Menu
Publications
2025
2024
2023
2022
2021
2020
2019
2018
2017
2016
2015
2014
2013
2012
2011
2010
2009
2008
2007
2006
2005
2004
2003
2002
2001
Editor-in-Chief
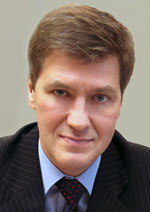
Nikiforov
Vladimir O.
D.Sc., Prof.
Partners
doi: 10.17586/2226-1494-2019-19-2-314-325
CONTROL METHODS FOR UPPER EXTREMITY PROSTHESES
Read the full article

Article in Russian
For citation:
Abstract
For citation:
Gorokhova N.M., Golovin M.A., ChezhinM.S. Control methods for upper extremity prostheses. Scientific and Technical Journal of Information Technologies, Mechanics and Optics, 2019, vol. 19, no. 2, pp. 314–325 (in Russian). doi: 10.17586/2226-1494-2019-19-2-314-325
Abstract
The paper deals with upper extremity prostheses control methods analysis. We consider prostheses control peculiarities for three main levels of amputation (prostheses for hand and fingers amputation, forearm amputation or forearm disarticulation, shoulder amputation or shoulder disarticulation). It is shown how amputation level is connected with used prosthesis control methods. The most perspective control methods for hand and fingers prostheses are the ones with extended feedback and adaptivity. It is beneficial to use neurointerfaces and muscle synergy phenomenon in control methods for forearm prostheses. Non-invasive and intuitive control methods are considered to be the most effective for shoulder prostheses. In spite of a great variety of modern technological achievements, the question of full recovery for all upper extremity functions remains open. A number of still unsolved problems, concerning the development of upper extremity prostheses control systems, is outlined: organization of extended “human – prosthesis”feedback, an effective control of multiple degrees of freedom, and simultaneous usage of the useful signal sources of different origins.
Keywords: upper extremity prosthesis, control system, control method, automatic control, EMG, EEG, pattern recognition, neural network, intuitive control, adaptive control
References
References
-
Kurdybailo S.F., Zamilatskii Yu.I., Andrievskaya A.O., Burov G.N.,Antipov A.V., Chekushina G.V., Petrov V.G. Constructions of Upper Limb Prostheses. Tutorial: Historical Review. St. Petersburg, Nimfa Publ., 2009, 458 p. (in Russian)
-
Kirakozov L.R., Monakhova M.I. Market research on highly functional electromechanical brushes for prosthetic upper limbs with bioelectric control. All-Russian Prosthetists and Orthopaedists Guild Bulletin, 2014, no. 4, pp. 23–26. (in Russian)
-
Burov G.N., Bolshakov V.A., Bolshakova M.A. Principles of creation of modern rehabilitation devices in prosthetics of the upper extremities. All-Russian Prosthetists and Orthopaedists Guild Bulletin, 2017, no. 1, pp. 9–13. (in Russian)
-
Bolshakov V.A., Burov G.N. On the formation of a prosthesis control system for amputation defects within the forearm.
All-Russian Prosthetists and Orthopaedists Guild Bulletin, 2014, no. 4, pp. 31–33. (in Russian) -
Burov G.N. Analysis of the control systems for upper limb prostheses. In Guide to Prosthetics and Orthotics. Eds. M.A. Dymochka, A.I. Sukhoverkhova, B.G. Spivak. 3rded. Vol. 2. Moscow, 2016, pp. 106–116. (in Russian)
-
Kuzhekin A.P., Moreinis I.Sh., Yakobson Ya.S. et al.Designs of Prosthetic and Orthopedic Products. Moscow, Legkaya i Pishchevaya Promyshlennost' Publ., 1984, 240 p. (in Russian)
-
Burov G.N., Bolshakov V.A. Determination of requirements to the sources of control signals of the forearm prosthesis control system. All-Russian Prosthetists and Orthopaedists Guild Bulletin, 2017, no. 2, pp. 36–40. (in Russian)
-
Burov G.N., Bolshakov V.A. Study of compensatory movements using a goniometric complex after forearm amputations. All-Russian Prosthetists and Orthopaedists Guild Bulletin, 2014, no. 2, pp. 15–19. (in Russian)
-
Pasluosta C.F., Chiu A.W.L. Modulation of grasping force in prosthetic hands using neural network-based predictive control. Methods in Molecular Biology, 2015, vol. 1260, pp. 179–194. doi: 10.1007/978-1-4939-2239-0_11
-
Rasouli M. et al. Stable force-myographic control of a prosthetic hand using incremental learning. Proc. 37th Annual Int. Conf. of the IEEE Engineering in Medicine and Biology Society. Milan, Italy,2015, pp. 4828–4831. doi: 10.1109/embc.2015.7319474
-
Fukushima S., Nozaki T., Ohnishi K. Development of haptic prosthetic hand for realization of intuitive operation. Proc. 42nd Annual Conference of the IEEE Industrial Electronics Society, 2016, pp. 6403–6408. doi: 10.1109/IECON.2016.7793456
-
Ortega-Palacios R. et al. Low-cost upper limb prosthesis, based on opensource projects with voice-myoelectric hybrid control. Proc. GMEPE/PAHCE. Porto, Portugal, 2018. doi: 10.1109/GMEPE-PAHCE.2018.8400727
-
Mablekos-Alexiou A. et al. A biomechatronic extended physiological proprioception (EPP) controller for upper-limb prostheses. Proc. IEEE/RSJ Int. Conf. on Intelligent Robots and Systems. Hamburg, Germany,2015, pp. 6173–6178. doi: 10.1109/IROS.2015.7354257
-
Dosen S. et al. EMG biofeedback for online predictive control of grasping force in a myoelectric prosthesis. Journal of Neuro Engineering and Rehabilitation, 2015, vol. 12, no. 1. doi: 10.1186/s12984-015-0047-z
-
Controzzi M. et al. The SSSA-MyHand: a dexterous lightweight myoelectric hand prosthesis. IEEE Transactions on Neural Systems and Rehabilitation Engineering, 2017, vol. 25, no. 5, pp. 459–468. doi: 10.1109/TNSRE.2016.2578980
-
Burov G.N., Bolshakov V.A. Bioelectrical Prosthesis Control System. Patent RU2653820, 2018.
