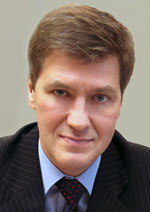
Nikiforov
Vladimir O.
D.Sc., Prof.
doi: 10.17586/2226-1494-2016-16-4-663-669
DISTANCE MEASUREMENT BASED ON A SINGLE DEFOCUSED PHOTOGRAPH
Read the full article

For citation: Degotinsky N.A., Lutsiv V.R. Distance measurement based on a single defocused photograph. Scientific and Technical Journal of Information Technologies, Mechanics and Optics, 2016, vol. 16, no. 4, pp. 663–669. doi: 10.17586/2226-1494-2016-16-4-663-669
Abstract
Subject of Research. Westudied a method of estimating the object distance on the basis of its single defocused photograph. The method is based on the analysis of image defocus at the contour points corresponding to borders of photographed objects. It is supposed that the brightness drop in not defocused image of border can be simulated with an ideal step function – the Heaviside function. Method. The contours corresponding to local maxima of brightness gradient are detected in the initial image to be analyzed and recorded for further analysis. Then the initial image is subjected to additional defocusing by a Gaussian filter having the dispersion parameter of defined in advance value. The ratios of local gradient values for the initial and additionally defocused images are then calculated at the contour points, and the defocus values of initial image at the points of objects borders are estimated based on these ratios. A sparse map of relative remoteness is built on the basis of these estimations for the border points of photographed objects, and a dense depth map of relative distances is then calculated using a special interpolation technique. Main Results. The efficiency of described technique is illustrated with the results of distance estimation in the photographs of real environment. Practical Relevance. In contrast to the widely applied stereo-technique and distance measurement methods analyzing the sets of defocused images, the investigated approach enables dealing with a single photograph acquired in a standard way without setting any additional conditions and limitations. If the relative remoteness of objects may be estimated instead of their absolute distances, no special calibration is needed for the camera applied, and the pictures taken once previously in diversified situations can be analyzed using the considered technique.
References
Chilian A., Hirschmüller H. Stereo camera based navigation of mobile robots on rough terrain. IEEE Int. Conf. on Intelligent Robots and Systems, IROS. St. Louis, USA, 2009, pp. 4571–4576. doi: 10.1109/IROS.2009.5354535
2. Mrovlje J., Vrancic D. Distance measuring based on stereoscopic pictures. Proc. 9th International PhD Workshop on Systems and Control. Izola, Slovenia, 2008.
3. Chaudhuri S., Rajagopalan A.N. Depth from Defocus: a Real Aperture Imaging Approach. Springer, 1999, 172 p. doi: 10.1007/978-1-4612-1490-8
4. Szeliski R. Computer Vision: Algorithms and Applications. Washington, Springer, 2011, 812 p. doi: 10.1007/978-1-84882-935-0
5. Horn B.K.P. Obtaining shape from shading information. In The Psychology of Computer Vision (P.H. Winston, Ed.). McGraw-Hill, 1975.
6. Marr D. Vision. A Computational Investigation into the Human Representation and Processing of Visual Information. NY, W.H. Freeman and Company, 1982, 415 p.
7. Pentland A. A new sense for depth of field. IEEE Transactions on Pattern Analysis and Machine Intelligence, 1987, vol. PAMI-9, no. 4, pp. 523–531. doi: 10.1109/TPAMI.1987.4767940
8. Grossmann P. Depth from focus. Pattern Recognition Letters, 1987, vol. 5, no. 1, pp. 63–69. doi: 10.1016/0167-8655(87)90026-2
9. Volkova M.A., Lutsiv V.R. Application of the longitudinal chromatic aberration effect for distances measurement on the basis of a single photo. Scientific and Technical Journal of Information Technologies, Mechanics and Optics, 2016, vol. 16, no. 2, pp. 251–257. doi:10.17586/2226-1494-2016-16-2-251-257
10. Zhuo S., Sim T. On the recovery of depth from a single defocused image. Lecture Notes in Computer Science, 2009, vol. 5702, pp. 889–897. doi: 10.1007/978-3-642-03767-2_108
11. Ray S.F. Applied Photographic Optics: Lenses and Optical Systems for Photography, Film, Video, and Electronic Imaging. Oxford, Focal Press, 2002, 89 p.
12. Gonsales R.C., Woods R.E., Eddins S.L. Digital Image Processing Using MATLAB. Prentice Hall, 2004, 344 p.
13. Canny J. A computational approach to edge detection. IEEE Transactions on Pattern Analysis and Machine Intelligence, 1986, vol. PAMI-8, no. 6, pp. 679–698. doi: 10.1109/TPAMI.1986.4767851
14. Damelin S., Miller W. The Mathematics of Signal Processing. Cambridge, Cambridge University Press, 2012, 462 p.
15. He K., Sun J., Tang X. Fast matting using large kernel matting Laplacian matrices. Proc. IEEE Computer Society Conference on Computer Vision and Pattern Recognition, CVPR. San Francisco, 2010, pp. 2165–2172. doi: 10.1109/CVPR.2010.5539896
16. Van der Vorst H.A. Bi-CGSTAB: a fast and smoothly converging variant of Bi-CG for the solution of nonsymmetric linear systems. SIAM Journal on Scientific Computing, 1992, vol. 13, pp. 631–644.