Меню
Публикации
2024
2023
2022
2021
2020
2019
2018
2017
2016
2015
2014
2013
2012
2011
2010
2009
2008
2007
2006
2005
2004
2003
2002
2001
Главный редактор
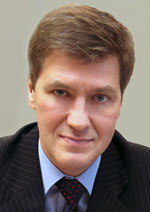
НИКИФОРОВ
Владимир Олегович
д.т.н., профессор
Партнеры
doi: 10.17586/2226-1494-2023-23-3-506-518
УДК 004.032.26
Детекция ключевых точек лица с помощью капсульных нейронных сетей
Читать статью полностью

Язык статьи - русский
Ссылка для цитирования:
Аннотация
Ссылка для цитирования:
Бойцев А.А., Волчек Д.Г., Магазенков Е.Н., Неваев М.К., Романов А.А. Детекция ключевых точек лица с помощью капсульных нейронных сетей // Научно-технический вестник информационных технологий, механики и оптики. 2023. Т. 23, № 3. С. 506–518. doi: 10.17586/2226-1494-2023-23-3-506-518
Аннотация
Введение. Исследована актуальная и востребованная задача детекции ключевых точек лица. Рассмотрены существующие подходы к решению задачи детекции ключевых точек лица, часто классифицируемые на параметрические и непараметрические. Определен наиболее качественный на сегодняшний день подход, основанный на методах глубокого обучения. Предложено два решения: капсульная сеть с динамической маршрутизацией и глубокая капсульная сеть. В качестве данных для проведения эксперимента выбраны 10 000 сгенерированных лиц из базы сайта Kaggle, размеченных с помощью фреймворка MediaPipe. Метод. Предложен метод использования капсульных архитектур нейронных сетей для решения задачи детекции ключевых точек лица. Метод включает в себя использование сегментации по распознанным с помощью фреймворка MediaPipe ключевым точкам лица. Для построения сетки лица применена триангуляция Делоне. Предложена архитектура глубокой капсульной сети с учетом семантической сегментации. Основные результаты. На основе размеченных данных выполнены эксперименты по детекции ключевых точек с помощью разработанных капсульных нейронных сетей. По результатам тестирования получены значения функции потерь 2,5–2,9 и точности 0,87–0,9. Обсуждение. Предложенная архитектура может быть использована в технологиях по сопоставлению геометрий сеток лица реального человека и трехмерной модели. Архитектура может найти применение в исследованиях капсульных нейронных сетей в области обработки и анализа изображений.
Ключевые слова: капсульные нейронные сети, детекция ключевых точек лица, распознавание изображений лиц, нейросети
Список литературы
Список литературы
1. Волкова С.С., Матвеев Ю.Н. Применение сверточных нейронных сетей для решения задачи противодействия атаке спуфинга в системах лицевой биометрии // Научно-технический вестник информационных технологий, механики и оптики. 2017. Т. 17. № 4. С. 702–710. https://doi.org/10.17586/2226-1494-2017-17-4-702-710
2. Дикий Д.И., Артемьева В.Д. Исследование применимости искусственных нейронных сетей для верификации пользователей по динамике почерка // Научно-технический вестник информационных технологий, механики и оптики. 2017. Т. 17. № 4. С. 677–684. https://doi.org/10.17586/2226-1494-2017-17-4-677-684
3. Abiodun O.I., Kiru M.U., Jantan A., Omolara A.E., Dada K.V., Umar A.M., Linus O.U., Arshad H., Kazaure A.A., Gana U. Comprehensive review of artificial neural network applications to pattern recognition // IEEE Access. 2019. V. 7. P. 158820–158846. https://doi.org/10.1109/access.2019.2945545
4. Krizhevsky A., Sutskever I., Hinton G.E. ImageNet classification with deep convolutional neural networks // Communications of the ACM. 2017. V. 60. N 6. P. 84–90. https://doi.org/10.1145/3065386
5. He K., Zhang X., Ren S., Sun J. Deep residual learning for image recognition // Proc. of the IEEE Conference on Computer Vision and Pattern Recognition (CVPR). 2016. P. 770–778. https://doi.org/10.1109/cvpr.2016.90
6. Redmon J., Divvala S., Girshick R., Farhadi A. You only look once: Unified, real-time object detection // Proc. of the IEEE Conference on Computer Vision and Pattern Recognition (CVPR). 2016. P. 779–788. https://doi.org/10.1109/cvpr.2016.91
7. Sabour S., Frosst N., Hinton G.E. Dynamic routing between capsules // Advances in Neural Information Processing Systems. 2017. V. 30. P. 3856–3866.
