Menu
Publications
2025
2024
2023
2022
2021
2020
2019
2018
2017
2016
2015
2014
2013
2012
2011
2010
2009
2008
2007
2006
2005
2004
2003
2002
2001
Editor-in-Chief
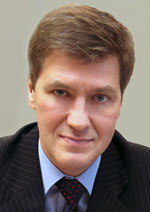
Nikiforov
Vladimir O.
D.Sc., Prof.
Partners
doi: 10.17586/2226-1494-2020-20-4-584-588
PARAMETRIC IDENTIFICATION OF DIFFERENTIAL-DIFFERENCE MODELS OF HEAT TRANSFER IN ONE-DIMENSIONAL BODIES BASED ON KALMAN FILTER ALGORITHMS
Read the full article

Article in Russian
For citation:
Abstract
For citation:
Pilipenko N.V., Zarichnyak Yu.P., Ivanov V.A., Khalyavin A.M. Parametric identification of differencial-difference models of heat transfer in one-dimensional bodies based on Kalman filter algorithms. Scientific and Technical Journal of Information Technologies, Mechanics and Optics, 2020, vol. 20, no. 4, pp. 584–588 (in Russian). doi: 10.17586/2226-1494-2020-20-4-584-588
Abstract
The paper considers the solution of the inverse heat conduction problem by parametric identification of differential- difference models of heat transfer in one-dimensional bodies. Differential-difference models is a system of differential ordinary equations of the first order with respect to the state vector. In this case, the direct and inverse heat conduction problems are solved, and the Kalman recurrent digital filter algorithm is used in terms of parameters for discrepancy minimization between the measured and model parameters values. The paper considers the Kalman algorithm application for two specific problems, namely: an experiment estimation and planning for restoration of heat transfer boundary conditions for a system of bodies. When planning an experiment or during field studies, the task parametrization is carried out initially and then parametric identification, as well. To determine the confidence area for measuring the desired parameters, the Gram matrix (Fisher information matrix) is used, involving the components which are sensitivity functions that represent all significant factors of heat metering: the type of heat transfer in the system, the number and location of temperature measurement points, the quality of channels for recording measured values, and the features of input actions, time measurement section and the number of measurement time points in this section. The paper gives an example of Kalman recurrent digital filter, considers the battery transformer of unsteady heat flux, for which the creation of the differential-difference model is carried out, shows the results of unsteady heat flux restoration, changing according to an arbitrary law. Confidence areas of the desired parameters are established.
Keywords: inverse heat conduction problem, parametric identification, Kalman filter
References
References
1. Beck J.V., Blackwell B., St. Clair Ch.R., jr. Inverse Heat Conduction. III-Posed Problems. James Beck, 1985, 308 p.
2. Kirillov K., Pilipenko N. Solution algorithms for direct and backward heat conductivity problems by means of differential-difference models. Scientific and Technical Bulletin of St. Petersburg State University of Information Technologies, Mechanics and Optics, 2010, no. 5(69), pp. 106–110. (in Russian)
3. Pilipenko N.V. The systematic errors in determining the nonstationary heat-exchange conditions with parametric identification. Measurement Techniques, 2007, vol. 50, no. 8, pp. 880–887. doi: 10.1007/s11018-007-0166-4
4. Pilipenko N.V., Polschikov G.V., Sivakov I.A. Setup for determination of dynamic characteristics of heat flux sensors. Journal of Instrument Engineering, 2013, vol. 56, no. 5, pp. 71–75. (in Russian)
5. Yaryshev N.A. Theoretical Basis of Non-Stationary Measurement of Temperature. Leningrad, Energoatomizdat Publ., 1990, 256 p. (in Russian)
6. Pilipenko N.V. Methods and Devices for Unsteady Heat Measuring Based on the Solution of Inverse Heat Conduction Problems. St. Petersburg, NRU ITMO Publ., 2011, 180 p. (in Russian)
7. Pilipenko N.V. Using the extended Kalman filter in nonstationary thermal measurement when solving inverse heat transfer problems. Journal of Instrument Engineering, 2019, vol. 62, no. 3, pp. 212–217. (in Russian). doi: 10.17586/0021-3454-2019-62-3-212-217
8. Pilipenko N.V. Methods of parametric identification in the non-stationary heat metering. Part 2. Journal of Instrument Engineering, 2003, vol. 46, no. 10, pp. 67–71. (in Russian)
9. Sivakov I.A., Pilipenko N.V. Kalman filter application when restoring heat flux density on study object surface in pulsed wind tunnel. Proc. IV All-Russian Scientific-Practical Conference with International Participation “Information Technologies in Science, Education and Economy”, 2012, pp. 55–58. (in Russian)
10. Pilipenko N.V. Uncertainty of non-stationary heat flux recovery by parametric identification of differential-difference model of heat transmission. Journal of Instrument Engineering, 2017, vol. 60, no. 7, pp. 664–671. (in Russian). doi: 10.17586/0021-3454-2017-60-7-664-671
11. Pilipenko N. Parametric identification of differential-difference heat transfer models in non-stationary thermal measurements. Heat Transfer Research, 2008, vol. 39, no. 4, pp. 311–315. doi: 10.1615/HeatTransRes.v39.i4.40
12. Pilipenko N.V., Kazartsev Y.V. Optimal design of experiment on heat exchange processes identification in heat flow sensors. Journal of Instrument Engineering, 2011, vol. 54, no. 5, pp. 88–93. (in Russian)
13. Hudson D.I. Statistic: Lectures on Elementary Statistics and Probability. 1964.
14. Pilipenko N.V. Retrieval of non-stationary heat flow based on solution of inverse problems of heat conduction. Journal of Instrument Engineering, 2017, vol. 60, no. 6, pp. 538–544. (in Russian). doi: 10.17586/0021-3454-2017-60-6-538-544
15. Pilipenko N.V. Non-stationary heat metering based on inverse thermal conduction problems. Proc. 9th International Scientific and Practical Conference “High-Tech Research, Development and Application in Industry”, 2010, pp. 388–392. (in Russian)