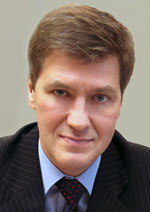
Nikiforov
Vladimir O.
D.Sc., Prof.
doi: 10.17586/2226-1494-2015-15-1-122-129
COMPARISON OF VARIOUS APPROACHES TO MULTI-CHANNEL
INFORMATION FUSION IN C-OTDR SYSTEMS FOR REMOTE MONITORING OF EXTENDED OBJECTS
Read the full article

For citation: Timofeev A.V. Comparison of various approaches to multi-channel Information fusion in c-otdr systems for remote monitoring of extended objects. Scientific and Technical Journal of Information Technologies, Mechanics and Optics, 2015, no. 1, vol.15, pp. 122–129 (in English)
Abstract
The paper presents new results concerning selection of optimal information fusion formula for ensembles of COTDR channels. Here C-OTDR is a coherent optical time domain reflectometer. Each of these channels provides data for appropriate automatic classifier which is designed to classify the elastic vibration sources in the multiclass case. Those classifiers form a so-called classifiers ensemble. Ensembles of Lipschitz Classifiers were considered. In this case the goal of information fusion is to create an integral classificator designed for effective classification of seismoacoustic target events. The Matching Pursuit Optimization Ensemble Classifiers (MPOEC), the Linear Programming Boosting (LP-Boost) (LP-β and LP-B variants), the Multiple Kernel Learning (MKL), and Weighing of Inversely as Lipschitz Constants (WILC) approaches were compared. The WILC is a brand new approach to optimal fusion of Lipschitz Classifiers Ensembles. The basics of these methods have been briefly described along with intrinsic features. All of those methods are based on reducing the task of choosing convex hull parameters to a solution of an optimization problem. All of the mentioned approaches can be successfully used for using in the C-OTDR system data processing. Results of practical usage are presented.
References
1. Choi K.N., Juarez J.C., Taylor H.F. Distributed fiber-optic pressure/seismic sensor for low-cost monitoring. Proceedings of SPIE - The International Society for Optical Engineering, 2003, vol. 5090, pp. 134–141. doi: 10.1117/12.484911
2. Timofeev A.V., Egorov D.V. Multichannel classification of target signals by means of an SVM ensemble in C-OTDR systems for remote monitoring of extended objects. Proc. MVML-2014. Prague, 2014, vol. 1, pp. 22–32.
3. Timofeev A.V. The guaranteed estimation of the Lipschitz classifier accuracy: confidence set approach. Journal of the Korean Statistical Society, 2012, vol. 41, no. 1, pp. 105–114. doi: 10.1016/j.jkss.2011.07.005
4. Von Luxburg U., Bousquet O. Distance-based classification with Lipschitz functions. Journal of Machine Learning Research, 2004, vol. 5, pp. 669–695.
5. Murty M.N., Devi V.S. Pattern Recognition: an Algorithmic Approach (Undergraduate Topics in Computer Science). London, Springer, 2011, 263 p.
6. Hofmann T., Sholkopf B., Smola A.J. Kernel methods in machine learning. Annals of Statistics, 2008, vol. 36, no. 3, pp. 1171–1220. doi: 10.1214/009053607000000677
7. Lanckriet G.R.G., Cristianini N., Bartlett P., Et Ghaoui L., Jordan M.I. Learning the kernel matrix with semidefinite programming. Journal of Machine Learning Research, 2004, vol. 5, pp. 27–72.
8. Bach F.R., Lanckriet G.R.G., Jordan M.I. Multiple kernel learning, conic duality, and the SMO algorithm. Proc. 21st Int. Conf. on Machine Learning, ICML 2004. Banff, Canada, 2004, pp. 41–48.
9. Hears M.A., Dumais S.T., Osman E., Platt J., Scholkopf B. Support vector machines. IEEE Intelligent Systems, 1998, vol. 13, no. 4, pp. 18–28. doi: 10.1109/5254.708428
10. Demiriz A., Bennett K.P., Shawe-Taylor J. Linear programming boosting via column generation. Machine Learning, 2002, vol. 46, no. 1–3, pp. 225–254. doi: 10.1023/A:1012470815092
11. Gehler P., Nowozin S. On feature combination for multiclass object classification. Proc. ICCV, 2009, pp. 47– 50.
12. Weston J., Watkins C. Support vector machines for multi-class pattern recognition. Proc. 7th European Symposium on Artificial Neural Networks, 1999, pp. 219–224.
13. Mallat S.G., Zhang Z. Matching pursuit with time-frequency dictionaries. IEEE Transaction on Signal Processing, 1993, vol. 41, no. 12, pp. 3397–3415. doi: 10.1109/78.258082
14. Mao S., Jiao L.C., Xiong L., Gou S. Greedy optimization classifiers ensemble based on diversity. Pattern Recognition, 2011, vol. 44, no. 6, pp. 1245–1261.
15. Blimes J.A. A gentle tutorial of the EM algorithm and its application to parameter estimation for Gaussian mixture and hidden Markov models. Technical Report TR-97-021. Berkley, California, International Computer Science Institute, 1998, pp. 97–021.