8. Nguyen H.H., Yamagishi J., Echizen I. Capsule-forensics: Using capsule networks to detect forged images and videos // Proc. of the ICASSP 2019-2019 IEEE International Conference on Acoustics, Speech and Signal Processing (ICASSP). 2019. P. 2307–2311. https://doi.org/10.1109/icassp.2019.8682602
9. Wang N., Gao X., Tao D., Yang H., Li X. Facial feature point detection: A comprehensive survey // Neurocomputing. 2018. V. 275. P. 50–65. https://doi.org/10.1016/j.neucom.2017.05.013
10. Beham M.P., Roomi S.M.M. A review of face recognition methods // International Journal of Pattern Recognition and Artificial Intelligence. 2013. V. 27. N 4. P. 1356005. https://doi.org/10.1142/S0218001413560053
11. Li H., Lin Z.L., Brandt J., Shen X., Hua G. Efficient boosted exemplar-based face detection // Proc. of the 2014 IEEE Conference on Computer Vision and Pattern Recognition. 2014. P. 1843–1850. https://doi.org/10.1109/cvpr.2014.238
12. Sun Y., Wang X., Tang X. Deep convolutional network cascade for facial point detection // Proc. of the IEEE Conference on Computer Vision and Pattern Recognition. 2013. P. 3476–3483. https://doi.org/10.1109/cvpr.2013.446
13. Zhang Z., Luo P., Loy C.C., Tang X. Facial landmark detection by deep multi-task learning // Lecture Notes in Computer Science. 2014. V. 8694. P. 94–108. https://doi.org/10.1007/978-3-319-10599-4_7
14. Trigeorgis G., Snape P., Nicolaou M.A., Antonakos E., Zafeiriou S. Mnemonic descent method: A recurrent process applied for end-to-end face alignment // Proc. of the IEEE Conference on Computer Vision and Pattern Recognition (CVPR). 2016. P. 4177–4187. https://doi.org/10.1109/cvpr.2016.453
15. Zhu X., Lei Z., Liu X., Shi H., Li S.Z. Face alignment across large poses: A 3D solution // Proc. of the IEEE Conference on Computer Vision and Pattern Recognition (CVPR). 2016. P. 146–155. https://doi.org/10.1109/cvpr.2016.23
16. Jourabloo A., Liu X. Large-pose face alignment via CNN-based dense 3D model fitting // Proc. of the IEEE Conference on Computer Vision and Pattern Recognition (CVPR). 2016. P. 4188–4196. https://doi.org/10.1109/cvpr.2016.454
17. Kartynnik Y., Ablavatski A., Grishchenko I., Grundmann M. Real-time facial surface geometry from monocular video on mobile GPUs // arXiv. 2019. arXiv:1907.06724. https://doi.org/10.48550/arXiv.1907.06724
18. Wood E., Baltrušaitis T., Hewitt Ch., Johnson M., Shen J., Milosavljević N., Wilde D., Garbin S., Sharp T., Stojiljković I., Cashman T., Valentin J. 3D face reconstruction with dense landmarks // Lecture Notes in Computer Science. 2022. V. 13673. P. 160–177. https://doi.org/10.1007/978-3-031-19778-9_10
19. Pechyonkin M. Understanding Hinton’s Capsule Networks. Part I: Intuition. Medium. 2018, December 18 [Электронный ресурс]. URL: https://medium.com/ai%C2%B3-theory-practice-business/understanding-hintons-capsule-networks-part-i-intuition-b4b559d1159b (дата обращения: 12.12.2022).
20. Yu D., Wang H., Chen P., Wei Z. Mixed Pooling for convolutional neural networks // Lecture Notes in Computer Science. 2014. V. 8818. P. 364–375. https://doi.org/10.1007/978-3-319-11740-9_34
21. Ding Y., Chen F., Zhao Y., Wu Z., Zhang C., Wu D. A stacked multi-connection simple reducing net for brain tumor segmentation // IEEE Access. 2019. V. 7. P. 104011–104024. https://doi.org/10.1109/access.2019.2926448
2. Дикий Д.И., Артемьева В.Д. Исследование применимости искусственных нейронных сетей для верификации пользователей по динамике почерка // Научно-технический вестник информационных технологий, механики и оптики. 2017. Т. 17. № 4. С. 677–684. https://doi.org/10.17586/2226-1494-2017-17-4-677-684
3. Abiodun O.I., Kiru M.U., Jantan A., Omolara A.E., Dada K.V., Umar A.M., Linus O.U., Arshad H., Kazaure A.A., Gana U. Comprehensive review of artificial neural network applications to pattern recognition // IEEE Access. 2019. V. 7. P. 158820–158846. https://doi.org/10.1109/access.2019.2945545
4. Krizhevsky A., Sutskever I., Hinton G.E. ImageNet classification with deep convolutional neural networks // Communications of the ACM. 2017. V. 60. N 6. P. 84–90. https://doi.org/10.1145/3065386
5. He K., Zhang X., Ren S., Sun J. Deep residual learning for image recognition // Proc. of the IEEE Conference on Computer Vision and Pattern Recognition (CVPR). 2016. P. 770–778. https://doi.org/10.1109/cvpr.2016.90
6. Redmon J., Divvala S., Girshick R., Farhadi A. You only look once: Unified, real-time object detection // Proc. of the IEEE Conference on Computer Vision and Pattern Recognition (CVPR). 2016. P. 779–788. https://doi.org/10.1109/cvpr.2016.91
7. Sabour S., Frosst N., Hinton G.E. Dynamic routing between capsules // Advances in Neural Information Processing Systems. 2017. V. 30. P. 3856–3866.