-
Ortenzi V. et al. Ultrasound imaging for hand prosthesis control: a comparative study of features and classification methods. Proc. 2015 IEEE Int. Conf. on Rehabilitation Robotics. Singapore, 2015. doi: 10.1109/icorr.2015.7281166
-
Castellini C. et al. Using ultrasound images of the forearm to predict finger positions. IEEE Transactions on Neural Systems and Rehabilitation Engineering, 2012, vol. 20, no. 6, pp. 788–797.doi:10.1109/TNSRE.2012.2207916
-
Dalal N., Triggs B. Histograms of oriented gradients for human detection. Proc. IEEE Computer Society Conference on Computer Vision & Pattern Recognition, 2005, vol. 2, pp. 886–893.doi:10.1109/CVPR.2005.177
-
Li Q.M., Lv Y.P. A fuzzy PID control method for the grasping force of an underactuated prosthetic hand. Applied Mechanics and Materials, 2014, vol. 551, pp. 514–522. doi: 10.4028/www.scientific.net/AMM.551.514
-
Deepika Princess D. et al. MEMS accelerometer based control of prosthetic hand. International Research Journal of Medical Sciences, 2014, vol. 2, no. 4, pp. 20–23.
-
Pan L.et al. Myoelectric control based on a generic musculoskeletal model: toward a multi-user neural-machine interface. IEEE Transactions on Neural Systems and Rehabilitation Engineering, 2018, vol. 26, no. 7, pp. 1435–1442. doi: 10.1109/TNSRE.2018.2838448
-
Winters J.M. Hill-based muscle models: a systems engineering perspective. In Multiple Muscle Systems. Eds. J.M. Winters, S.LY. Woo. Springer, 1990. doi: 10.1007/978-1-4613-9030-5_5
-
Merad M. et al. Intuitive prosthetic control using upper limb inter-joint coordinations and IMU-based shoulder angles measurement: a pilot study. IEEE/RSJ Int. Conf. on Intelligent Robots and Systems, 2016, pp. 5677–5682. doi: 10.1109/IROS.2016.7759835
-
Ogiri Y. et al. Development of an upper limb neuroprosthesis to voluntarily control elbow and hand. Proc. 26th IEEE Int. Symposium on Robot and Human Interactive Communication, RO-MAN. Lisbon, Portugal, 2017, pp. 298–303. doi: 10.1109/ROMAN.2017.8172317
-
Ruhunage I. et al. EMG signal controlled transhumerai prosthetic with EEG-SSVEP based approch for hand open/close. Proc. IEEE Int. Conf. on Systems, Man, and Cybernetics. Banff, Canada, 2017, pp. 3169–3174. doi: 10.1109/SMC.2017.8123115
-
Bennett D.A., Goldfarb M. IMU-based wrist rotation control of a transradial myoelectric prosthesis. IEEE Transactions on Neural Systems and Rehabilitation Engineering, 2018, vol. 26, no. 2, pp. 419–427. doi: 10.1109/TNSRE.2017.2682642
-
Alshammary N.A. et al. Synergistic elbow control for a myoelectric transhumeral prosthesis. IEEE Transactions on Neural Systems and Rehabilitation Engineering, 2018, vol. 26, no. 2, pp. 468–476. doi: 10.1109/TNSRE.2017.2781719
-
Burov G.N., Bolshakov V.A. Forearm Prosthesis. Patent RU2615278, 2017.
