Меню
Публикации
2025
2024
2023
2022
2021
2020
2019
2018
2017
2016
2015
2014
2013
2012
2011
2010
2009
2008
2007
2006
2005
2004
2003
2002
2001
Главный редактор
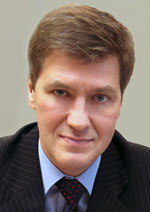
НИКИФОРОВ
Владимир Олегович
д.т.н., профессор
Партнеры
doi: 10.17586/2226-1494-2023-23-3-595-607
УДК УДК 004.822:616-07
Интеллектуальная поддержка клинических решений при небольших выборках числа пациентов
Читать статью полностью

Язык статьи - русский
Ссылка для цитирования:
Аннотация
Ссылка для цитирования:
Ватьян А.С., Голубев А.А., Гусарова Н.Ф., Добренко Н.В., Зубаненко А.А., Кустова Е.С., Татаринова А.А., Томилов И.В., Шовкопляс Г.Ф. Интеллектуальная поддержка клинических решений при небольших выборках числа пациентов // Научно-технический вестник информационных технологий, механики и оптики. 2023. Т. 23, № 3. С. 595–607. doi: 10.17586/2226-1494-2023-23-3-595-607
Аннотация
Введение. Рассмотрены пути обоснования клинического решения врачей в условиях отсутствия клинических протоколов лечения. Метод. Выполнена сравнительная оценка статистических методов ранжирования клинических симптомов по степени значимости для прогнозирования исхода заболевания в условиях небольшой выборки числа пациентов с COVID-19 и сердечно-сосудистыми заболеваниями в анамнезе. Набор данных (141 пациент, 81 фактор) сформирован по материалам электронных медицинских карт пациентов ФГБУ «Национальный медицинский исследовательский центр имени В.А. Алмазова». Выделен поднабор контролируемых факторов риска (51 фактор). Для ранжирования факторов использованы методы дескриптивной статистики (однофакторный дисперсионный анализ, тесты Манна-Уитни и χ²) и методы снижения размерности (одномерная линейная регрессия в сочетании с множественной логистической регрессией, обобщенный дискриминантный анализ, а также различные варианты алгоритмов дерева решений). Для сравнения результатов ранжирования и оценки статистической устойчивости применена корреляция Кендалла, визуализированная в виде тепловой карты и позиционного графика. Основные результаты. Установлено, что использование методов дескриптивной статистики правомерно при ранжировании на небольших размерах выборки пациентов. Показано, что ансамблирование результатов ранжирования может оказаться статистически несостоятельным. Сделан вывод, что позиции одних и тех же признаков, полученных при ранжировании их в составе полного набора и поднабора признаков, не совпадают, поэтому при выборе метода статистической обработки для экспертной оценки следует учитывать содержательную постановку задачи. Показано, что статистическая устойчивость ранжирования в условиях малых выборок зависит от количества учитываемых признаков, и эта зависимость существенно отличается для разных методов ранжирования. Обсуждение. Предложенная методика интеллектуальной поддержки и верификации клинических решений в аспекте выбора наиболее значимых клинических признаков может найти применение для выбора и обоснования тактики ведения пациентов при отсутствии клинических протоколов.
Ключевые слова: поддержка клинических решений, клиническая экспертиза, ранжирование признаков, небольшие группы, статистические методы
Благодарности. Работа поддержана грантом Президента Российской Федерации для государственной поддержки молодых российских ученых – кандидатов наук МК-5723.2021.1.6.
Список литературы
Благодарности. Работа поддержана грантом Президента Российской Федерации для государственной поддержки молодых российских ученых – кандидатов наук МК-5723.2021.1.6.