8. Nguyen H.H., Yamagishi J., Echizen I. Capsule-forensics: Using capsule networks to detect forged images and videos // Proc. of the ICASSP 2019-2019 IEEE International Conference on Acoustics, Speech and Signal Processing (ICASSP). 2019. P. 2307–2311. https://doi.org/10.1109/icassp.2019.8682602
9. Wang N., Gao X., Tao D., Yang H., Li X. Facial feature point detection: A comprehensive survey // Neurocomputing. 2018. V. 275. P. 50–65. https://doi.org/10.1016/j.neucom.2017.05.013
10. Beham M.P., Roomi S.M.M. A review of face recognition methods // International Journal of Pattern Recognition and Artificial Intelligence. 2013. V. 27. N 4. P. 1356005. https://doi.org/10.1142/S0218001413560053
11. Li H., Lin Z.L., Brandt J., Shen X., Hua G. Efficient boosted exemplar-based face detection // Proc. of the 2014 IEEE Conference on Computer Vision and Pattern Recognition. 2014. P. 1843–1850. https://doi.org/10.1109/cvpr.2014.238
12. Sun Y., Wang X., Tang X. Deep convolutional network cascade for facial point detection // Proc. of the IEEE Conference on Computer Vision and Pattern Recognition. 2013. P. 3476–3483. https://doi.org/10.1109/cvpr.2013.446
13. Zhang Z., Luo P., Loy C.C., Tang X. Facial landmark detection by deep multi-task learning // Lecture Notes in Computer Science. 2014. V. 8694. P. 94–108. https://doi.org/10.1007/978-3-319-10599-4_7
14. Trigeorgis G., Snape P., Nicolaou M.A., Antonakos E., Zafeiriou S. Mnemonic descent method: A recurrent process applied for end-to-end face alignment // Proc. of the IEEE Conference on Computer Vision and Pattern Recognition (CVPR). 2016. P. 4177–4187. https://doi.org/10.1109/cvpr.2016.453
15. Zhu X., Lei Z., Liu X., Shi H., Li S.Z. Face alignment across large poses: A 3D solution // Proc. of the IEEE Conference on Computer Vision and Pattern Recognition (CVPR). 2016. P. 146–155. https://doi.org/10.1109/cvpr.2016.23
16. Jourabloo A., Liu X. Large-pose face alignment via CNN-based dense 3D model fitting // Proc. of the IEEE Conference on Computer Vision and Pattern Recognition (CVPR). 2016. P. 4188–4196. https://doi.org/10.1109/cvpr.2016.454
17. Kartynnik Y., Ablavatski A., Grishchenko I., Grundmann M. Real-time facial surface geometry from monocular video on mobile GPUs // arXiv. 2019. arXiv:1907.06724. https://doi.org/10.48550/arXiv.1907.06724
18. Wood E., Baltrušaitis T., Hewitt Ch., Johnson M., Shen J., Milosavljević N., Wilde D., Garbin S., Sharp T., Stojiljković I., Cashman T., Valentin J. 3D face reconstruction with dense landmarks // Lecture Notes in Computer Science. 2022. V. 13673. P. 160–177. https://doi.org/10.1007/978-3-031-19778-9_10
19. Pechyonkin M. Understanding Hinton’s Capsule Networks. Part I: Intuition. Medium. 2018, December 18 [Электронный ресурс]. URL: https://medium.com/ai%C2%B3-theory-practice-business/understanding-hintons-capsule-networks-part-i-intuition-b4b559d1159b (дата обращения: 12.12.2022).
20. Yu D., Wang H., Chen P., Wei Z. Mixed Pooling for convolutional neural networks // Lecture Notes in Computer Science. 2014. V. 8818. P. 364–375. https://doi.org/10.1007/978-3-319-11740-9_34
21. Ding Y., Chen F., Zhao Y., Wu Z., Zhang C., Wu D. A stacked multi-connection simple reducing net for brain tumor segmentation // IEEE Access. 2019. V. 7. P. 104011–104024. https://doi.org/10.1109/access.2019.2926448