Menu
Publications
2025
2024
2023
2022
2021
2020
2019
2018
2017
2016
2015
2014
2013
2012
2011
2010
2009
2008
2007
2006
2005
2004
2003
2002
2001
Editor-in-Chief
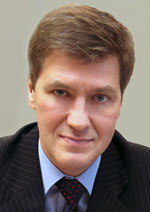
Nikiforov
Vladimir O.
D.Sc., Prof.
Partners
doi: 10.17586/2226-1494-2019-19-3-546-552
EFFECT OF VARIOUS DIMENSION CONVOLUTIONAL LAYER FILTERS ON TRAFFIC SIGN CLASSIFICATION ACCURACY
Read the full article

For citation:
Abstract
Sichkar V.N., Kolyubin S.A. Effect of various dimension convolutional layer filters on traffic sign classification accuracy. Scientific and Technical Journal of Information Technologies, Mechanics and Optics, 2019, vol. 19, no. 3, pp. 546–552 (in English). doi: 10.17586/2226-1494-2019-19-3-546-552
Abstract
The paper presents the study of an effective classification method for traffic signs on the basis of a convolutional neural network with various dimension filters. Every model of convolutional neural network has the same architecture but different dimension of filters for convolutional layer. The studied dimensions of the convolution layer filters are: 3 × 3, 5 × 5, 9 × 9, 13 × 13, 15 × 15, 19 × 19, 23 × 23, 25 × 25 and 31 ×31. In each experiment, the input image is convolved with the filters of certain dimension and with certain processing depth of image borders, which depends directly on the dimension of the filters and varies from 1 to 15 pixels. Performances of the proposed methods are evaluated with German Traffic Sign Benchmarks (GTSRB). Images from this dataset were reduced to 32 × 32 pixels in dimension. The whole dataset was divided into three subsets: training, validation and testing. The effect of the dimension of the convolutional layer filters on the extracted feature maps is analyzed in accordance with the classification accuracy and the average processing time. The testing dataset contains 12000 images that do not participate in convolutional neural network training. The experiment results have demonstrated that every model shows high testing accuracy of more than 82%. The models with filter dimensions of 9 × 9, 15 × 15 and 19 × 19 achieve top three with the best results on classification accuracy equal to 86.4 %, 86 % and 86.8 %, respectively. The models with filter dimensions of 5 × 5, 3 × 3 and 13 × 13 achieve top three with the best results on the average processing time equal to 0.001879, 0.002046 and 0.002364 seconds, respectively. The usage of convolutional layer filter with middle dimension has shown not only the high classification accuracy of more than 86 %, but also the fast classification rate, that enables these models to be used in real-time applications.
Keywords: traffic signs classification, convolutional neural network, convolutional layer filters, feature maps extraction, classification accuracy
References
References
1. Balali V., Ashouri Rad A., Golparvar-Fard M. Detection, classification, and mapping of U.S. traffic signs using google street view images for roadway inventory management. Visualization in Engineering, 2015, vol. 3, no. 1. doi: 10.1186/s40327-015-0027-1
2. Lu Y., Lu J., Zhang S., Hall P. Traffic signal detection and classification in street views using an attention model. Computational Visual Media, 2018, vol. 4, no. 3, pp. 253–266. doi: 10.1007/s41095-018-0116-x
3. Balali V., Golparvar-Fard M. Segmentation and recognition of roadway assets from car-mounted camera video streams using a scalable non-parametric image parsing method. Automation in Construction, 2016, vol. 49, pp. 27–39. doi: 10.1016/j.autcon.2014.09.007
4. Khalilikhah M., Heaslip K. The effects of damage on sign visibility: an assist in traffic sign replacement. Journal of Traffic and Transportation Engineering, 2016, vol. 3, no. 6, pp. 571– 581. doi: 10.1016/j.jtte.2016.03.009
5. Kryvinska N., Poniszewska-Maranda A., Gregus M. An approach towards service system building for road traffic signs detection and recognition. Procedia Computer Science, 2018, vol. 141, pp. 64–71. doi: 10.1016/j.procs.2018.10.150
6. Khalilikhah M., Heaslip K. Analysis of factors temporarily impacting traffic sign readability. International Journal of Transportation Science and Technology, 2016, vol. 5, no. 2, pp. 60–67. doi: 10.1016/j.ijtst.2016.09.003
7. Shustanov A., Yakimov P. CNN design for real-time traffic sign recognition. Procedia Engineering, 2017, vol. 201, pp. 718–725. doi: 10.1016/j.proeng.2017.09.594
8. Indolia S., Kumar Goswami A., Mishra S.P., Asopa P.. Conceptual understanding of convolutional neural network – a deep learning approach. Procedia Computer Science, 2018, vol. 132, pp. 679– 688. doi: 10.1016/j.procs.2018.05.069