Список литературы
1. Adu-Amankwaah J., Mprah R., Adekunle A.O., Noah M.L.N., Adzika G.K., Machuki J.O., Sun H. The cardiovascular aspect of COVID-19 // Annals of Medicine. 2021. V. 53. N 1. P. 227–236. https://doi.org/10.1080/07853890.2020.1861644
2. Madjid M., Safavi-Naeini P., Solomon S.D., Vardeny O. Potential effects of coronaviruses on the cardiovascular system: a review // JAMA Cardiology. 2020. V. 5. N 7. P. 831–840. https://doi.org/10.1001/jamacardio.2020.1286
3. Румянцев П.О., Саенко В.Д., Румянцева У.В. Статистические методы анализа в клинической практике. Часть I. Одномерный статистический анализ // Проблемы эндокринологии. 2009. Т. 55. № 5. С. 48–55. https://doi.org/10.14341/probl200955548-55
4. Remeseiro B., Bolon-Canedo V. A review of feature selection methods in medical applications // Computers in Biology and Medicine. 2019. V. 112. P. 103375. https://doi.org/10.1016/j.compbiomed.2019.103375
5. Soares I., Dias J., Rocha H., do Carmo Lopes M., Ferreira B. Feature selection in small databases: a medical-case study // IFMBE Proceedings. 2016. V. 57. P. 814–819. https://doi.org/10.1007/978-3-319-32703-7_158
6. Nezhad M.Z., Zhu D., Li X., Yang K., Levy Ph. SAFS: A deep feature selection approach for precision medicine // Proc. of the 2016 IEEE International Conference on Bioinformatics and Biomedicine (BIBM). 2016. P. 501–506. https://doi.org/10.1109/bibm.2016.7822569
7. Alelyani S. Stable bagging feature selection on medical data // Journal of Big Data. 2021. V. 8. N 11. P. 11. https://doi.org/10.1186/s40537-020-00385-8
8. Wu L., Hu Y., Liu X., Zhang X., Chen W., Yu A.S.L., Kellum J.A., Waitman L.R., Liu M. Feature ranking in predictive models for hospital-acquired acute kidney injury // Scientific Reports. 2018. V. 8. P. 17298. https://doi.org/10.1038/s41598-018-35487-0
9. Golugula A., Lee G., Madabhushi A. Evaluating feature selection strategies for high dimensional, small sample size datasets // Proc. of the 33rd Annual International Conference of the IEEE Engineering in Medicine and Biology Society. 2011. P. 949–952. https://doi.org/10.1109/iembs.2011.6090214
10. Gao L., Wu W. Relevance assignation feature selection method based on mutual information for machine learning // Knowledge-Based Systems. 2020. V. 209. P. 106439 https://doi.org/10.1016/j.knosys.2020.106439
11. Wang B., Li R., Lu Z., Huang Y. Does comorbidity increase the risk of patients with covid-19: Evidence from meta-analysis // Aging. 2020. V. 12. N 7. P. 6049–6057. https://doi.org/10.18632/aging.103000
12. Amin M.S., Chiam Y., Varathan K.D. Identification of significant features and data mining techniques in predicting heart disease // Telematics and Informatics. 2019. V. 36. P. 82–93. https://doi.org/10.1016/j.tele.2018.11.007
13. Joloudari J.H., Joloudari E.H., Saadatfar H., Ghasemigol M., Razavi S.M., Mosavi A., Nabipour N., Shamshirband S., Nadai L. Coronary artery disease diagnosis; ranking the significant features using a random trees model // International Journal of Environmental Research and Public Health. 2020. V. 17. N 3. P. 731. https://doi.org/10.3390/ijerph17030731
14. Pasha S.J., Mohamed E.S. Novel feature reduction (NFR) model with machine learning and data mining algorithms for effective disease risk prediction // IEEE Access. 2020. V. 8. P. 184087–184108. https://doi.org/10.1109/ACCESS.2020.3028714
15. Alam Z., Rahman S., Rahman S. A Random Forest based predictor for medical data classification using feature ranking // Informatics in Medicine Unlocked. 2019. V. 15. P. 100180. https://doi.org/10.1016/j.imu.2019.100180
16. Saqlain S.M., Sher M., Shah F.A., Khan I., Ashraf M.U., Awais M., Ghani A. Fisher score and Matthews correlation coefficient-based feature subset selection for heart disease diagnosis using support vector machines // Knowledge and Information Systems. 2019. V. 58. N 1. P. 139–167. https://doi.org/10.1007/s10115-018-1185-y
