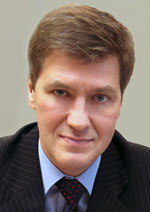
Nikiforov
Vladimir O.
D.Sc., Prof.
doi: 10.17586/2226-1494-2016-16-3-387-401
ANALYSIS OF MULTIMODAL FUSION TECHNIQUES FOR AUDIO-VISUAL SPEECH RECOGNITION
Read the full article

For citation: Ivanko D.V., Kipyatkova I.S., Ronzhin A.L., Karpov A.A. Analysis of multimodal fusion techniques for audio-visual speech recognition. Scientific and Technical Journal of Information Technologies, Mechanics and Optics, 2016, vol. 16, no. 3, pp. 387–401. doi: 10.17586/2226-1494-2016-16-3-387-401
Abstract
The paper deals with analytical review, covering the latest achievements in the field of audio-visual (AV) fusion (integration) of multimodal information. We discuss the main challenges and report on approaches to address them. One of the most important tasks of the AV integration is to understand how the modalities interact and influence each other. The paper addresses this problem in the context of AV speech processing and speech recognition. In the first part of the review we set out the basic principles of AV speech recognition and give the classification of audio and visual features of speech. Special attention is paid to the systematization of the existing techniques and the AV data fusion methods. In the second part we provide a consolidated list of tasks and applications that use the AV fusion based on carried out analysis of research area. We also indicate used methods, techniques, audio and video features. We propose classification of the AV integration, and discuss the advantages and disadvantages of different approaches. We draw conclusions and offer our assessment of the future in the field of AV fusion. In the further research we plan to implement a system of audio-visual Russian continuous speech recognition using advanced methods of multimodal fusion.
Acknowledgements. The research is financially supported by the Russian Foundation for Basic Research (projects No. 15-07-04415-a and 15-07-04322-а) and by the Council for Grants of the President of Russia (projects No. MD-3035.2015.8 and МК-5209.2015.8).
References
1. Katsaggelos A.K., Bahaadini S., Molina R. Audiovisual fusion: challenges and new approaches. Proc. of the IEEE, 2015, vol. 103, no. 9, pp. 1635–1653. doi: 10.1109/JPROC.2015.2459017
2. Narayanan S., Alwan A. Noise source models for fricative consonants. IEEE Transactions on Speech and Audio Processing, 2000, vol. 8, no. 3, pp. 328–344. doi: 10.1109/89.841215
3. Yehia H., Rubin P., Vatikiotis-Bateson E. Quantitative association of vocal-tract and facial behavior. Speech Communication, 1998, vol. 26, no. 1–2, pp. 23–43.
4. McGurk H., MacDonald J. Hearing lips and seeing voices. Nature, 1976, vol. 264, no. 5588, pp. 746–748.
5. Hershey J., Attias H., Jojic N., Kristjansson T. Audio-visual graphical models for speech processing. Proc. IEEE International Conference Acoustics, Speech and Signal Processing, 2004, vol. 5, pp. 649–652.
6. Nock H.J., Iyengar G., Neti C. Speaker localisation using audio-visual synchrony: an empirical study. Lecture Notes in Computer Science, 2003, vol. 2728, pp. 488–499.
7. Ngiam J., Khosla A., Kim M., Nam J., Lee H., Ng A.Y. Multimodal deep learning. Proc. 28th International Conference on Machine Learning. Bellevue, USA, 2011, pp. 689–696.
8. Noda K., Yamaguchi Y., Nakadai K., Okuno H.G., Ogata T. Audio-visual speech recognition using deep learning. Application Intelligence, 2015, vol. 42, no. 4, pp. 722–737. doi: 10.1007/s10489-014-0629-7
9. Nefian A.V., Liang L., Pi X., Liu X., Murphy K. Dynamic Bayesian networks for audio-visual speech recognition. EURASIP Journal on Advanced Signal Processing, 2002, vol. 2002, no. 11, pp. 1274–1288. doi: 10.1155/S1110865702206083
10. Terry L., Katsaggelos A.K. A phone-viseme dynamic Bayesian network for audio-visual automatic speech recognition. Proc. 19th International Conference Pattern Recognition, 2008, art. 4761927.
