Menu
Publications
2025
2024
2023
2022
2021
2020
2019
2018
2017
2016
2015
2014
2013
2012
2011
2010
2009
2008
2007
2006
2005
2004
2003
2002
2001
Editor-in-Chief
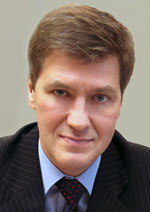
Nikiforov
Vladimir O.
D.Sc., Prof.
Partners
doi: 10.17586/2226-1494-2021-21-1-109-117
METHODS OF COUNTERING SPEECH SYNTHESIS ATTACKS ON VOICE BIOMETRIC SYSTEMS IN BANKING
Read the full article

Article in English
For citation:
Abstract
For citation:
Kuznetsov A.Yu., Murtazin R.A., Garipov I.M., Fedorov E.A., Kholodenina A.V., Vorobeva A.A. Methods of countering speech synthesis attacks on voice biometric systems in banking. Scientific and Technical Journal of Information Technologies, Mechanics and Optics, 2021, vol. 21, no. 1, pp. 109–117. doi: 10.17586/2226-1494-2021-21-1-109-117
Abstract
The paper considers methods of countering speech synthesis attacks on voice biometric systems in banking. Voice biometrics security is a large-scale problem significantly raised over the past few years. Automatic speaker verification systems (ASV) are vulnerable to various types of spoofing attacks: impersonation, replay attacks, voice conversion, and speech synthesis attacks. Speech synthesis attacks are the most dangerous as the technologies of speech synthesis are developing rapidly (GAN, Unit selection, RNN, etc.). Anti-spoofing approaches can be based on searching for phase and tone frequency anomalies appearing during speech synthesis and on a preliminary knowledge of the acoustic differences of specific speech synthesizers. ASV security remains an unsolved problem, because there is no universal solution that does not depend on the speech synthesis methods used by the attacker. In this paper, we provide the analysis of existing speech synthesis technologies and the most promising attacks detection methods for banking and financial organizations. Identification features should include emotional state and cepstral characteristics of voice. It is necessary to adjust the user’s voiceprint regularly. Analyzed signal should not be too smooth and containing unnatural noises or sharp interruptions changes in the signal level. Analysis of speech intelligibility and semantics are also important. Dynamic passwords database should contain words that are difficult to synthesize and pronounce. The proposed approach could be used for design and development of authentication systems for banking and financial organizations resistant to speech synthesis attacks.
Keywords: biometrics, automatic speaker verification, banking authentication, synthetic speech, spoofing detection
Acknowledgements. The paper was prepared at ITMO University within the framework of the scientific project No. 50449 “Development of cyberspace protection algorithms for solving applied problems of ensuring cybersecurity of banking organizations”.
References
Acknowledgements. The paper was prepared at ITMO University within the framework of the scientific project No. 50449 “Development of cyberspace protection algorithms for solving applied problems of ensuring cybersecurity of banking organizations”.
References
1. Fingerprint falsification — possible, but difficult. Available at: https:// www.kaspersky.ru/blog/sas2020-fingerprint-cloning/28101 (accessed: 20.12.2020)
2. Schemelinin V.L. Methods and complex of means to assess the efficiency of authentication by voice biometric systems. Dissertation for the degree of candidate of technical sciences, St. Petersburg, 2015. (in Russian)
3. Garipov I.M., Sulavko A.E., Kuprik I.A. Personality recognition methods based on analysis of the characteristics of the outer ear (review). Information security questions, 2020, no. 1(128), pp. 33–41. (in Russian)
4. Sudjenkova A.V. Overview of methods for extracting acoustic speech features in speaker recognition. Transaction of scientific papers of the Novosibirsk state technical university, 2019, no. 3-4(96), pp. 139–164. (in Russian).
5. Paul D., Pal M., Saha G. Spectral features for synthetic speech detection. IEEE Journal of Selected Topics in Signal Processing, 2017, vol. 11, no. 4, pp. 605–617. doi: 10.1109/JSTSP.2017.2684705
6. Huang T., Wang H., Chen Y., He P. GRU-SVM model for synthetic speech detection. Lecture Notes in Computer Science (including subseries Lecture Notes in Artificial Intelligence and Lecture Notes in Bioinformatics), 2020, vol. 12022, pp. 115–125. doi: 10.1007/978-3-030-43575-2_9
7. Yang J., Das R.K., Li H. Significance of subband features for synthetic speech detection. IEEE Transactions on Information Forensics and Security, 2020, vol. 15, pp. 2160–2170. doi: 10.1109/TIFS.2019.2956589
8. Sawada K. A statistical approach to speech synthesis and image recognition based on Hidden Markov Models. Doctoral dissertation, Nagoya Institute of Technology, 2018.
