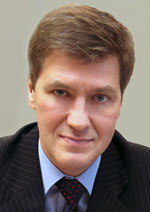
Nikiforov
Vladimir O.
D.Sc., Prof.
doi: 10.17586/2226-1494-2021-21-2-241-248
An analysis of the ways to reduce the vulnerability of networks based on the sequential removal of key elements
Read the full article

For citation:
Semenov K.V., Shuvaev F.L., Vitenzon K.I. An analysis of the ways to reduce the vulnerability of networks based on the sequential removal of key elements. Scientific and Technical Journal of Information Technologies, Mechanics and Optics, 2021, vol. 21, no. 2, pp. 241–248 (in Russian). doi: 10.17586/2226-1494-2021-21-2-241-248
Abstract
The research focuses on the metrics that allow assessing the stability of a graph and centrality measures. Their calculation underlies the percolation of the key elements of the graph. The experiment involved methods for calculating the average path length of vertex connectivity, clustering coefficient, and graph efficiency based on graph theory. To determine the optimal network stability metric, the principal component method was used, based on the theoretical provisions of mathematical statistics. In this study, the authors solved two scientific tasks: the main and auxiliary ones. The latter was to review the existing network stability metrics, which allowed them to choose the optimal one. The choice of the metrics was carried out using the principal component method. As a result, the average path length proved to be optimal. The solution of the auxiliary problem enabled the authors to analyze the ways to reduce the network stability based on the sequential removal of key elements, which is the main scientific task of the study. The analysis revealed that the nodes whose importance is expressed based on the measurement of centrality by degree are best suited for reducing the network stability. To estimate the stability of networks, an original complex two-criterion coefficient was developed. The analysis of the ways to reduce the stability was carried out by measuring this coefficient in model and real networks. Thus, testing of the proposed methods confirmed their efficiency and enabled their application in various fields of science and technology, e.g., sociology, medicine, physics and radio engineering.
References
- Ashtiani M., Salehzadeh-Yazdi A., Razaghi-Moghadam Z., Hennig H., Wolkenhauer O., Mirzaie M., Jafari M. A Systematic survey of centrality measures for protein-protein interaction networks. BMC Systems Biology, 2018, vol. 12, no. 1, pp. 80. doi: 10.1186/s12918-018-0598-2
- Eremeev I., Tatarka M., Shuvaev F., Cyganov A. Comparative analysis of centrality measures of network nodes based on principal component analysis. Informatics and Automation, 2020, vol. 19, no. 6, pp. 1307–1331. (in Russian). doi: 10.15622/ia.2020.19.6.7
- Singer Y. Dynamic measure of network robustness. Proc. IEEE 24th Convention of Electrical & Electronics Engineers in Israel, 2006, pp. 366–370. doi: 10.1109/EEEI.2006.321105
- Mingxing Z., Jing L., Shuai W., Shan H. A comparative study of robustness measures for cancer signaling networks. Big Data and Information Analytics, 2017, vol. 2, no. 1, pp. 87–96. doi: 10.3934/bdia.2017011
- Liu J., Zhou M., Wang S., Liu P. A comparative study of network robustness measures. Frontiers of Computer Science, 2017, vol. 11, no. 4, pp. 568–584. doi: 10.1007/s11704-016-6108-z
- Vitenzon K.I., Vishinskiy A.P., Kolesnik A.V. The method of estimating the structural stability of the satellite communication system taking into account the importance of transmitted information. Proceedings of the Military Space academy named after A.F. Mozhaisky, 2019, no. 667, pp. 74–79. (in Russian)
- Barabási A.-L. Network Science. Glasgow, Cambridge University Press, 2016, 453 p.
- Shuvaev F.L., Tatarka M.V. Analysis of mathematical models of random graphs used in simulation of information and communication networks. Vestnik Sankt-Peterburgskogo universiteta GPS MChS Rossii, 2020, no. 2, pp. 67–77. (in Russian)
- Newman M.E.J. Networks: An Introduction. N.Y., Oxford University Press Inc., 2010, 1042 p.
- Hartmann A., Mézard M. Distribution of diameters for Erdős-Rényi random graphs. Physical Review E, 2018, vol. 97, no. 3, pp. 032128. doi: 10.1103/PhysRevE.97.032128
- Csardi G., Nepusz T. The igraph software package for complex network research. InterJournal Complex Systems, 2006, vol. 1695.
- Lê S., Josse J., Husson F. FactoMineR: An R package for multivariate analysis. Journal of Statistical Software, 2008, vol. 25, no. 1, pp. 1–18. doi: 10.18637/jss.v025.i01
- Bonchi F., De Francisci Morales G., Riondato M. Centrality measures on big graphs: Exact, approximated, and distributed algorithms. Proc. of the 25th International Conference Companion on World Wide Web, 2016, pp. 1017–1020. doi: 10.1145/2872518.2891063
- Brandes U., Borgatti S., Freeman L. Maintaining the duality of closeness and betweenness centrality. Social Networks, 2016, vol. 44, pp. 153–159. doi: 10.1016/j.socnet.2015.08.003
- Shevchenko D.N., Litvin A.U., Fedianin M.A. Reliability analysis of technical systems by simulation modeling of graph of states. Problems of Physics, Mathematics and Technics, 2018, no. 3(36), pp. 101–104 (in Russian).
- Chen P.-Y., Choudhury S., Hero A.O. Multi-centrality graph spectral decompositions and their application to cyber intrusion detection. Proc. 41st IEEE International Conference on Acoustics, Speech and Signal Processing (ICASSP), 2016, pp. 4553–4557. doi: 10.1109/ICASSP.2016.7472539
- Shuvaev F.L., Tatarka M.V. Dynamics of centrality measures of random graph mathematical models. Scientific and Technical Journal of Information Technologies, Mechanics and Optics, 2020, vol. 20, no. 2, pp. 249–256. (in Russian). doi: 10.17586/2226-1494-2020-20-2-249-256.
- Gibson H., Vickers P. Using adjacency matrices to lay out larger small-world networks. Applied Soft Computing Journal, 2016, vol. 42, pp. 80–92. doi: 10.1016/j.asoc.2016.01.036
- Faust K. Comparing social networks: Size, density, and local structure. Metodološki zvezki, 2006, vol. 3, no. 2, pp. 185–216.
- HolmeP., Kim B.J., Yoon C.N., Han S.K. Attack vulnerability of complex networks. Physical Review E, 2002, vol. 65, no. 5, pp. 056109. doi: 10.1103/PhysRevE.65.056109
- Lu Z.-M., Li X.-F. Attack vulnerability of network controllability. PLoS One, 2016, vol. 11, no. 9, pp. e0162289. doi: 10.1371/journal.pone.0162289
- Dong S., Mostafizi A., Wang H., Gao J., Li X. Measuring the topological robustness of transportation networks to disaster-induced failures: a percolation approach. Journal of Infrastructure Systems, 2020, vol. 26, no. 2, pp. 04020009. doi: 10.1061/(ASCE)IS.1943-555X.0000533