11. Ninomiya H., Kitaoka N., Tamura S., Iribe Y., Takeda K. Integration of deep bottleneck features for audio-visual speech recognition. Proc. 16th Annual Conference of the International Speech Communication Association, Interspeech 2015. Dresden, Germany, 2015, pp. 563–567.
12. Kalantari S., Dean D., Ghaemmaghami H., Sridharan S., Fookes C. Cross database training of audio-visual hidden Markov models for phone recognition. Proc. 16th Annual Conference of the International Speech Communication Association, Interspeech 2015. Dresden, Germany, 2015, pp. 553–557.
13. Biswas A., Sahu P.K., Bhowmick A., Chandra M. AAM based features for multiple camera visual speech recognition in car environment. Procedia Computer Science, 2015, vol. 57, pp. 614–621. doi: 10.1016/j.procs.2015.07.417
14. Mroueh Y., Marcheret E., Goel V. Deep multimodal learning for audio-visual speech recognition. Proc. IEEE Int. Conf. on Acoustics, Speech and Signal Processing. Brisbane, Australia, 2015, pp. 2130–2134. doi: 10.1109/ICASSP.2015.7178347
15. Navarathna R., Dean D., Sridharan S., Lucey P. Multiple cameras for audio-visual speech recognition in an automotive environment. Computer Speech and Language, 2013, vol. 27, no. 4, pp. 911–927. doi: 10.1016/j.csl.2012.07.005
16. Marcheret E., Potamianos G., Vopicka J., Goel V. Detecting audio-visual synchrony using deep neural networks. Proc. 16th Annual Conference of the International Speech Communication Association, Interspeech 2015. Dresden, Germany, 2015, pp. 548–552.
17. Aleksic P., Katsaggelos A. An audio-visual person identification and verification system using FAPS as visual features. Proc. ACM Workshop Multimodal User Authentication, 2003, pp. 80–84.
18. Keating P.A. Underspecification in phonetics. Phonology, 1988, vol. 5, no. 2, pp. 275–292.
19. Bengio S. Multimodal authentication using asynchronous HMMs. Lecture Notes in Computer Science, 2003, vol. 2688, pp. 770–777.
20. Kanak A., Erzin E., Yemez Y., Tekalp A.M. Joint audio-video processing for biometric speaker identification. Proc. IEEE International Conference on Acoustic Speech and Signal Processing. Hong Kong, 2003, vol. 2, pp. 377–380.
21. Chetty G., Wagner M. Audio-visual multimodal fusion for biometric person authentication and liveness verification. Proc. NICTA-HCSNet Multimodal User Interaction Workshop, 2006, vol. 57, pp. 17–24.
22. Atrey P.K., Kankanhalli M.S., Jain R. Information assimilation framework for event detection in multimedia surveillance systems. Multimedia Systems, 2006, vol. 12, no. 3, pp. 239–253. doi: 10.1007/s00530-006-0063-8
23. Xu H., Chua T.-S. Fusion of AV features and external information sources for event detection in team sports video. ACM Transactions on Multimedia Computing, Communications and Applications, 2006, vol. 2, no. 1, pp. 44–67.
24. Shao X., Barker J. Stream weight estimation for multistream audio-visual speech recognition in a multispeaker environment. Speech Communication, 2008, vol. 50, no. 4, pp. 337–353. doi: 10.1016/j.specom.2007.11.002
25. Petridis S., Rajgarhia V., Pantic M. Comparison of single-model and multiple-model prediction-based audiovisual fusion. Facial Analysis, Animation and Auditory-Visual Speech Processing, FAAVSP. Vienna, Austria, 2015, pp. 109–114.
26. Zou X., Bhanu B. Tracking humans using multi-modal fusion. Proc. IEEE Computer Society Conference Computer Vision and Pattern Recognition Workshops. San Diego, USA, 2005, pp. 4–11. doi: 10.1109/CVPR.2005.545
27. Talantzis F., Pnevmatikakis A., Polymenakos L.C. Real time audio-visual person tracking. Proc. IEEE 8th Workshop Multimedia Signal Process. Victoria, Canada, 2006, pp. 243–247. doi: 10.1109/MMSP.2006.285306
28. Vermaak J., Gangnet M., Blake A., Perez P. Sequential Monte Carlo fusion of sound and vision for speaker tracking. Proc. IEEE International Conference on Computer Vision. Vancouver, Canada, 2001, vol. 1, pp. 741–745.