9. Saratxaga I., Sanchez J., Wu Z., Hernaez I., Navas E. Synthetic speech detection using phase information. Speech Communication, 2016, vol. 81, pp. 30–41. doi: 10.1016/j.specom.2016.04.001
10. van Niekerk B., Nortje L., Kamper H. Vector-quantized neural networks for acoustic unit discovery in the ZeroSpeech 2020 challenge. Proc. 21st Annual Conference of the International Speech Communication Association, INTERSPEECH, 2020, pp. 4836–4840. doi: 10.21437/Interspeech.2020-1693
11. Wu Z., Yamagishi J., Kinnunen T., Hanilçi C., Sahidullah M., Sizov A., Evans N., Todisco M., Delgado H. ASVspoof: the automatic speaker verification spoofing and countermeasures challenge. IEEE Journal of Selected Topics in Signal Processing, 2017, vol. 11, no. 4, pp. 588–604. doi: 10.1109/JSTSP.2017.2671435
12. Lavrentyeva G.M., Novoselov S.A., Kozlov A.V., Kudashev O.Yu., Shchemelinin V.L., Matveev Yu.N., De Marsico M. Audio-replay attacks spoofing detection for speaker recognition systems. Scientific and Technical Journal of Information Technologies, Mechanics and Optics, 2018, vol. 18, no. 4, pp. 428–437. (in Russian). doi: 10.17586/2226-1494-2018-18-3-428-436
13. Hunt A.J., Black A.W. Unit selection in a concatenative speech synthesis system using a large speech database. Proc. of the 1996 IEEE International Conference on Acoustics, Speech, and Signal Processing, ICASSP, 1996, pp. 373–376. doi: 10.1109/ICASSP.1996.541110
14. Jiang Y., Zhou X.C., Hu Ding Y.J., Ling Z.H., Dai L.R. The USTC system for Blizzard Challenge 2018. Blizzard Challenge Workshop, 2018.
15. Kaliev A., Rybin S.V. Speech synthesis: past and present. Computer Tools in Education, 2019, no. 1, pp. 5–28. (in Russian). doi: 10.32603/2071-2340-2019-1-5-28
16. Schemelinin V.L., Simonchik K.K. Study of voice verification system tolerance to spoofing attacks using a text-to-speech system. Journal of Instrument Engineering, 2014, vol. 57, no. 2, pp. 84–88. (in Russian)
17. Sushchenok O.A. Estimation of an overall performance of biometric systems. Information Processing Systems, 2011, no. 4, pp. 79–81. (in Russian)
18. Wu Z., Gao S., Cling E.S., Li H. A study on replay attack and anti- spoofing for text-dependent speaker verification. Proc. of the Asia- Pacific Signal and Information Processing Association Annual Summit and Conference, APSIPA 2014, 2014, pp. 7041636. doi: 10.1109/APSIPA.2014.7041636
19. Villalba J., Lleida E. Preventing replay attacks on speaker verification systems. Proc. of the IEEE International Carnahan Conference on Security Technology, ICCST, 2011, pp. 06095943. doi: 10.1109/CCST.2011.6095943
20. Pal M., Paul D., Saha G. Synthetic speech detection using fundamental frequency variation and spectral features. Computer Speech & Language, 2018, vol. 48, pp. 31–50. doi: 10.1016/j.csl.2017.10.001
21. Alam M.J., Kenny P., Bhattacharya G., Stafylakis T. Development of CRIM system for the automatic speaker verification spoofing and countermeasures challenge 2015. Proc. 16th Annual Conference of the International Speech Communication Association, INTERSPEECH’15, 2015, pp. 2072–2076.
22. Xiao X., Tian X., Du S., Xu H., Chng E.S., Li H. Spoofing speech detection using high dimensional magnitude and phase features: The NTU approach for ASVspoof 2015 challenge. Proc. 16th Annual Conference of the International Speech Communication Association, INTERSPEECH’15, 2015, pp. 2052–2056.
23. Patel T.B., Patil H.A. Combining evidences from mel cepstral, cochlear filter cepstral and instantaneous frequency features for detection of natural vs. spoofed speech. Proc. 16th Annual Conference of the International Speech Communication Association, INTERSPEECH’15, 2015, pp. 2062–2066.
24. Correia M.J., Abad A., Trancoso I. Preventing converted speech spoofing attacks in speaker verification. Proc. 37th International Convention on Information and Communication Technology, Electronics and Microelectronics, MIPRO, 2014, pp. 1320–1325. doi: 10.1109/MIPRO.2014.6859772
25. Nayana P.K., Mathew D., Thomas A. Performance comparison of speaker recognition systems using GMM and i-vector methods with PNCC and RASTA PLP features. Proc. of the International Conference on Intelligent Computing, Instrumentation and Control Technologies, ICICICT 2017, 2017, pp. 438–443. doi: 10.1109/ICICICT1.2017.8342603