17. Shah S.S.M., Batool S.S., Khan I., Muhammad Ashraf U., Abbas S.H., Hussain S.A. Feature extraction through parallel Probabilistic Principal Component Analysis for heart disease diagnosis // Physica A: Statistical Mechanics and its Applications. 2017. V. 482. P. 796–807. https://doi.org/10.1016/j.physa.2017.04.113
18. Abdollahi J., Nouri-Moghaddam B. Feature selection for medical diagnosis: Evaluation for using a hybrid Stacked-Genetic approach in the diagnosis of heart disease // arXiv. 2021. arXiv:2103.08175. https://doi.org/10.48550/arXiv.2103.08175
19. Velusamy D., Ramasamy K. Ensemble of heterogeneous classifiers for diagnosis and prediction of coronary artery disease with reduced feature subset // Computer Methods and Programs in Biomedicine. 2021. V. 198. P. 105770. https://doi.org/10.1016/j.cmpb.2020.105770
20. Ghosh P., Azam S., Jonkman M., Karim A., Shamrat F.M.J., Ignatious E., Shultana S., Beeravolu A.R., De Boer F. Efficient prediction of cardiovascular disease using machine learning algorithms with relief and LASSO feature selection techniques // IEEE Access. 2021. V. 9. P. 19304–19326. https://doi.org/10.1109/ACCESS.2021.3053759
21. Zhou F., Yu T., Du R., Fan G., Liu Y., Liu Z., Xiang J., Wang Y., Song B., Gu X., Guan L., Wei Y., Li H., Wu X., Xu J., Tu S., Zhang Y., Chen H., Cao B. Clinical course and risk factors for mortality of adult inpatients with COVID-19 in Wuhan, China: a retrospective cohort study // Lancet. 2020. V. 395. P. 1054–1062. https://doi.org/10.1016/S0140-6736(20)30566-3
22. Ruan Q., Yang K., Wang W., Jiang L., Song J. Clinical predictors of mortality due to COVID-19 based on an analysis of data of 150 patients from Wuhan, China // Intensive Care Medicine. 2020. V. 46. N 5. P. 846–848. https://doi.org/10.1007/s00134-020-05991-x
23. Li X., Xu S., Yu M., Wang K., Tao Y., Zhou Y., Shi J., Zhou M., Wu B., Yang Z., Zhang C., Yue J., Zhang Z., Renz H., Liu X., Xie J., Xie M., Zhao J. Risk factors for severity and mortality in adult COVID-19 inpatients in Wuhan // Journal of Allergy and Clinical Immunology. 2020. V. 146. N 1. P. 110–118. https://doi.org/10.1016/j.jaci.2020.04.006
24. Liu X., Xue S., Xu J., Ge H., Mao Q., Xu X., Jiang H. Clinical characteristics and related risk factors of disease severity in 101 COVID-19 patients hospitalized in Wuhan, China // Acta Pharmacologica Sinica. 2022. V. 43. N 1. P. 64–75. https://doi.org/10.1038/s41401-021-00627-2
25. Alshaikh M.K., Alotair H., Alnajjar F., Sharaf H., Alhafi B., Alashgar L., Aljuaid M. Cardiovascular risk factors among patients infected with COVID-19 in Saudi Arabia // Vascular Health and Risk Management. 2021. V. 17. P. 161–168. https://doi.org/10.2147/vhrm.s300635
26. Phelps M., Christensen D.M., Gerds T., Fosbøl E., Torp-Pedersen Ch., Schou M., Køber L., Kragholm K., Andersson Ch., Biering-Sørensen T., Christensen H.C., Andersen M.P., Gislason G. Cardiovascular comorbidities as predictors for severe COVID-19 infection or death // European Heart Journal - Quality of Care and Clinical Outcomes. 2021. V. 7. N 2. P. 172–180. https://doi.org/10.1093/ehjqcco/qcaa081
27. Kovvuri V.R.R., Liu S., Seisenberger M., Fan X., Muller B., Fu H. On understanding the influence of controllable factors with a feature attribution algorithm: a medical case study // Proc. of the 2022 International Conference on Innovations in Intelligent SysTems and Applications (INISTA). 2022. P. 1–8. https://doi.org/10.1109/inista55318.2022.9894147
28. Lundberg S.M., Lee S.I. A unified approach to interpreting model predictions // NIPS'17: Proc. of the 31st International Conference on Neural Information Processing Systems. 2017. P. 4768–4777.