29. Gatica-Perez D., Lathoud G., McCowan I., Odobez J.M., Moore D. Audio-visual speaker tracking with importance particle filters. Proc. IEEE International Conference on Image Processing. Barcelona, Spain, 2003, vol. 3, pp. 25–28.
30. Crisan D., Doucet A. A survey of convergence results on particle filtering methods for practitioners. IEEE Transactions on Signal Processing, 2002, vol. 50, no. 3, pp. 736–746. doi: 10.1109/78.984773
31. Zotkin D.N., Duraiswami R., Davis L.S. Joint audio-visual tracking using particle filters. EURASIP Journal on Applied Signal Processing, 2002, vol. 2002, no. 11, pp. 1154–1164. doi: 10.1155/S1110865702206058
32. Gehrig T., Nickel K., Ekenel H. K., Klee U., McDonough J. Kalman filters for audio-video source localization. Proc. IEEE Workshop on Applied Signal Processing to Audio and Acoustics. New Paltz, USA, 2005, pp. 118–121. doi: 10.1109/ASPAA.2005.1540183
33. Nock H.J., Iyengar G., Neti C. Speaker localisation using audio-visual synchrony: an empirical study. Lecture Notes in Computer Science, 2003, vol. 2728, pp. 488–499.
34. Wu Y., Chang K.C., Chang E.Y., Smith J.R. Optimal multimodal fusion for multimedia data analysis. Proc. 12th ACM International Conference on Multimedia. New York, 2004, pp. 572–579.
35. Adams W.H., Iyengar G., Lin C.-Y., Naphade M.R., Neti C., Nock H.J., Smith J.R. Semantic indexing of multimedia content using visual, audio, text cues. EURASIP Journal on Advanced Signal Processing, vol. 2003, no. 2, pp. 170–185. doi: 10.1155/S1110865703211173
36. Iyengar G., Nock H.J., Neti C. Discriminative model fusion for semantic concept detection and annotation in video. Proc. 11th ACM International Conference on Multimedia. Berkeley, USA, 2003, pp. 255–258.
37. Anderson B.D.O., Moore J.B. Optimal Filtering. NY, Courier Dover, 2012, 368 p.
38. Estellers V., Gurban M., Thiran J.-P. On dynamic stream weighting for audio-visual speech recognition. IEEE Transactions on Audio, Speech and Language Processing, 2012, vol. 20, no. 4, pp. 1145–1157. doi: 10.1109/TASL.2011.2172427
39. Hsu W.H.-M., Chang S.-F. Generative, discriminative, ensemble learning on multi-modal perceptual fusion toward news video story segmentation. Proc. IEEE International Conference on Multimedia and Expo. Taipei, Taiwan, 2004, vol. 2, pp. 1091–1094.
40. Terry L.H., Livescu K., Pierrehumbert J.B., Katsaggelos A.K. Audio-visual anticipatory coarticulation modeling by human and machin. Proc. 11th Annual Conference of the International Speech Communication Association, Interspeech 2010. Makuhari, Japan, 2010, pp. 2682–2685.
41. Terry L. Audio-Visual Asynchrony Modeling and Analysis for Speech Alignment and Recognition. Ph.D. dissertation. Evanston, USA, Northwestern University, 2011.
42. Kryvonos Iu.G., Krak Iu.V., Barmak O.V., Shkilniuk D.V. Construction and identification of gesture communication elements. Kibernetika i Sistemny Analiz, 2013, no. 2, pp. 3–14.
43. Zhou Z., Zhao G., Hong X., Pietikainen M. A review of recent advances in visual speech decoding. Image and Vision Computing, 2014, vol. 32, no. 9, pp. 590–605. doi: 10.1016/j.imavis.2014.06.004
44. Dupont S., Luettin J. Audio-visual speech modeling for continuous speech recognition. IEEE Transactions on Multimedia, 2000, vol. 2, no. 3, pp. 141–151. doi: 10.1109/6046.865479
45. Karpov A., Ronzhin A., Kipyatkova I. Designing a multimodal corpus of audio-visual speech using a high-speed camera. Proc. 11th IEEE Int. Conf. on Signal Processing. Beijing, China, 2012, pp. 519–522. doi: 10.1109/ICoSP.2012.6491539
46. Karpov A., Kipyatkova I., Zelezny M. A framework for recording audio-visual speech corpora with a microphone and a high-speed camera. Lecture Notes in Computer Science, 2014, vol. 8773, pp. 50–57.
