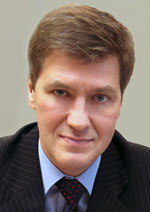
Nikiforov
Vladimir O.
D.Sc., Prof.
doi: 10.17586/2226-1494-2017-17-1-117-128
DYNAMIC FEATURE SELECTION FOR WEB USER IDENTIFICATION ON LINGUISTIC AND STYLISTIC FEATURES OF ONLINE TEXTS
Read the full article

For citation: Vorobeva A.A. Dynamic feature selection for web user identification on linguistic and stylistic features of online texts. Scientific and Technical Journal of Information Technologies, Mechanics and Optics, 2017, vol. 17, no. 1, pp. 117–128. doi: 10.17586/2226-1494-2017-17-1-117-128
Abstract
The paper deals with identification and authentication of web users participating in the Internet information processes (based on features of online texts).In digital forensics web user identification based on various linguistic features can be used to discover identity of individuals, criminals or terrorists using the Internet to commit cybercrimes. Internet could be used as a tool in different types of cybercrimes (fraud and identity theft, harassment and anonymous threats, terrorist or extremist statements, distribution of illegal content and information warfare). Linguistic identification of web users is a kind of biometric identification, it can be used to narrow down the suspects, identify a criminal and prosecute him. Feature set includes various linguistic and stylistic features extracted from online texts. We propose dynamic feature selection for each web user identification task. Selection is based on calculating Manhattan distance to k-nearest neighbors (Relief-f algorithm). This approach improves the identification accuracy and minimizes the number of features. Experiments were carried out on several datasets with different level of class imbalance. Experiment results showed that features relevance varies in different set of web users (probable authors of some text); features selection for each set of web users improves identification accuracy by 4% at the average that is approximately 1% higher than with the use of static set of features. The proposed approach is most effective for a small number of training samples (messages) per user
References
1. Lebedev I.S., Sukhoparov M.Y. Methodologies of Internet portals users' short messages texts authorship identification based on the methods of mathematical linguistics. In the World of Scientific Discoveries, 2014, no. 6.1, pp. 599–622. (In Russian).
2. Vorob'yeva A.A., Gvozdev A.V. Anonymous website user identification based on combined feature set (writing style and technical features). Scientific and Technical Journal of Information Technologies, Mechanics and Optics, 2014, no. 1, pp. 139–144. (In Russian).
3. Abbasi A., Chen H. Applying authorship analysis to extremist-group web forum messages. IEEE Intelligent Systems, 2005, vol. 20, no. 5, pp. 67–75. doi: 10.1109/MIS.2005.81
4. Frommholz I., al-Khateeb H.M., Potthast M., Ghasem Z., Shukla M., Short E. On textual analysis and machine learning for cyberstalking detection. Datenbank-Spektrum, 2016, vol. 16, no. 2, pp. 127–135. doi: 10.1007/s13222-016-0221-x
5. Rosenblum N., Zhu X., Miller B.P. Who wrote this code? Identifying the authors of program binaries. Lecture Notes in Computer Science, 2011, vol. 6879, pp. 172–189. doi: 10.1007/978-3-642-23822-2_10
6. Iqbal F., Binsalleeh H., Fung B.C.M., Debbabi M. A unified data mining solution for authorship analysis in anonymous textual communications. Information Sciences, 2013, vol. 231, pp. 98–112. doi: 10.1016/j.ins.2011.03.006
7. van der Knaap L., Grootjen F.A. Author identification in chatlogs using formal concept analysis. Proc. 19th Belgian-Dutch Conference on Artificial Intelligence, BNAIC, 2007, pp. 181–188.
8. Yule G.U. On sentence-length as a statistical characteristic of style in prose, with application to two cases of disputed authorship. Biometrika, 1939, vol. 30, no. 3/4, pp. 363–390. doi: 10.2307/2332655
9. Williams C.B. A note on the statistical analysis of sentence-length as a criterion of literary style. Biometrika, 1940, vol. 31, no. 3/4, pp. 356–361. doi: 10.2307/2332615
10. Mendenhall Т.С. A mechanical solution of a literary problem. Popular Science Monthly, 1901, vol. 60.
11. Greg W.W., Yule G.U. The statistical study of literary vocabulary. The Modern Language Review, 1944, vol. 39, no. 3, pp. 291. doi: 10.2307/3717870
12. Morozov N.A. Linguistic spectra: Means to distinguish plagiarism from the true works of one or the other well-known author: Stilemetrichesky sketch. Izvestiya Otdela Russkogo Yazyka i Slovesnosti Imperatorskoi Akademii Nauk, 1915, vol. 20, pp. 93–127. (In Russian)
