Menu
Publications
2025
2024
2023
2022
2021
2020
2019
2018
2017
2016
2015
2014
2013
2012
2011
2010
2009
2008
2007
2006
2005
2004
2003
2002
2001
Editor-in-Chief
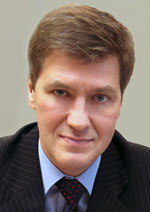
Nikiforov
Vladimir O.
D.Sc., Prof.
Partners
doi: 10.17586/2226-1494-2019-19-6-1072-1078
SEA SURFACE IMAGE SUB-BAND ANALYSIS BASED ON COSINE TRANSFORM
Read the full article

Article in Russian
For citation:
Abstract
For citation:
Chernomorets D.A., Mikhelev V.M., Bolgova E.V., Chernomorets A.A. Sea surface image sub-band analysis based on cosine transform. Scientific and Technical Journal of Information Technologies, Mechanics and Optics, 2019, vol. 19, no. 6, pp. 1072–1078 (in Russian). doi: 10.17586/2226-1494-2019-19-6-1072-1078
Abstract
Subject of Research. We propose an approach to the research of the frequency properties of the sea surface images sequence (video frames) on the basis of sub-band analysis within the cosine transform. The study of frequency properties makes it possible to solve the problem of object detection on the agitated sea surface. Method. The main theoretical propositions of the sub-band analysis within the cosine transform are given. The paper presents the method of formation of the cosine transform sub-band matrices used in data analysis and method of calculation of the analyzed vector energy parts in different frequency intervals. The sub-band approach to the analysis of the image component energy distribution in the frequency domain is described. Realization of separate components of the sea surface image sequence (video frames) in the vector form is shown. The vectors contain the pixel values with the same coordinates in the image set and give the possibility to calculate the energy parts in specified frequency intervals. Main Results. Video of the sea surface with various agitation levels were used in computing experiments. The distribution analysis of the energy part values of the matching components in the used video records showed that different sea surface agitation corresponds to different distributions of the matching component energy in the frequency domain. It has been found that at low agitation, the energy of the image components is concentrated in the low-frequency region and with the increase of the sea surface agitation, the energy part values of the corresponding image components increase in the high-frequency region. Practical Relevance. The obtained distribution features of the sea surface image component energy parts can be used when solving the detection problems for objects, floating on the sea surface, based on the analysis of their frequency characteristics.
Keywords: agitated sea surface, sub-band analysis, cosine transform, sub-band matrix, energy part, frequency interval
Acknowledgements. The reported study was funded by the RFBR, project number 19-07-00657.
References
Acknowledgements. The reported study was funded by the RFBR, project number 19-07-00657.
References
- Kravchenko V.F., Lutsenko V.I., Lutsenko I.V.. Scattering of radio waves by the sea and detection of objects against its background. Moscow, FIZMATLIT Publ., 2015, 448 p. (in Russian)
- Rodriguez-Blanco M., Golikov V. Multiframe GLRT-based adaptive detection of multipixel targets on a sea surface. IEEE Journal of Selected Topics in Applied Earth Observations and Remote Sensing, 2016, vol. 9, no. 12, pp. 5506–5512. doi: 10.1109/JSTARS.2016.2582383
- Golikov V., Rodriguez-Blanco M., Lebedeva O. Robust multipixel matched subspace detection with signal-dependent background power. Journal of Applied Remote Sensing, 2016, vol. 10, no. 1, pp. 015006. doi: 10.1117/1.JRS.10.015006
- Manolakis D., Truslow E., Pieper M., Cooley T., Brueggeman M. Detection algorithms in hyperspectral imaging systems: an overview of practical algorithms. IEEE Signal Processing Magazine, 2014, vol. 31, no. 1, pp. 24–33. doi: 10.1109/MSP.2013.2278915
- Scharf L.L., Friedlander B. Matched subspace detectors. IEEE Transactions on Signal Processing, 1994, vol. 42, no. 8, pp. 2146–2157. doi: 10.1109/78.301849
- Harsanyi J.C., Chang C.-I. Hyperspectral image classification and dimensionality reduction: an orthogonal subspace projection approach. IEEE Transactions on Geoscience and Remote Sensing, 1994, vol. 32, no. 4, pp. 779–785. doi: 10.1109/36.298007
- Kraut S., Scharf L.L., McWhorter L.T. Adaptive subspace detectors. IEEE Transactions on Signal Processing, 2001, vol. 49, no. 1, pp. 1–16. doi: 10.1109/78.890324
- Chen Y., Nasrabadi N.M., Tran T.D. Sparse representation for target detection in hyperspectral imagery. IEEE Journal on Selected Topics in Signal Processing, 2011, vol. 5, no. 3, pp. 629–640. doi: 10.1109/JSTSP.2011.2113170
- Manolakis D., Siracusa C., Shaw G. Hyperspectral subpixel target detection using the linear mixing model. IEEE Transactions on Geoscience and Remote Sensing, 2001, vol. 39, no. 7, pp. 1392–1409. doi: 10.1109/36.934072
- Zhang L., Zhang L., Tao D., Huang X., Du B. Hyperspectral remote sensing image subpixel target detection based on supervised metric learning. IEEE Transactions on Geoscience and Remote Sensing, 2014, vol. 52, no. 8, pp. 4955–4965. doi: 10.1109/TGRS.2013.2286195
- Golikov V., Lebedeva O., Rodriguez-Blanco M. GLRT subspace detection of multi-pixel targets with known and unknown spatial parameters in presence of signal-dependent background power. Computers and Electrical Engineering, 2016, vol. 52, pp. 38–48. doi: 10.1016/j.compeleceng.2016.04.016
- Zhilyakov E.G., Chernomorets A.A. Optimal separation of image subband components. Information Systems and Technologies, 2013, no. 1(75), pp. 5–11. (in Russian)
- Zhilyakov E.G., Belov S.P., Chernomorets A.A., Rakov V.I. Variational methods of analysis of signals based on the frequency of ideas. International Journal of Computer Applications in Technology, 2016, vol. 9, no. 35, pp. 71–76.
- Chernomorets A.A., Bolgova E.V. On the analysis of data based on the cosine transformation. Belgorod State University Scientific Bulletin. Economics. Information technologies, 2015, no. 1(198), pp. 68–73. (in Russian)
- Chernomorets A.A., Bolgova E.V., Chernomorets D.A. The generalized subband analysis on the basis of unitary transformations. Belgorod State University Scientific Bulletin. Economics. Information technologies, 2015, no. 7(204), pp. 97–104. (in Russian)
- Bolgova E.V. The properties of subinterval matrices for a two-dimensional cosine transform. Information Systems and Technologies, 2017, no. 6(104), pp. 1928. (in Russian)