47. Karpov A.A. An automatic multimodal speech recognition system with audio and video information. Automation and Remote Control, 2014, vol. 75, no. 12, pp. 2190–2200. doi: 10.1134/S000511791412008X
48. Basov O.O., Karpov A.A. Analysis of strategies and methods for multimodal information fusion. Informatsionno-Upravliaiushchie Sistemy, 2015, no. 2(75), pp. 7–14.
49. Kovshov E.E., Zavistovskaya T.A. Development of software for testing algorithms design information structures. Cloud of Science, 2014, vol. 1, no. 2, pp. 279–291.
50. Krak Yu.V., Ternov A.S. Lipsreading at sign language: synthesis and analysis. Speech Technology, 2014, no. 2, pp. 121–131.
51. Snoek C.G., Worring M., Smeulders A.W. Early versus late fusion in semantic video analysis. Proc. 13th Annual ACM International Conference on Multimedia. Singapore, 2005, pp. 399–402. doi: 10.1145/1101149.1101236
52. Wu Z., Cai L., Meng H. Multi-level fusion of audio and visual features for speaker identification. Lecture Notes in Computer Science, 2005, vol. 3832, pp. 493–499.
53. Atrey P.K., Hossain M.A., Saddik A.E., Kankanhalli M.S. Multimodal fusion for multimedia analysis: a survey. Multimedia Systems, 2010, vol. 16, no. 6, pp. 345–379. doi: 10.1007/s00530-010-0182-0
54. Barnard M., Koniusz P., Wang W., Kittler J., Naqvi S.M., Chambers J. Robust multi-speaker tracking via dictionary learning and identity modeling. IEEE Transactions on Multimedia, 2014, vol. 16, no. 3, pp. 864–880. doi: 10.1109/TMM.2014.2301977
55. Bayesian Network. Available at: https://en.wikipedia.org/wiki/Bayesian_network (accessed 20.12.2015).
56. Zhao Y., Wang H., Ji Q. Audio-visual Tibetan speech recognition based on a deep dynamic Bayesian network for natural human robot interaction. International Journal of Advanced Robotic Systems, 2012, vol. 9, no. 258, pp. 57–72. doi: 10.5772/54000
57. Noulas A.K., Krose B.J. EM detection of common origin of multi-modal cues. Proc. 8th ACM International Conference on Multimodal Interfaces. Banff, Canada, 2006, pp. 201–208. doi: 10.1145/1180995.1181037
58. Dielmann A., Renals S. Automatic meeting segmentation using dynamic Bayesian networks. IEEE Transactions on Multimedia, 2007, vol. 9, no. 1, pp. 25–36. doi: 10.1109/TMM.2006.886337
59. Bilmes J.A., Bartels C. Graphical model architectures for speech recognition. IEEE Signal Processing Magazine, 2005, vol. 22, no. 5, pp. 89–100. doi: 10.1109/MSP.2005.1511827
60. Bengio S. Multimodal speech processing using asynchronous hidden Markov models. Information Fusion, 2004, vol. 5, no. 2, pp. 81–89. doi: 10.1016/j.inffus.2003.04.001
61. Morency L.-P., de Kok I., Gratch J. A probabilistic multimodal approach for predicting listener backchannels. Autonomous Agents and Multi-Agent Systems, 2010, vol. 20, no. 1, pp. 70–84. doi: 10.1007/s10458-009-9092-y
62. Casanovas A.L., Monaci G., Vandergheynst P., Gribonval R. Blind audiovisual source separation based on sparse redundant representations. IEEE Transactions on Multimedia, 2010, vol. 12, no. 5, pp. 358–371. doi: 10.1109/TMM.2010.2050650
63. Liu Q., Wang W., Jackson P.J.B., Barnard M., Kittler J., Chambers J. Source separation of convolutive and noisy mixtures using audio-visual dictionary learning and probabilistic time-frequency masking. IEEE Transactions on Signal Processing, 2013, vol. 61, no. 22, pp. 5520–5535. doi: 10.1109/TSP.2013.2277834