-
Fajardo J. et al. An Affordable open-source multifunctional upper-limb prosthesis with intrinsic actuation. IEEE Workshop on Advanced Robotics and its Social Impacts. Austin, USA, 2017. doi: 10.1109/ARSO.2017.8025206
-
Lyons K.R., Sanjay S.J. Upper limb prosthesis control for high-level amputees via myoelectric recognition of leg gestures. IEEE Transactions on Neural Systems and Rehabilitation Engineering, 2018, vol. 26, no. 5, pp. 1056–1066. doi: 10.1109/TNSRE.2018.2807360
-
Nishino W. et al. Development of a myoelectric prosthesis simulator using augmented reality. IEEE International Conference on Systems, Man, and Cybernetics. Banff, Canada, 2017, pp. 1046–1051. doi: 10.1109/SMC.2017.8122749
-
Azhder V.D., Azhder T.B. Intelligentinformationsystems. Novaya Nauka: Sovremennoe Sostoyanie i Puti Razvitiya, 2017, no. 1-2, pp. 113–115. (in Russian)
-
Samant P., Agarwal R. Real-time speech recognition system for prosthetic arm control. International Journal of Sensing, Computing and Control, 2015, vol. 5, no. 1, pp. 39–46.
-
Golovin M.A., Burov G.N., Belyanin O.L. The structure and possibilities of the method of controlling the movements of the Tong and rotation of the hand in the upper limb prosthesis All-Russian Prosthetists and Orthopaedists Guild Bulletin, 2017, no. 1, pp. 14–18. (in Russian)
-
Burov G.N., Bolshakov V.A. Forearm Prosthesis. Patent RU2541816, 2015.
-
Amsuess S. et al. Self-correcting pattern recognition system of surface EMG signals for upper limb prosthesis control. IEEE Transactions on Biomedical Engineering, 2014, vol. 61, no. 4, pp. 1167–1176. doi: 10.1109/TBME.2013.2296274
-
Muhammed H.H., Raghavendra J. A new approach for rehabilitation and upper-limb prosthesis control using optomyography (OMG). Proc. 1st Int. Conf. on Biomedical Engineering. Yogyakarta, Indonesia, 2016. doi: 10.1109/IBIOMED.2016.7869814
-
Sherstan C. et al. A Collaborative approach to the simultaneous multi-joint control of a prosthetic arm. Proc. IEEE Int. Conf.
on Rehabilitation Robotics. Singapore, 2015. doi: 10.1109/ICORR.2015.7281168 -
Baker C.A. et al. Real-time, ultrasound-based control of a virtual hand by a trans-radial amputee. Proc. 38th Annual Int. Conf. of the IEEE Engineering in Medicine and Biology Society. Orlando, USA,2016, pp. 3219–3222. doi: 10.1109/EMBC.2016.7591414
-
Guo W. et al. Toward an enhanced human–machine interface for upper-limb prosthesis control with combined EMG and NIRS signals. IEEE Transactions on Human-Machine Systems, 2017, vol. 47, no. 4, pp. 564–575. doi: 10.1109/THMS.2016.2641389
-
Wilson S., Ravi V. Upper-limb prosthetic control using wearable multichannel mechanomyography. Proc. Int. Conf. on Rehabilitation Robotics. London, 2017, pp. 1293–1298. doi: 10.1109/ICORR.2017.8009427
-
Saravanan A. et al. A method to control bionic arm using Galvanic skin response. Proc. 8th Int. Conf. on Communication Systems and Networks. Bangalore, India, 2016. doi: 10.1109/COMSNETS.2016.7440030
-
Navaraj W.T. et al. Upper limb prosthetic control using toe gesture sensors. Proc. IEEE SENSORS. Busan, South Korea, 2015, pp. 1–4. doi: 10.1109/ICSENS.2015.7370369
-
Kim J.H. et al. Three-dimensional upper limb movement decoding from EEG signals. International Winter Workshop on Brain-Computer Interface. Gangwo, South Korea, 2013, pp. 109–111. doi:10.1109/IWW-BCI.2013.6506648
-
Cheesborough J. et al. Targeted muscle reinnervation and advanced prosthetic arms. Seminars in Plastic Surgery, 2015, vol. 29, no. 1, pp. 62–72. doi: 10.1055/s-0035-1544166
-
Tkach Dennis C. et al. Real-time and offline performance of pattern recognition myoelectric control using a generic electrode grid with targeted muscle reinnervation patients. IEEE Transactions on Neural Systems and Rehabilitation Engineering, 2014, vol. 22, no. 4, pp. 727–734. doi: 10.1109/TNSRE.2014.2302799
-
Lauretti C. et al. Fusion of M-IMU and EMG signals for the control of trans-humeral prostheses. Proc. 6th IEEE Int. Conf. on Biomedical Robotics and Biomechatronics. Singapore, 2016, pp. 1123–1128. doi: 10.1109/BIOROB.2016.7523782
-
He L., Pierre A.M. Degree-wise control of an humanoid manipulator based on biceps brachii muscle activity. IEEE EMBS International Student Conference. Ottawa, Canada, 2016, pp. 1–4. doi: 10.1109/EMBSISC.2016.7508612
-
Yang J. et al. Hybrid EEG-EOG system for intelligent prosthesis control based on common spatial pattern algorithm. IEEE Int. Conf. on Information and Automation. Ningbo, China,2016, pp. 1261–1266. doi: 10.1109/ICInfA.2016.7832013