Menu
Publications
2024
2023
2022
2021
2020
2019
2018
2017
2016
2015
2014
2013
2012
2011
2010
2009
2008
2007
2006
2005
2004
2003
2002
2001
Editor-in-Chief
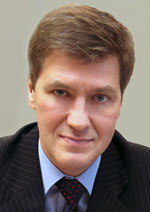
Nikiforov
Vladimir O.
D.Sc., Prof.
Partners
MULTISCALE DIFFERENTIAL METHOD FOR DIGITAL IMAGE SHARPENING
Read the full article

Article in Russian
Abstract
Abstract
We have proposed and tested a novel method for digital image sharpening. The method is based on multi-scale image analysis, calculation of differential responses of image brightness in different spatial scales, and the subsequent calculation of a restoration function, which sharpens the image by simple subtraction of its brightness values from those of the original image. The method features spatial transposition of the restoration function elements, its normalization, and taking into account the sign of the brightness differential response gradient close to the object edges. The calculation algorithm for the proposed method makes use of integer arithmetic that significantly reduces the computation time. The paper shows that for the images containing small amount of the blur due to the residual aberrations of an imaging system, only the first two scales are needed for the calculation of the restoration function. Similar to the blind deconvolution, the method requires no a priori information about the nature and magnitude of the blur kernel, but it is computationally inexpensive and is much easier in practical implementation. The most promising applications of the method are machine vision and surveillance systems based on real-time intelligent pattern recognition and decision making.
Keywords: digital image, image contrast, sharpness, blur kernel, deconvolution
Acknowledgements. The research has been carried out under financial support of the Ministry of Education & Science of the Russian Federation.
References
Acknowledgements. The research has been carried out under financial support of the Ministry of Education & Science of the Russian Federation.
References
1. Bezzubik V.V., Belashenkov N.R., Nikiforov V.O. Metod kolichestvennoi otsenki kontrasta tsifrovogo izobrazheniya [Quantitative estimation method of digital image contrast]. Scientific and Technical Journal of Information Technologies, Mechanics and Optics, 2010, no. 6 (70), pp. 86–88.
2. Polesel A., Ramponi G., Mathews V.J. Image enhancement via adaptive unsharp masking. IEEE Transactions on Image Processing, 2000, vol. 9, no. 3, pp. 505–510. doi: 10.1109/83.826787
3. Cao G., Zhao Y., Ni R., Kot A.C. Unsharp masking sharpening detection via overshoot artifacts analysis.IEEE Signal Processing Letters, 2011, vol. 18, no. 10, pp. 603–606. doi: 10.1109/LSP.2011.2164791
4. Kim S.H., Allebach J.P. Optimal unsharp mask for image sharpening and noise removal. Journal of Electronic Imaging, 2005,vol. 14, no. 2, art. 023005, pp. 1–13. doi: 10.1117/1.1924510
5. Kotera H., Wang H. Multiscale image sharpening adaptive to edge profile. Journal of Electronic Imaging, 2005, vol. 14, no. 1, art. 013002, pp. 1–17. doi:10.1117/1.1866147
6. Kwok N.M., Shi H.Y., Fang G., Ha Q.P. Intensity-based gain adaptive unsharp masking for image contrast enhancement. Proc. 5th Int. Congress on Image and Signal Processing, CISP 2012. Chongqing, China, 2012, pp. 529–533. doi: 10.1109/CISP.2012.6469772
7. Hong H., Li L., Park I.K., Zhang T. Universal deblurring method for real images using transition region. Optical Engineering, 2012,vol. 51, no. 4,art. 047006. doi: 10.1117/1.OE.51.4.047006
8. Loza A., Bull D.R., Hill P.R., Achim A.M.Automatic contrast enhancement of low-light images based on local statistics of wavelet coefficients. Digital Signal Processing, 2013,vol. 23, no. 6, pp. 1856–1866. doi: 10.1016/j.dsp.2013.06.002
9. Morigi S., Reichel L., Sgallari F., Shyshkov A. Cascadic multiresolution methods for image deblurring. SIAM Journal on Imaging Sciences, 2008, vol. 1, no. 1, pp. 51–74. doi:10.1137/070694065
10.Levin A., Weiss Y., Durand F., Freeman W.T. Understanding and evaluating blind deconvolution algorithms. Proc. IEEE Computer Society Conference On Computer Vision and Pattern Recognition Workshops.Miami, US, 2009, pp. 1964–1971. doi: 10.1109/CVPRW.2009.5206815
11.Barone F., Rossi C. Deconvolution with partially known kernel of nonnegative signals. Machine Vision and Applications, 1990, vol. 3, no. 2, pp. 107–115. doi: 10.1007/BF01212194
12.Markham J., Conchello J.-A. Parametric blind deconvolution: a robust method for the simultaneous estimation of image and blur. Journal of Optical Society of America A: Optics and Image Science, and Vision, 1999, vol. 16, no. 10, pp. 2377–2391.
13.Kundur D., Hatzinakos D. Blind image deconvolution. IEEE Signal Processing Magazine, 1996, vol. 13, no. 3, pp. 43–64. doi: 10.1109/79.489268
14.Mo X., Jiao J., Shen C. PSF-constraints based iterative blind deconvolution method for image deblurring. Lecture Notes in Computer Science,2010, vol. 5916 LNCS, pp. 141–151. doi:10.1007/978-3-642-11301-7_17
15.Laligant O., Truchetet F., Dupasquier A. Edge enhancement by local deconvolution.Pattern Recognition, 2005, vol. 38, no. 5, pp. 661–672. doi:10.1016/j.patcog.2004.10.006
16.The Oxford Dictionary of Statistical Terms. 6th ed. Ed. Y. Dodge. Oxford, Oxford University Press, 2003, 498 p.
17.Woods R.E., Gonzales R.C. Digital Image Processing. 2nd ed. Upper Saddle River, Prentice Hall, 2002, 793 p.