Menu
Publications
2025
2024
2023
2022
2021
2020
2019
2018
2017
2016
2015
2014
2013
2012
2011
2010
2009
2008
2007
2006
2005
2004
2003
2002
2001
Editor-in-Chief
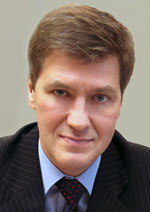
Nikiforov
Vladimir O.
D.Sc., Prof.
Partners
doi: 10.17586/2226-1494-2019-19-1-52-58
NEW IDENTIFICATION ALGORITHM FOR LINEARLY VARYING FREQUENCY OF SINUSOIDAL SIGNAL
Read the full article

Article in Russian
For citation:
Abstract
For citation:
Le Van Tuan, Korotina M.M., Bobtsov A.A., Aranovskiy S.V. New identification algorithm for linearly varying frequency of sinusoidal signal. Scientific and Technical Journal of Information Technologies, Mechanics and Optics , 2019, vol. 19, no. 1, pp. 52–58 (in Russian). doi: 10.17586/2226-1494-2019-19-1-52-58
Abstract
The paper deals with the problem of identification of linearly varying frequency of sinusoidal signal with unknown amplitude and phase. Identification task for linearly varying frequency occurs, for example, during telescope operation control and it is of practical interest. Existing synthesis methods for identification algorithms of linearly varying frequency of sinusoidal signal use unlimited functions of time that is not attractive from a technical point of view, since the measurement noise multiplied by an unlimited function tends to give extremely poor estimates of the tunable parameter. This paper proposes a new approach for identification of linearly varying frequency comprising iterative filtering of measured sinusoidal signal (with the use of linear first order stable components), which in turn gives the possibility to obtain a simple linear regression model with one unknown constant parameter. We present computer simulation results, illustrating the performance of the proposed identification algorithm. Computer modeling was performed both in the presence and absence of the measurement noise. Also, comparative analysis of the proposed approach with the previously obtained methods was carried out within the framework of computer simulation. It was shown that the presented solution provides a significant improvement in the accuracy of an unknown frequency identification in the noise presence.
Keywords: identification, sinusoidal signals, non-stationary frequency, linear regression model, robustness
Acknowledgements. This work is supported by the Russian Science Foundation, project No. 18-19-00627.
References
Acknowledgements. This work is supported by the Russian Science Foundation, project No. 18-19-00627.
References
1. Aranovskiy S.V., Bobtsov A.A., Pyrkin A.A. Identification of the linearly varying frequency for the sinusoidal signal. Scientific and Technical Journal of Information Technologies, Mechanics and Optics, 2012, no. 1, pp. 28–32. (in Russian)
2. Vasiliev V.N., Tomasov V.S., Shargorodsky V.D., Sadovnikov M.A. Precision electric drive systems used in high accuracy complexes of watching for space objects, current statement and development prospects. Izv. vuzov. Priborostroenie, 2008, vol. 51, no. 6, pp. 5–12. (in Russian)
3. Arakelyan A.K., Afanas'ev A.A. Valve Electrical Machines. Moscow, Energoatomizdat Publ., 1997, 236 p. (in Russian)
4. Erm T., Sandrock S. Adaptive correction of periodic errors improves telescope performance. Proc. American Control Conference. Portland, USA, 2005, vol. 6, pp. 3776–3777. doi: 10.1109/acc.20
5. Aranovskiy S., Bobtsov A., Kremlev A., Nikolaev N., Slita O. Identification of frequency of biased harmonic signal. European Journal of Control, 2010, vol. 16, no. 2, pp. 129–139. doi: 10.3166/ejc.16.129-139
6. Hou M. Parameter identification of sinusoids. IEEE Transactions on Automatic Control, 2012, vol. 57, no. 2, pp. 467–472. doi: 10.1109/TAC.2011.2164736
7. Marino R., Tomei P. Frequency estimation of periodic signals. Proc. European Control Conference. Strasbourg, France, 2014, pp. 7–12. doi: 10.1109/ecc.2014
8. Pyrkin A.A., Bobtsov A.A., Vedyakov A.A., Kolyubin S.A. Estimation of polyharmonic signal parameters. Automation and Remote Control, 2015, vol. 76, no. 8, pp. 1400–1416. doi: 10.1134/S0005117915080068
9. Bobtsov A.A., Efimov D., Pyrkin A.A., Zolghadri A. Switched algorithm for frequency estimation with noise rejection. IEEE Transactions on Automatic Control, 2012, vol. 57, no. 9, pp. 2400–2404. doi: 10.1109/TAC.2012.2186685
10. Aranovskiy S.V., Bobtsov A.A., Pyrkin A.A., Gritcenko P.A. Adaptive filters cascade applied to a frequency identification improvement problem. International Journal of Adaptive Control and Signal Processing, 2016, vol. 30, no. 5, pp. 677–689. doi: 10.1002/acs.2602
11. Aranovskiy S., Bobtsov A., Ortega R., Pyrkin A. Improved transients in multiple frequencies estimation via dynamic regressor extension and mixing. IFAC-Papers On Line, 2016, vol. 49, no. 13, pp. 99–104. doi: 10.1016/j.ifacol.2016.07.934
12. Le Van Tuan, Bobtsov A.A., Pyrkin A.A. New algorithm of variable parameters identification for linear regression model. Scientific and Technical Journal of Information Technologies, Mechanics and Optics, 2017, vol. 17, no. 5, pp. 952–955 (in Russian). doi: 10.17586/2226-1494-2017-17-5-952-955
13. Wang J., Le Van Tuan, Pyrkin A.A., Kolyubin S.A., Bobtsov A.A. Identification of piecewise linear parameters of regression models of non-stationary deterministic systems. Avtomatika i Telemekhanika, 2018, no. 12. (in Russian) (in press)