9. Ozturk S., Akdemir B. Effects of histopathological image pre-processing on convolutional neural networks. Procedia Computer Science, 2018, vol. 132, pp. 396–403. doi: 10.1016/j.procs.2018.05.166
10. Kurniawan J., Syahra S.G.S., Dewa C.K., Afiahayati. Traffic congestion detection: learning from CCTV monitoring images using convolutional neural network. Procedia Computer Science, 2018, vol. 144, pp. 291–297. doi: 10.1016/j.procs.2018.10.530
11. Aghdam H.H., Heravi E.J., Puig D. A practical approach for detection and classification of traffic signs using Convolutional Neural Networks. Robotics and Autonomous Systems, 2016, vol. 84, pp. 97–112. doi: 10.1016/j.robot.2016.07.003
12. Stallkamp J., Schlipsing M., Salmen J., Igel C. The German traffic sign recognition benchmark: a multi-class classification competition. Proc. Int. Joint Conference on Neural Networks. San Jose, USA, 2011, pp. 1453–1460. doi: 10.1109/IJCNN.2011.6033395
13. Houben S., Stallkamp J., Salmen J., Schlipsing M., Igel C. Detection of traffic signs in real-world images: the german traffic sign detection benchmark. Proc. Int. Joint Conference on Neural Networks. Dallas, USA, 2013, pp. 1–8. doi: 10.1109/ IJCNN.2013.6706807
14. Eckle K., Schmidt-Hieber J. A comparison of deep networks with ReLU activation function and linear spline-type methods. Neural Networks, 2019, vol. 110, pp. 232–242. doi: 10.1016/j. neunet.2018.11.005
15. Lin G., Shen W. Research on convolutional neural network based on improved Relu piecewise activation function. Procedia Computer Science, 2018, vol. 131, pp. 977–984. doi: 10.1016/j. procs.2018.04.239
2. Lu Y., Lu J., Zhang S., Hall P. Traffic signal detection and classification in street views using an attention model. Computational Visual Media, 2018, vol. 4, no. 3, pp. 253–266. doi: 10.1007/s41095-018-0116-x
3. Balali V., Golparvar-Fard M. Segmentation and recognition of roadway assets from car-mounted camera video streams using a scalable non-parametric image parsing method. Automation in Construction, 2016, vol. 49, pp. 27–39. doi: 10.1016/j.autcon.2014.09.007
4. Khalilikhah M., Heaslip K. The effects of damage on sign visibility: an assist in traffic sign replacement. Journal of Traffic and Transportation Engineering, 2016, vol. 3, no. 6, pp. 571– 581. doi: 10.1016/j.jtte.2016.03.009
5. Kryvinska N., Poniszewska-Maranda A., Gregus M. An approach towards service system building for road traffic signs detection and recognition. Procedia Computer Science, 2018, vol. 141, pp. 64–71. doi: 10.1016/j.procs.2018.10.150
6. Khalilikhah M., Heaslip K. Analysis of factors temporarily impacting traffic sign readability. International Journal of Transportation Science and Technology, 2016, vol. 5, no. 2, pp. 60–67. doi: 10.1016/j.ijtst.2016.09.003
7. Shustanov A., Yakimov P. CNN design for real-time traffic sign recognition. Procedia Engineering, 2017, vol. 201, pp. 718–725. doi: 10.1016/j.proeng.2017.09.594
8. Indolia S., Kumar Goswami A., Mishra S.P., Asopa P.. Conceptual understanding of convolutional neural network – a deep learning approach. Procedia Computer Science, 2018, vol. 132, pp. 679– 688. doi: 10.1016/j.procs.2018.05.069
9. Ozturk S., Akdemir B. Effects of histopathological image pre-processing on convolutional neural networks. Procedia Computer Science, 2018, vol. 132, pp. 396–403. doi: 10.1016/j.procs.2018.05.166
10. Kurniawan J., Syahra S.G.S., Dewa C.K., Afiahayati. Traffic congestion detection: learning from CCTV monitoring images using convolutional neural network. Procedia Computer Science, 2018, vol. 144, pp. 291–297. doi: 10.1016/j.procs.2018.10.530
11. Aghdam H.H., Heravi E.J., Puig D. A practical approach for detection and classification of traffic signs using Convolutional Neural Networks. Robotics and Autonomous Systems, 2016, vol. 84, pp. 97–112. doi: 10.1016/j.robot.2016.07.003
12. Stallkamp J., Schlipsing M., Salmen J., Igel C. The German traffic sign recognition benchmark: a multi-class classification competition. Proc. Int. Joint Conference on Neural Networks. San Jose, USA, 2011, pp. 1453–1460. doi: 10.1109/IJCNN.2011.6033395
13. Houben S., Stallkamp J., Salmen J., Schlipsing M., Igel C. Detection of traffic signs in real-world images: the german traffic sign detection benchmark. Proc. Int. Joint Conference on Neural Networks. Dallas, USA, 2013, pp. 1–8. doi: 10.1109/ IJCNN.2013.6706807
14. Eckle K., Schmidt-Hieber J. A comparison of deep networks with ReLU activation function and linear spline-type methods. Neural Networks, 2019, vol. 110, pp. 232–242. doi: 10.1016/j. neunet.2018.11.005
15. Lin G., Shen W. Research on convolutional neural network based on improved Relu piecewise activation function. Procedia Computer Science, 2018, vol. 131, pp. 977–984. doi: 10.1016/j. procs.2018.04.239