29. Bhadra T., Mallik S., Hasan N., Zhao Z. Comparison of five supervised feature selection algorithms leading to top features and gene signatures from multi-omics data in cancer // BMC Bioinformatics. 2022. V. 23. N 3S. P. 153. https://doi.org/10.1186/s12859-022-04678-y
30. Barraza N., Moro S., Ferreyra M., de la Peña A. Mutual information and sensitivity analysis for feature selection in customer targeting: A comparative study // Journal of Information Science. 2019. V. 45. N 1. P. 53–67. https://doi.org/10.1177/0165551518770967
31. Bouchlaghem Y., Akhiat Y., Amjad S. Feature selection: A Review and comparative study // E3S Web of Conferences. 2022. V. 351. P. 01046. https://doi.org/10.1051/e3sconf/202235101046
32. Chen R.-C., Dewi Ch., Huang S.-W., Caraka R.E. Selecting critical features for data classification based on machine learning methods // Journal of Big Data. 2020. V. 7. N 1. P. 52. https://doi.org/10.1186/s40537-020-00327-4
33. Sun P., Wang D., Mok V.C., Shi L. Comparison of feature selection methods and machine learning classifiers for radiomics analysis in glioma grading // IEEE Access. 2019. V. 7. P. 102010–102020. https://doi.org/10.1109/access.2019.2928975
34. Nguyen G., Kim D., Nguyen A. The effectiveness of feature attribution methods and its correlation with automatic evaluation scores // Proc. of the 35th Conference on Neural Information Processing Systems (NeurIPS 2021). 2021.
35. Amrhein V., Korner-Nievergelt F., Roth T. The earth is flat (p > 0.05): significance thresholds and the crisis of unreplicable research // PeerJ. 2017. V. 5. P. e3544. https://doi.org/10.7717/peerj.3544
36. Kolukisa B., Hacilar H., Goy G., Kus M., Bakir-Gungor B., Aral A., Gungor V.C. Evaluation of classification algorithms, linear discriminant analysis and a new hybrid feature selection methodology for the diagnosis of coronary artery disease // Proc. of the 2018 IEEE International Conference on Big Data (Big Data). 2018. P. 2232–2238. https://doi.org/10.1109/BigData.2018.8622609
37. Ricciardi C., Valente A.S., Edmunds K., Cantoni V., Green R., Fiorillo A., Picone I., Santini S., Cesarelli M. Linear discriminant analysis and principal component analysis to predict coronary artery disease // Health Informatics Journal. 2020. V. 26. N 3. P. 2181–2192. https://doi.org/10.1177/1460458219899210
38. Breiman L. Random Forests // Machine Learning. 2001. V. 45. P. 5–32. https://doi.org/10.1023/A:1010933404324
39. Ke G., Meng Q., Finley T., Wang T., Chen W., Ma W., Ye Q., Liu T.-Y. LightGBM: A highly efficient gradient boosting decision tree // Proc. of the 31st Conference on Neural Information Processing Systems (NIPS 2017). 2017. P. 3149–3157.
40. Prokhorenkova L., Gusev G., Vorobev A., Dorogush A.V., Gulin A. CatBoost: unbiased boosting with categorical features // Proc. of the 32nd Conference on Neural Information Processing Systems (NeurIPS 2018). 2018.
