Menu
Publications
2025
2024
2023
2022
2021
2020
2019
2018
2017
2016
2015
2014
2013
2012
2011
2010
2009
2008
2007
2006
2005
2004
2003
2002
2001
Editor-in-Chief
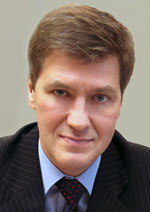
Nikiforov
Vladimir O.
D.Sc., Prof.
Partners
doi: 10.17586/2226-1494-2021-21-4-449-456
DREM procedure application for piecewise constant parameters identification
Read the full article

Article in Russian
For citation:
Abstract
For citation:
Glushchenko A.I., Petrov V.A., Lastochkin K.A. DREM procedure application for piecewise constant parameters identification. Scientific and Technical Journal of Information Technologies, Mechanics and Optics, 2021, vol. 21, no. 4, pp. 449–456 (in Russian). doi: 10.17586/2226-1494-2021-21-4-449-456
Abstract
The research focuses on the applicability of the Dynamic Regressor Extension and Mixing (DREM) procedure for the identification of the piecewise constant parameters of a linear regression. Unlike the known papers, it is shown that the application of the baseline DREM procedure to the identification of piecewise constant parameters generates the scalar perturbed regressions at some time intervals, which significantly deteriorates the quality of the unknown parameters estimates. The methods of the research imply integral and differential calculus and mathematical modeling. To solve the revealed problem, the authors propose a new method of dynamic regressor extension, which is based on the interval integral filtering with exponential forgetting and resetting. The study describes the modified DREM procedure, which, unlike the baseline one, allows one to generate the scalar regressions with an adjustable level of perturbation. Numerical experiments to identify piecewise constant parameters yielded the following results: the correctness of the obtained perturbed regression description and the presence of overshoot of such perturbed regression parameter estimates using the gradient method and FCT-D (Finite Convergence Time DREM). It is also shown that the values of such overshoot can be adjusted if the proposed modified DREM procedure is applied. The proposed procedure can be applied to the development of identification and adaptive control systems.
Keywords: identification, linear regression, DREM, FCT-D, interval-based filtration, finite excitation
Acknowledgements. The study was financially supported by the Russian Foundation for Basic Research (Project No. 18-47-310003-p_a)
References
Acknowledgements. The study was financially supported by the Russian Foundation for Basic Research (Project No. 18-47-310003-p_a)
References
-
Aranovskiy S., Bobtsov A., Ortega R., Pyrkin A. Performance enhancement of parameter estimators via dynamic regressor extension and mixing. IEEE Transaction on Automatic Control, 2017, vol. 62, no. 7, pp. 3546–3550. https://doi.org/10.1109/TAC.2016.2614889
-
Korotina M., Aranovskiy S., Ushirobira R., Vedyakov A.On parameter tuning and convergence properties of the DREM procedure. Proc. 18th European Control Conference, 2020, pp. 53–58. https://doi.org/10.23919/ECC51009.2020.9143808
-
Aranovskiy S., Belov A., Ortega R., Barabanov N., Bobtsov A. Parameter identification of linear time‐invariant systems using dynamic regressor extension and mixing. International Journal of Adaptive Control and Signal Processing, 2019, vol. 33, no. 6, pp. 1016–1030. https://doi.org/10.1002/acs.3006
-
Ortega R., Bobtsov A., Pyrkin A., Aranovskiy A. A parameter estimation approach to state observation of nonlinear systems. Systems and Control Letters, 2015, vol. 85, pp. 84–94. https://doi.org/10.1016/j.sysconle.2015.09.008
-
Gerasimov D., Ortega R., Nikiforov V. Adaptive control of multivariable systems with reduced knowledge of high frequency gain: Application of dynamic regressor extension and mixing estimators. IFAC-PapersOnLine, 2018, vol. 51, no. 15, pp. 886–890. https://doi.org/10.1016/j.ifacol.2018.09.108
-
Pyrkin A., Bobtsov A., Ortega R., Vedyakov A., Aranovskiy S. Adaptive state observers using dynamic regressor extension and mixing. Systems and Control Letters, 2019, vol. 133, pp. 104519. https://doi.org/10.1016/j.sysconle.2019.104519
-
Ortega R., Bobtsov A., Nikolaev N., Schiffer J., Dochain D.Generalized parameter estimation-based observers: Application to power systems and chemical–biological reactors. Automatica, 2021, vol. 129, pp. 109635. https://doi.org/10.1016/j.automatica.2021.109635
-
Ortega R., Nikiforov V., Gerasimov D. On modified parameter estimators for identification and adaptive control. A unified framework and some new schemes. Annual Reviews in Control, 2020, vol. 50, pp. 278–293. https://doi.org/10.1016/j.arcontrol.2020.06.002
-
Ortega R., Aranovskiy S., Pyrkin A., Astolfi A., Bobtsov A.New results on parameter estimation via dynamic regressor extension and mixing: Continuous and discrete-time cases. IEEE Transactions on Automatic Control, 2021, vol. 66, no. 5, pp. 2265–2272. https://doi.org/10.1109/TAC.2020.3003651
-
Bobtsov A., Yi B., Ortega R., Astolfi A. Generation of new exciting regressors for consistent on-line estimation of unknown parameters. arXiv.org, 2021, arXiv:2104.02210, pp. 1–6.
-
Ortega R., Bobtsov A., Nikolaev N. Parameter identification with finite-convergence time alertness preservation. IEEE Control Systems Letters, 2021, vol. 6, pp. 205–210. https://doi.org/10.1109/LCSYS.2021.3057012
-
Glushchenko A., Petrov V., Lastochkin K. Regression filtration with resetting to provide exponential convergence of MRAC for plants with jump change of unknown parameters. arXiv.org, 2021, arXiv:2102.10359, pp. 1–12.
-
Lee H.I., Shin H.S., Tsourdos A. Concurrent learning adaptive control with directional forgetting. IEEE Transactions on Automatic Control, 2019, vol. 64, no. 12, pp. 5164–5170. https://doi.org/10.1109/TAC.2019.2911863
-
Quoc D., Bobtsov A. An adaptive state observer for linear time-varying systems with inaccurate parameters. Automation and Remote Control, 2020, vol. 81, no. 12, pp. 2220–2229. https://doi.org/10.1134/S0005117920120061
-
Pan Y., Aranovskiy S., Bobtsov A., Yu H. Efficient learning from adaptive control under sufficient excitation. International Journal of Robust and Nonlinear Control, 2019, vol. 29, no. 10, pp. 3111–3124. https://doi.org/10.1002/rnc.4541
-
Ioannou P., Sun J. Robust Adaptive Control. N.Y., Dover, 2013, 834 p.