13. Mosteller F., Wallace D. Inference and Disputed Authorship: The Federalist. Addison-Wesley, 1964,287 p.
14. Fomenko V.P., Fomenko T.G. Avtorskii invariant russkikh literaturnykh tekstov. In Fomenko A.T. Novaya Khronologiya Gretsii. Moscow, MSU Publ., 1995, vol. 2. (In Russian)
15. Potthast M., Braun S., Buz T., Duffhauss F., Friedrich F. et al. Who wrote the web? Revisiting influential author identification research applicable to information retrieval. Lecture Notes in Computer Science, 2016, vol. 9626, pp. 393–407. doi: 10.1007/978-3-319-30671-1_29
16. Haj Hassan F.I., Chaurasia M.A. N-gram based text author verification. Proc. Int. Conf. on Innovation and Information Management, ICIIM 2012. Chengdu, China, 2012, vol. 36, pp. 67–71.
17. Corney M., Anderson A., Mohay G., de Vel. O. Identifying the authors of suspect email. 2001. Available at: http://eprints.qut.edu.au/8021/1/CompSecurityPaper.pdf (accessed: 22.07.2016).
18. de Vel O., Anderson A., Corney M., Mohay G. Mining e-mail content for author identification forensics. ACM SIGMOD Record, 2001, vol. 30, no. 4, pp. 55–64. doi: 10.1145/604264.604272
19. Zheng R., Li J., Huang Z., Chen H. A Framework for authorship identification of online messages: writing style features and classification techniques. Journal of the American Society for Information Science and Technology, 2006, vol. 57, no. 3, pp. 378–393. doi: 10.1002/asi.20316
20. Luyckx K., Daelemans W. Personae, a corpus for author and personality prediction from text. Proc. LREC, 2008, vol. L08-1, pp. 2981–2987.
21. Romanov A.S. Technique and Software Package for the Identification of Author of an Unknown Text. PhD Thesis Eng. Sci. Tomsk, 2010, 26 p. (In Russian)
22. Sukhoparov M.E. Technique for Identification of Internet Portals Users Based on Mathematical Linguistics Methods. PhD Thesis Eng. Sci. St. Petersburg, 2015, 18 p. (In Russian)
23. Afroz S. Deception in Authorship Attribution. PhD thesis. Drexel University, 2013.
24. Yang M., Chow K.P. Authorship attribution for forensic investigation with thousands of authors. Proc. 29th IFIP Advances in Information and Communication Technology, 2014, vol. 428, pp. 339–350. doi: 10.1007/978-3-642-55415-5_28
25. Kuznetsov A.V. Written colloquial speach in online communication. Molodoi Uchenyi, 2011, no. 3-2, pp. 24–26. (In Russian).
26. Sigachev A.S. Model of text as a set of numerical signs. Intellektual'nye Tekhnologii i Sistemy, 2006, no. 7. (In Russian).
27. Vorobeva A.A. List of functional words used for web user (author) identification, 2016. doi: 10.13140/RG.2.2.30776.14080
28. Vorobeva A.A. Examining the performance of classification algorithms for imbalanced data sets in web author identification. Proc. 18th Conference of Open Innovations Association, 2016, pp. 385–390. doi: 10.1109/fruct-ispit.2016.7561554
29. Houvardas J., Stamatatos E. N-gram feature selection for authorship identification. Lecture Notes in Computer Science, 2006, vol. 4183, pp. 77–86. doi: 10.1007/11861461_10
30. Kononenko I. Estimating attributes: analysis and extensions of RELIEF. Lecture Notes in Computer Science, 1994, vol. 784, pp. 171–182. doi: 10.1007/3-540-57868-4_57
31. Vorobeva A.A. Forensic linguistics: automatic web author identification. Scientific and Technical Journal of Information Technologies, Mechanics and Optics, 2016, vol. 16, no. 2, pp. 295–302. doi:10.17586/2226-1494-2016-16-2-295-302
32. Vorob'eva A.A., Pantyukhin I.S., Shved D.V. Tool to Create Database of Users Messages of the Internet Portals. Certificate of State Registration of Computer Programs, no. 2013661841.
33. Vorob'eva A.A., Pantyukhin I.S., Shved D.V. Software Component of Linguistic Analysis and Text Processingfor Author Identification. Certificate of State Registration of Computer Programs, no.2014611567.
34. Vorobeva A.A. 100 most informative features. 2016. Available at: https://www.researchgate.net/publication/311510278_100_Most_informative_features (accessed: 08.12.2016). doi: 10.13140/RG.2.2.10289.58724
|