2. Madjid M., Safavi-Naeini P., Solomon S.D., Vardeny O. Potential effects of coronaviruses on the cardiovascular system: a review // JAMA Cardiology. 2020. V. 5. N 7. P. 831–840. https://doi.org/10.1001/jamacardio.2020.1286
3. Румянцев П.О., Саенко В.Д., Румянцева У.В. Статистические методы анализа в клинической практике. Часть I. Одномерный статистический анализ // Проблемы эндокринологии. 2009. Т. 55. № 5. С. 48–55. https://doi.org/10.14341/probl200955548-55
4. Remeseiro B., Bolon-Canedo V. A review of feature selection methods in medical applications // Computers in Biology and Medicine. 2019. V. 112. P. 103375. https://doi.org/10.1016/j.compbiomed.2019.103375
5. Soares I., Dias J., Rocha H., do Carmo Lopes M., Ferreira B. Feature selection in small databases: a medical-case study // IFMBE Proceedings. 2016. V. 57. P. 814–819. https://doi.org/10.1007/978-3-319-32703-7_158
6. Nezhad M.Z., Zhu D., Li X., Yang K., Levy Ph. SAFS: A deep feature selection approach for precision medicine // Proc. of the 2016 IEEE International Conference on Bioinformatics and Biomedicine (BIBM). 2016. P. 501–506. https://doi.org/10.1109/bibm.2016.7822569
7. Alelyani S. Stable bagging feature selection on medical data // Journal of Big Data. 2021. V. 8. N 11. P. 11. https://doi.org/10.1186/s40537-020-00385-8
8. Wu L., Hu Y., Liu X., Zhang X., Chen W., Yu A.S.L., Kellum J.A., Waitman L.R., Liu M. Feature ranking in predictive models for hospital-acquired acute kidney injury // Scientific Reports. 2018. V. 8. P. 17298. https://doi.org/10.1038/s41598-018-35487-0
9. Golugula A., Lee G., Madabhushi A. Evaluating feature selection strategies for high dimensional, small sample size datasets // Proc. of the 33rd Annual International Conference of the IEEE Engineering in Medicine and Biology Society. 2011. P. 949–952. https://doi.org/10.1109/iembs.2011.6090214
10. Gao L., Wu W. Relevance assignation feature selection method based on mutual information for machine learning // Knowledge-Based Systems. 2020. V. 209. P. 106439 https://doi.org/10.1016/j.knosys.2020.106439
11. Wang B., Li R., Lu Z., Huang Y. Does comorbidity increase the risk of patients with covid-19: Evidence from meta-analysis // Aging. 2020. V. 12. N 7. P. 6049–6057. https://doi.org/10.18632/aging.103000
12. Amin M.S., Chiam Y., Varathan K.D. Identification of significant features and data mining techniques in predicting heart disease // Telematics and Informatics. 2019. V. 36. P. 82–93. https://doi.org/10.1016/j.tele.2018.11.007
13. Joloudari J.H., Joloudari E.H., Saadatfar H., Ghasemigol M., Razavi S.M., Mosavi A., Nabipour N., Shamshirband S., Nadai L. Coronary artery disease diagnosis; ranking the significant features using a random trees model // International Journal of Environmental Research and Public Health. 2020. V. 17. N 3. P. 731. https://doi.org/10.3390/ijerph17030731
14. Pasha S.J., Mohamed E.S. Novel feature reduction (NFR) model with machine learning and data mining algorithms for effective disease risk prediction // IEEE Access. 2020. V. 8. P. 184087–184108. https://doi.org/10.1109/ACCESS.2020.3028714
15. Alam Z., Rahman S., Rahman S. A Random Forest based predictor for medical data classification using feature ranking // Informatics in Medicine Unlocked. 2019. V. 15. P. 100180. https://doi.org/10.1016/j.imu.2019.100180
16. Saqlain S.M., Sher M., Shah F.A., Khan I., Ashraf M.U., Awais M., Ghani A. Fisher score and Matthews correlation coefficient-based feature subset selection for heart disease diagnosis using support vector machines // Knowledge and Information Systems. 2019. V. 58. N 1. P. 139–167. https://doi.org/10.1007/s10115-018-1185-y
