagol P.-A. Extracting and composing robust features with Denoising autoencoders // Proceedings of the 25th International Conference on Machine learning (ICML). P. 1096–1103. https://doi.org/10.1145/1390156.1390294
-
Vincent P., Larochelle H., Lajoie I., Bengio Y., Manzagol P.A. Stacked denoising autoencoders: Learning useful representations in a deep network with a local denoising criterion // Journal of Machine Learning Research. 2010. V. 11. P. 3371–3408.
-
Deng J., Zhang Z., Marchi E., Schuller B. Sparse autoencoder-based feature transfer learning for speech emotion recognition // Proc. of the 2013 Humaine Association Conference on Affective Computing and Intelligent Interaction. 2013. P. 511–516. https://doi.org/10.1109/acii.2013.90
-
Yan B., Han G. Effective feature extraction via stacked sparse autoencoder to improve intrusion detection system // IEEE Access. 2018. V. 6. P. 41238–41248. https://doi.org/10.1109/access.2018.2858277
-
Farahnakian F., Heikkonen J. A deep auto-encoder based approach for intrusion detection system // Proc. of the 20th International Conference on Advanced Communication Technology (ICACT). 2018. P. 178–183. https://doi.org/10.23919/icact.2018.8323688
-
Shone N., Ngoc T.N. A deep learning approach to network intrusion detection // IEEE Transactions on Emerging Topics in Computational Intelligence. 2018. V. 2. N 1. P. 41–50. https://doi.org/10.1109/tetci.2017.2772792
-
Zhang C., Ruan F., Yin L., Chen X., Zhai L., Liu F. A deep learning approach for network intrusion detection based on NSL-KDD dataset // Proc. of the IEEE 13th International Conference on Anti-counterfeiting, Security, and Identification (ASID). 2019. P. 41–45. https://doi.org/10.1109/icasid.2019.8925239
-
Al-Qatf M., Lasheng Y., Al-Habib M., Al-Sabahi K. Deep learning approach combining sparse autoencoder with SVM for network intrusion detection // IEEE Access. 2018. V. 6. P. 2169–3536. https://doi.org/10.1109/access.2018.2869577
-
Zhang Y., Zhang Y., Zhang N., Xiao M. A network intrusion detection method based on deep learning with higher accuracy // Procedia Computer Science. 2020. V. 174. P. 50–54. https://doi.org/10.1016/j.procs.2020.06.055
-
Hinton G.E., Osindero S., Teh Y.-W. A fast learning algorithm for deep belief nets // Neural Computation. 2006. V. 18. N 7. P. 1527–1554. https://doi.org/10.1162/neco.2006.18.7.152
-
Ranzato M.A., Boureau Y.l., Cun Y.L. Sparse feature learning for deep belief networks // Proc. of the 21st Annual Conference on Neural Information Processing Systems (NIPS). 2008. P. 1185–1192.
-
Gao N., Gao L., Gao Q., Wang H. An intrusion detection model based on deep belief networks // Proc. of the Second International Conference on Advanced Cloud and Big Data. 2014. P. 247–252. https://doi.org/10.1109/cbd.2014.41
-
Coli G.O., Aina S., Okegbile S.D., LawalA.R., Oluwaranti A.I. DDoS attacks detection in the IoT using deep gaussian-bernoulli restricted boltzmann machine // Modern Applied Science. 2022. V. 16. N 2. P. 12. https://doi.org/10.5539/mas.v16n2p12
-
Erpek T., Sagduyu Y.E., Shi Y. Deep learning for launching and mitigating wireless jamming attacks // IEEE Transactions on Cognitive Communications and Networking. 2019. V. 5. N 1. P. 2–14. https://doi.org/10.1109/tccn.2018.2884910
-
Tang C., Luktarhan N., Zhao Y. SAAE-DNN: deep learning method on intrusion detection // Symmetry. 2020. V. 12. N 10. P. 1695. https://doi.org/10.3390/sym12101695
-
Naseer S., Saleem Y., Khalid S., Bashir M.K., Han J., Iqbal M.M., Han K. Enhanced network anomaly detection based on deep neural networks // IEEE Access. 2018. V. 6. P. 48231–48246. https://doi.org/10.1109/access.2018.2863036
-
Liu G., Zhang J. CNID: Research of network intrusion detection based on convolutional neural network // Discrete Dynamics in Nature and Society. 2020. V. 2020. P. 1–11. https://doi.org/10.1155/2020/4705982
-
Al-Emadi S., Al-Mohannadi A., Al-Senaid F. Using deep learning techniques for network intrusion detection // IEEE International Conference on Informatics, IoT, and Enabling Technologies (ICIoT). 2020. P. 171–176.https://doi.org/10.1109/iciot48696.2020.9089524
-
Graves A., Mohamed A.R., Hinton G. Speech recognition with deep recurrent neural networks // Proc. of the 2013 IEEE International Conference on Acoustics, Speech and Signal Processing. 2013. P. 6645–6649. https://doi.org/10.1109/icassp.2013v.6638947
-
Sutskever O., Vinyals Q.V., Le Q.V. Sequence to sequence learning with neural networks // Proc. of the 27th International Conference on Neural Information Processing Systems (NIPS'14). 2014. P. 3104–3112.
-
Thilagam T., Aruna R. Intrusion detection for network based cloud computing by custom RC-NN and optimization // ICT Express. 2021. V. 2. N 4. P. 512–520. https://doi.org/10.1016/j.icte.2021.04.006
-
Prabhakaran V., Kulandasamy A. Hybrid semantic deep learning architecture and optimal advanced encryption standard key management scheme for secure cloud storage and intrusion detection // Neural Computing and Applications. 2021. V. 33. N 21. P. 14459–14479. https://doi.org/10.1007/s00521-021-06085-5
-
Al-Yaseen W.L. Multiagent System for an Adaptive Real Time Intrusion Detection System. LAP Lambert Academic Publishing, 2016. 272 p.