14. Ioannou P.A., Sun J. Robust Adaptive Control. Upper Saddle River, Prentice Hall, 1996, 825 p.
15. Miroshnik I.V., Nikiforov V.O., Fradkov A.L. Nonlinear and Adaptive Control of Complex Dynamic Systems. St. Petersburg, Nauka Publ., 2000, 549 p. (in Russian).
2. Vasiliev V.N., Tomasov V.S., Shargorodsky V.D., Sadovnikov M.A. Precision electric drive systems used in high accuracy complexes of watching for space objects, current statement and development prospects. Izv. vuzov. Priborostroenie, 2008, vol. 51, no. 6, pp. 5–12. (in Russian)
3. Arakelyan A.K., Afanas'ev A.A. Valve Electrical Machines. Moscow, Energoatomizdat Publ., 1997, 236 p. (in Russian)
4. Erm T., Sandrock S. Adaptive correction of periodic errors improves telescope performance. Proc. American Control Conference. Portland, USA, 2005, vol. 6, pp. 3776–3777. doi: 10.1109/acc.20
5. Aranovskiy S., Bobtsov A., Kremlev A., Nikolaev N., Slita O. Identification of frequency of biased harmonic signal. European Journal of Control, 2010, vol. 16, no. 2, pp. 129–139. doi: 10.3166/ejc.16.129-139
6. Hou M. Parameter identification of sinusoids. IEEE Transactions on Automatic Control, 2012, vol. 57, no. 2, pp. 467–472. doi: 10.1109/TAC.2011.2164736
7. Marino R., Tomei P. Frequency estimation of periodic signals. Proc. European Control Conference. Strasbourg, France, 2014, pp. 7–12. doi: 10.1109/ecc.2014
8. Pyrkin A.A., Bobtsov A.A., Vedyakov A.A., Kolyubin S.A. Estimation of polyharmonic signal parameters. Automation and Remote Control, 2015, vol. 76, no. 8, pp. 1400–1416. doi: 10.1134/S0005117915080068
9. Bobtsov A.A., Efimov D., Pyrkin A.A., Zolghadri A. Switched algorithm for frequency estimation with noise rejection. IEEE Transactions on Automatic Control, 2012, vol. 57, no. 9, pp. 2400–2404. doi: 10.1109/TAC.2012.2186685
10. Aranovskiy S.V., Bobtsov A.A., Pyrkin A.A., Gritcenko P.A. Adaptive filters cascade applied to a frequency identification improvement problem. International Journal of Adaptive Control and Signal Processing, 2016, vol. 30, no. 5, pp. 677–689. doi: 10.1002/acs.2602
11. Aranovskiy S., Bobtsov A., Ortega R., Pyrkin A. Improved transients in multiple frequencies estimation via dynamic regressor extension and mixing. IFAC-Papers On Line, 2016, vol. 49, no. 13, pp. 99–104. doi: 10.1016/j.ifacol.2016.07.934
12. Le Van Tuan, Bobtsov A.A., Pyrkin A.A. New algorithm of variable parameters identification for linear regression model. Scientific and Technical Journal of Information Technologies, Mechanics and Optics, 2017, vol. 17, no. 5, pp. 952–955 (in Russian). doi: 10.17586/2226-1494-2017-17-5-952-955
13. Wang J., Le Van Tuan, Pyrkin A.A., Kolyubin S.A., Bobtsov A.A. Identification of piecewise linear parameters of regression models of non-stationary deterministic systems. Avtomatika i Telemekhanika, 2018, no. 12. (in Russian) (in press)
14. Ioannou P.A., Sun J. Robust Adaptive Control. Upper Saddle River, Prentice Hall, 1996, 825 p.
15. Miroshnik I.V., Nikiforov V.O., Fradkov A.L. Nonlinear and Adaptive Control of Complex Dynamic Systems. St. Petersburg, Nauka Publ., 2000, 549 p. (in Russian).