17. Shah S.S.M., Batool S.S., Khan I., Muhammad Ashraf U., Abbas S.H., Hussain S.A. Feature extraction through parallel Probabilistic Principal Component Analysis for heart disease diagnosis // Physica A: Statistical Mechanics and its Applications. 2017. V. 482. P. 796–807. https://doi.org/10.1016/j.physa.2017.04.113
18. Abdollahi J., Nouri-Moghaddam B. Feature selection for medical diagnosis: Evaluation for using a hybrid Stacked-Genetic approach in the diagnosis of heart disease // arXiv. 2021. arXiv:2103.08175. https://doi.org/10.48550/arXiv.2103.08175
19. Velusamy D., Ramasamy K. Ensemble of heterogeneous classifiers for diagnosis and prediction of coronary artery disease with reduced feature subset // Computer Methods and Programs in Biomedicine. 2021. V. 198. P. 105770. https://doi.org/10.1016/j.cmpb.2020.105770
20. Ghosh P., Azam S., Jonkman M., Karim A., Shamrat F.M.J., Ignatious E., Shultana S., Beeravolu A.R., De Boer F. Efficient prediction of cardiovascular disease using machine learning algorithms with relief and LASSO feature selection techniques // IEEE Access. 2021. V. 9. P. 19304–19326. https://doi.org/10.1109/ACCESS.2021.3053759
21. Zhou F., Yu T., Du R., Fan G., Liu Y., Liu Z., Xiang J., Wang Y., Song B., Gu X., Guan L., Wei Y., Li H., Wu X., Xu J., Tu S., Zhang Y., Chen H., Cao B. Clinical course and risk factors for mortality of adult inpatients with COVID-19 in Wuhan, China: a retrospective cohort study // Lancet. 2020. V. 395. P. 1054–1062. https://doi.org/10.1016/S0140-6736(20)30566-3
22. Ruan Q., Yang K., Wang W., Jiang L., Song J. Clinical predictors of mortality due to COVID-19 based on an analysis of data of 150 patients from Wuhan, China // Intensive Care Medicine. 2020. V. 46. N 5. P. 846–848. https://doi.org/10.1007/s00134-020-05991-x
23. Li X., Xu S., Yu M., Wang K., Tao Y., Zhou Y., Shi J., Zhou M., Wu B., Yang Z., Zhang C., Yue J., Zhang Z., Renz H., Liu X., Xie J., Xie M., Zhao J. Risk factors for severity and mortality in adult COVID-19 inpatients in Wuhan // Journal of Allergy and Clinical Immunology. 2020. V. 146. N 1. P. 110–118. https://doi.org/10.1016/j.jaci.2020.04.006
24. Liu X., Xue S., Xu J., Ge H., Mao Q., Xu X., Jiang H. Clinical characteristics and related risk factors of disease severity in 101 COVID-19 patients hospitalized in Wuhan, China // Acta Pharmacologica Sinica. 2022. V. 43. N 1. P. 64–75. https://doi.org/10.1038/s41401-021-00627-2
25. Alshaikh M.K., Alotair H., Alnajjar F., Sharaf H., Alhafi B., Alashgar L., Aljuaid M. Cardiovascular risk factors among patients infected with COVID-19 in Saudi Arabia // Vascular Health and Risk Management. 2021. V. 17. P. 161–168. https://doi.org/10.2147/vhrm.s300635
26. Phelps M., Christensen D.M., Gerds T., Fosbøl E., Torp-Pedersen Ch., Schou M., Køber L., Kragholm K., Andersson Ch., Biering-Sørensen T., Christensen H.C., Andersen M.P., Gislason G. Cardiovascular comorbidities as predictors for severe COVID-19 infection or death // European Heart Journal - Quality of Care and Clinical Outcomes. 2021. V. 7. N 2. P. 172–180. https://doi.org/10.1093/ehjqcco/qcaa081
27. Kovvuri V.R.R., Liu S., Seisenberger M., Fan X., Muller B., Fu H. On understanding the influence of controllable factors with a feature attribution algorithm: a medical case study // Proc. of the 2022 International Conference on Innovations in Intelligent SysTems and Applications (INISTA). 2022. P. 1–8. https://doi.org/10.1109/inista55318.2022.9894147
28. Lundberg S.M., Lee S.I. A unified approach to interpreting model predictions // NIPS'17: Proc. of the 31st International Conference on Neural Information Processing Systems. 2017. P. 4768–4777.
29. Bhadra T., Mallik S., Hasan N., Zhao Z. Comparison of five supervised feature selection algorithms leading to top features and gene signatures from multi-omics data in cancer // BMC Bioinformatics. 2022. V. 23. N 3S. P. 153. https://doi.org/10.1186/s12859-022-04678-y
30. Barraza N., Moro S., Ferreyra M., de la Peña A. Mutual information and sensitivity analysis for feature selection in customer targeting: A comparative study // Journal of Information Science. 2019. V. 45. N 1. P. 53–67. https://doi.org/10.1177/0165551518770967
31. Bouchlaghem Y., Akhiat Y., Amjad S. Feature selection: A Review and comparative study // E3S Web of Conferences. 2022. V. 351. P. 01046. https://doi.org/10.1051/e3sconf/202235101046
32. Chen R.-C., Dewi Ch., Huang S.-W., Caraka R.E. Selecting critical features for data classification based on machine learning methods // Journal of Big Data. 2020. V. 7. N 1. P. 52. https://doi.org/10.1186/s40537-020-00327-4
33. Sun P., Wang D., Mok V.C., Shi L. Comparison of feature selection methods and machine learning classifiers for radiomics analysis in glioma grading // IEEE Access. 2019. V. 7. P. 102010–102020. https://doi.org/10.1109/access.2019.2928975
34. Nguyen G., Kim D., Nguyen A. The effectiveness of feature attribution methods and its correlation with automatic evaluation scores // Proc. of the 35th Conference on Neural Information Processing Systems (NeurIPS 2021). 2021.
35. Amrhein V., Korner-Nievergelt F., Roth T. The earth is flat (p > 0.05): significance thresholds and the crisis of unreplicable research // PeerJ. 2017. V. 5. P. e3544. https://doi.org/10.7717/peerj.3544
36. Kolukisa B., Hacilar H., Goy G., Kus M., Bakir-Gungor B., Aral A., Gungor V.C. Evaluation of classification algorithms, linear discriminant analysis and a new hybrid feature selection methodology for the diagnosis of coronary artery disease // Proc. of the 2018 IEEE International Conference on Big Data (Big Data). 2018. P. 2232–2238. https://doi.org/10.1109/BigData.2018.8622609
37. Ricciardi C., Valente A.S., Edmunds K., Cantoni V., Green R., Fiorillo A., Picone I., Santini S., Cesarelli M. Linear discriminant analysis and principal component analysis to predict coronary artery disease // Health Informatics Journal. 2020. V. 26. N 3. P. 2181–2192. https://doi.org/10.1177/1460458219899210
38. Breiman L. Random Forests // Machine Learning. 2001. V. 45. P. 5–32. https://doi.org/10.1023/A:1010933404324
39. Ke G., Meng Q., Finley T., Wang T., Chen W., Ma W., Ye Q., Liu T.-Y. LightGBM: A highly efficient gradient boosting decision tree // Proc. of the 31st Conference on Neural Information Processing Systems (NIPS 2017). 2017. P. 3149–3157.
40. Prokhorenkova L., Gusev G., Vorobev A., Dorogush A.V., Gulin A. CatBoost: unbiased boosting with categorical features // Proc. of the 32nd Conference on Neural Information Processing Systems (NeurIPS 2018). 2018.